Adaptive Constraint Satisfaction for Markov Decision Process Congestion Games: Application to Transportation Networks
arxiv(2022)
摘要
Under the Markov decision process (MDP) congestion game framework, we study the problem of enforcing population distribution constraints on a population of players with stochastic dynamics and coupled congestion costs. Existing research demonstrates that the constraints on the players' population distribution can be satisfied by enforcing tolls. However, computing the minimum toll value for constraint satisfaction requires accurate modeling of the player's congestion costs. Motivated by settings where an accurate congestion cost model is unavailable (e.g. transportation networks), we consider an MDP congestion game with unknown congestion costs. We assume that a constraint-enforcing authority can repeatedly enforce tolls on a population of players who converges to an $\epsilon$-optimal population distribution for any given toll. We then construct a myopic update algorithm to compute the minimum toll value while ensuring that the constraints are satisfied on average. We analyze how the players' sub-optimal responses to tolls impact the rates of convergence towards the minimum toll value and constraint satisfaction. Finally, we construct a congestion game model for Uber drivers in Manhattan, New York City (NYC) using data from the Taxi and Limousine Commission (TLC) to illustrate how to efficiently reduce congestion while minimizing the impact on driver earnings.
更多查看译文
关键词
Markov decision process,Incentive design,Congestion games,Online optimization,Transportation systems,Stochastic games
AI 理解论文
溯源树
样例
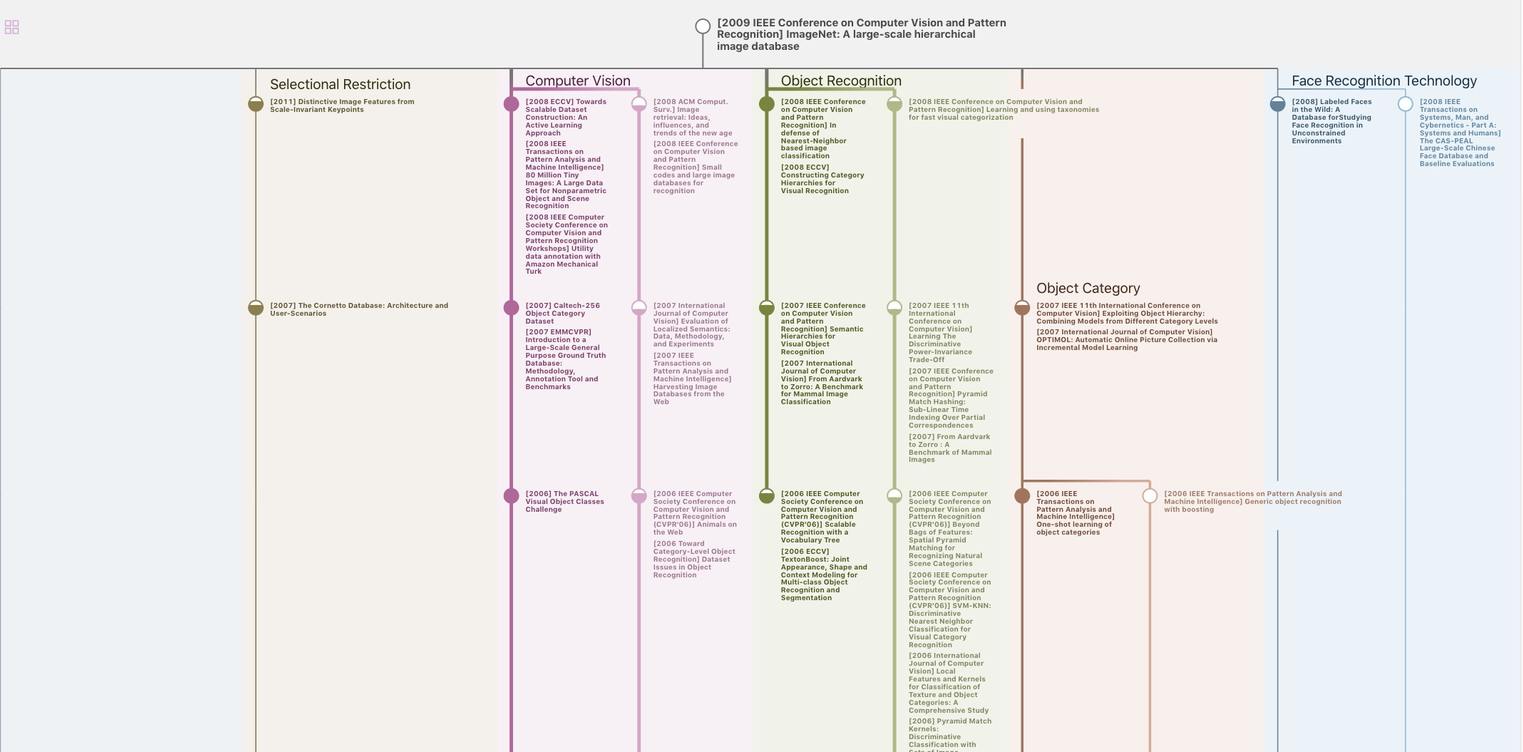
生成溯源树,研究论文发展脉络
Chat Paper
正在生成论文摘要