Off-policy Learning for Multiple Loggers
KDD'19: PROCEEDINGS OF THE 25TH ACM SIGKDD INTERNATIONAL CONFERENCCE ON KNOWLEDGE DISCOVERY AND DATA MINING(2019)
摘要
It is well known that the historical logs are used for evaluating and learning policies in interactive systems, e.g. recommendation, search, and online advertising. Since direct online policy learning usually harms user experiences, it is more crucial to apply off-policy learning in real-world applications instead. Though there have been some existing works, most are focusing on learning with one single historical policy. However, in practice, usually a number of parallel experiments, e.g. multiple AB tests, are performed simultaneously. To make full use of such historical data, learning policies from multiple loggers becomes necessary. Motivated by this, in this paper, we investigate off-policy learning when the training data coming from multiple historical policies. Specifically, policies, e.g. neural networks, can be learned directly from multi-logger data, with counterfactual estimators. In order to understand the generalization ability of such estimator better, we conduct generalization error analysis for the empirical risk minimization problem. We then introduce the generalization error bound as the new risk function, which can be reduced to a constrained optimization problem. Finally, we give the corresponding learning algorithm for the new constrained problem, where we can appeal to the minimax problems to control the constraints. Extensive experiments on benchmark datasets demonstrate that the proposed methods achieve better performances than the state-of-the-arts.
更多查看译文
关键词
log data, multiple loggers, off-policy learning
AI 理解论文
溯源树
样例
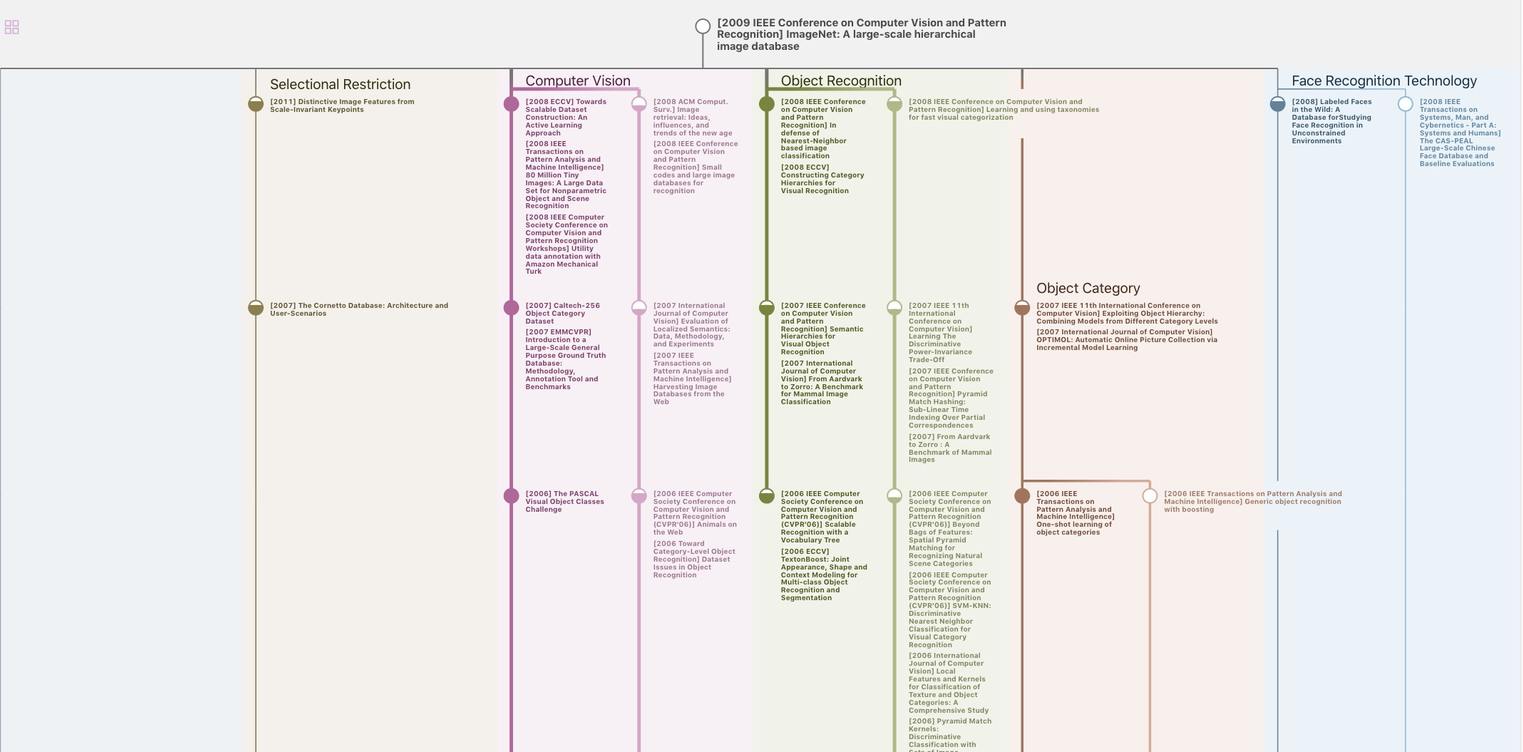
生成溯源树,研究论文发展脉络
Chat Paper
正在生成论文摘要