A deep learning system for predicting size and fit in fashion e-commerce
RECSYS 2019: 13TH ACM CONFERENCE ON RECOMMENDER SYSTEMS(2019)
摘要
Personalized size and fit recommendations bear crucial significance for any fashion e-commerce platform. Predicting the correct fit drives customer satisfaction and benefits the business by reducing costs incurred due to size-related returns. Traditional collaborative filtering algorithms seek to model customer preferences based on their previous orders. A typical challenge for such methods stems from extreme sparsity of customer-article orders. To alleviate this problem, we propose a deep learning based content-collaborative methodology for personalized size and fit recommendation. Our proposed method can ingest arbitrary customer and article data and can model multiple individuals or intents behind a single account. The method optimizes a global set of parameters to learn population-level abstractions of size and fit relevant information from observed customer-article interactions. It further employs customer and article specific embedding variables to learn their properties. Together with learned entity embeddings, the method maps additional customer and article attributes into a latent space to derive personalized recommendations. Application of our method to two publicly available datasets demonstrate an improvement over the state-of-the-art published results. On two proprietary datasets, one containing fit feedback from fashion experts and the other involving customer purchases, we further outperform comparable methodologies, including a recent Bayesian approach for size recommendation.
更多查看译文
关键词
cold-start problem, collaborative filtering, entity embedding, personalization, recommendation, size and fit prediction
AI 理解论文
溯源树
样例
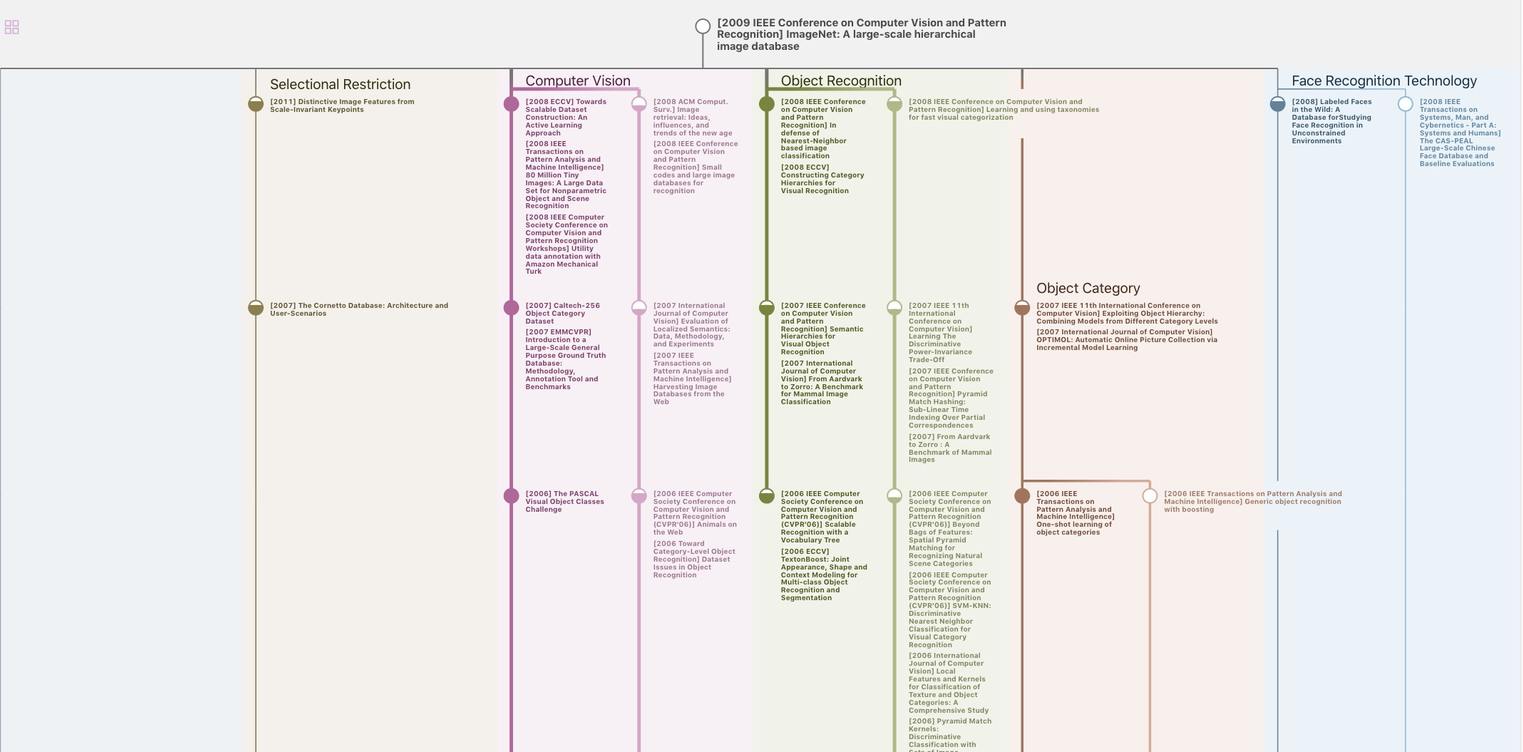
生成溯源树,研究论文发展脉络
Chat Paper
正在生成论文摘要