Temporal exponential random graph models of longitudinal brain networks after stroke
JOURNAL OF THE ROYAL SOCIETY INTERFACE(2022)
摘要
Plasticity after stroke is a complex phenomenon. Functional reorganization occurs not only in the perilesional tissue but throughout the brain. However, the local connection mechanisms generating such global network changes remain largely unknown. To address this question, time must be considered as a formal variable of the problem rather than a simple repeated observation. Here, we hypothesized that the presence of temporal connection motifs, such as the formation of temporal triangles (T) and edges (E) over time, would explain large-scale brain reorganization after stroke. To test our hypothesis, we adopted a statistical framework based on temporal exponential random graph models (tERGMs), where the aforementioned temporal motifs were implemented as parameters and adapted to capture global network changes after stroke. We first validated the performance on synthetic time-varying networks as compared to standard static approaches. Then, using real functional brain networks, we showed that estimates of tERGM parameters were sufficient to reproduce brain network changes from 2 weeks to 1 year after stroke. These temporal connection signatures, reflecting within-hemisphere segregation (T) and between hemisphere integration (E), were associated with patients' future behaviour. In particular, interhemispheric temporal edges significantly correlated with the chronic language and visual outcome in subcortical and cortical stroke, respectively. Our results indicate the importance of time-varying connection properties when modelling dynamic complex systems and provide fresh insights into modelling of brain network mechanisms after stroke.
更多查看译文
关键词
functional magnetic resonance imaging,functional connectivity,network models,stroke
AI 理解论文
溯源树
样例
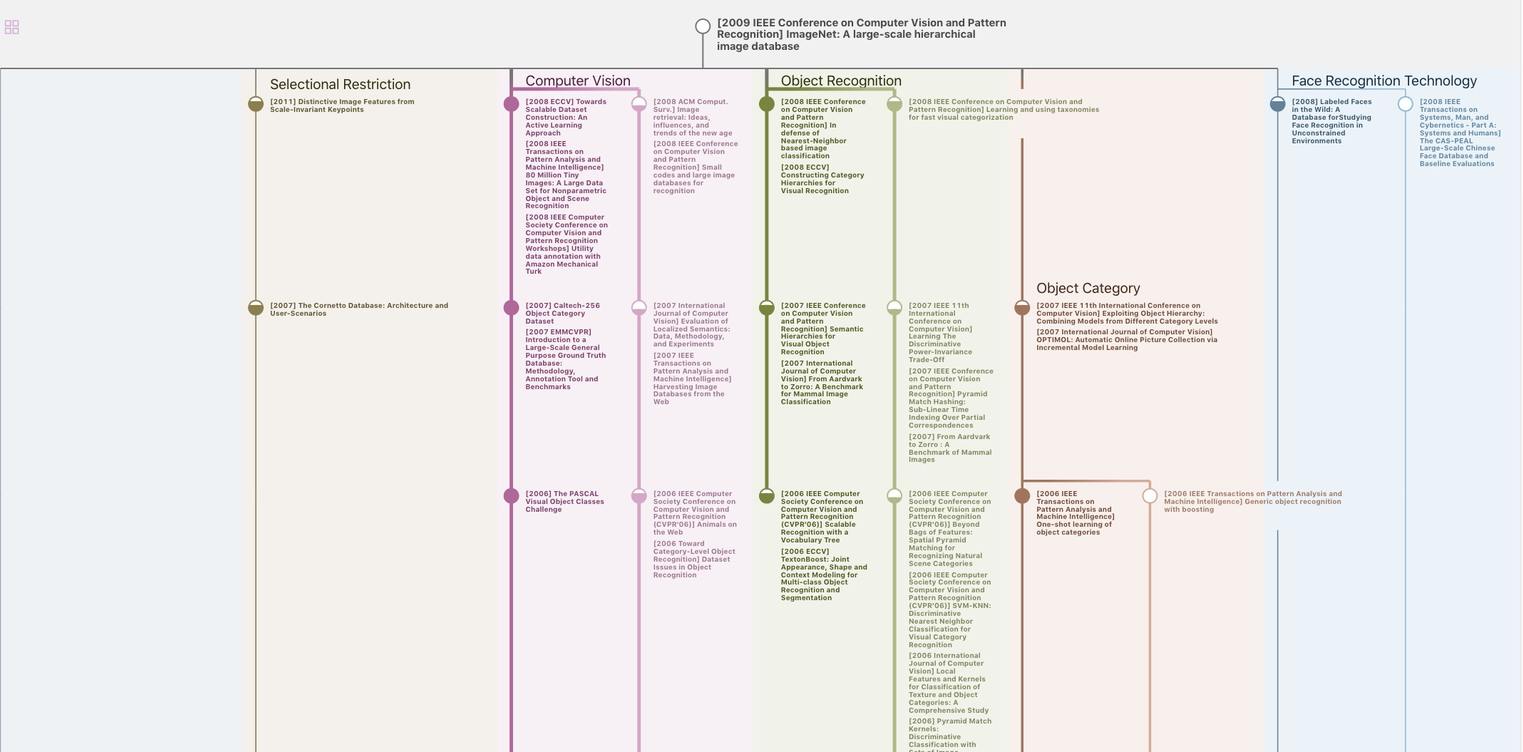
生成溯源树,研究论文发展脉络
Chat Paper
正在生成论文摘要