Leverage Implicit Feedback for Context-aware Product Search
eCOM@SIGIR(2019)
摘要
Product search serves as an important entry point for online shopping. In contrast to web search, the retrieved results in product search not only need to be relevant but also should satisfy customers' preferences in order to elicit purchases. Previous work has shown the efficacy of purchase history in personalized product search. However, customers with little or no purchase history do not benefit from personalized product search. Furthermore, preferences extracted from a customer's purchase history are usually long-term and may not always align with her short-term interests. Hence, in this paper, we leverage clicks within a query session, as implicit feedback, to represent users' hidden intents, which further act as the basis for re-ranking subsequent result pages for the query. It has been studied extensively to model user preference with implicit feedback in recommendation tasks. However, there has been little research on modeling users' short-term interest in product search. We study whether short-term context could help promote users' ideal item in the following result pages for a query. Furthermore, we propose an end-to-end context-aware embedding model which can capture long-term and short-term context dependencies. Our experimental results on the datasets collected from the search log of a commercial product search engine show that short-term context leads to much better performance compared with long-term and no context. Our results also show that our proposed model is more effective than word-based context-aware models.
更多查看译文
AI 理解论文
溯源树
样例
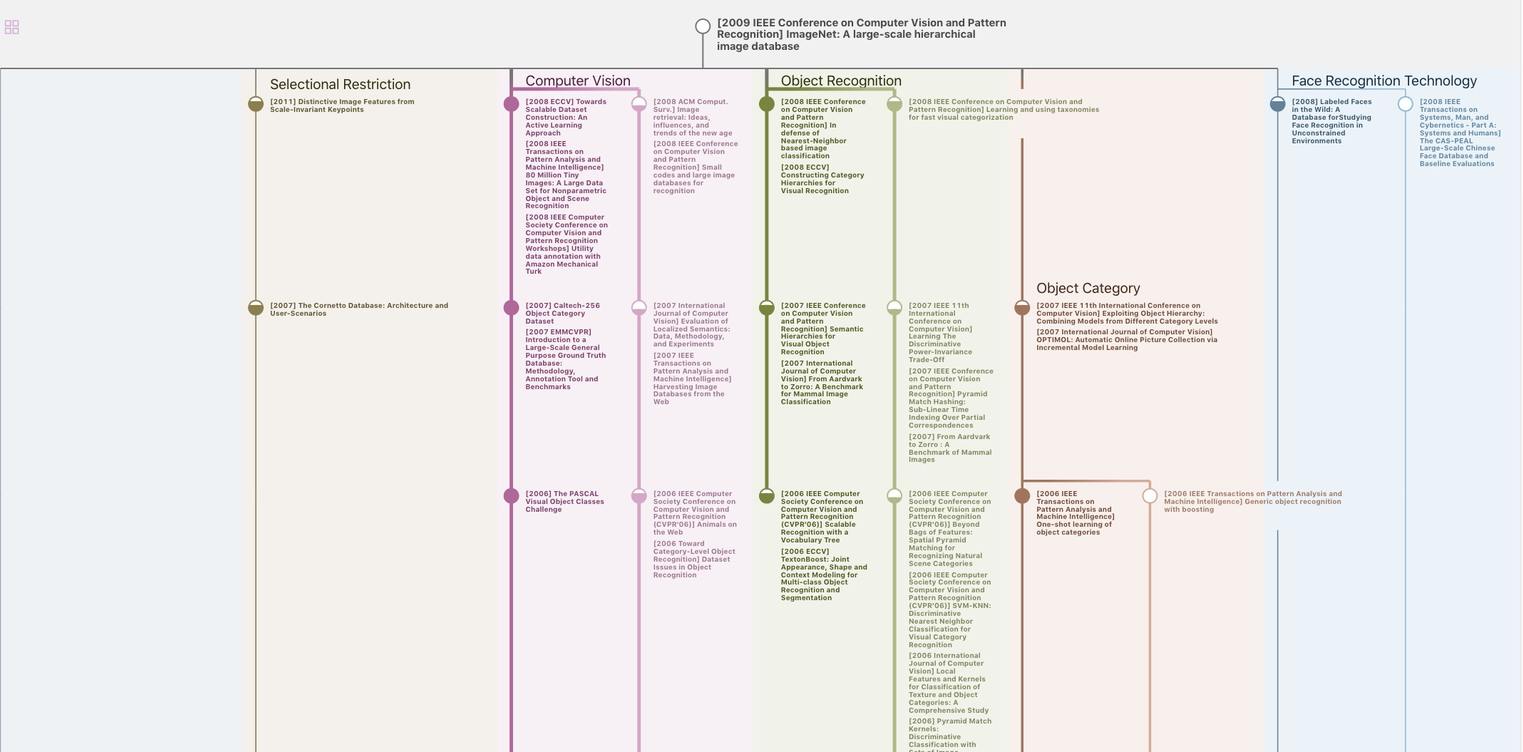
生成溯源树,研究论文发展脉络
Chat Paper
正在生成论文摘要