Classification of Indoor Point Clouds Using Multiviews.
Web3D '19: The 24th International Conference on 3D Web Technology LA CA USA July, 2019(2019)
摘要
We present an approach for classifying 3D point clouds of indoor environments using Convolutional Neural Network (CNN)-based image analysis for entropy-selected 3D views. Prior to classification, the 3D point clouds are clustered using either Density-based Spatial Clustering of Applications with Noise (DBSCAN) or k-means algorithms. We then use randomly sampled 3D point normal vectors as an entropy descriptor to calculate the direction and position of the virtual camera, which is placed around these clusters. It synthesizes 2D images of a given cluster from multiple views with positions and directions that have highest visual entropy. We then proceed to classify the images using a retrained CNN. We test our approach for classifying common office furniture items using both synthetic and actual 3D point clouds of typical indoor office spaces. The empirical results demonstrate that our approach is suited towards classifying specific indoor furniture objects. We also describe how our approach can be implemented as a lightweight component within a service-oriented system that is used for visualization and classification of 3D point clouds using a given Web3D tool. The resulting semantically-enriched 3D point clouds can further be used for digital indoor environment representations, with further use as base data for Building Information Modeling (BIM), Facility Management (FM), and Digital Twin (DT) applications.
更多查看译文
关键词
Viewpoint Entropy, Multiview Classification, Indoor Point Clouds, Semantic Enrichment
AI 理解论文
溯源树
样例
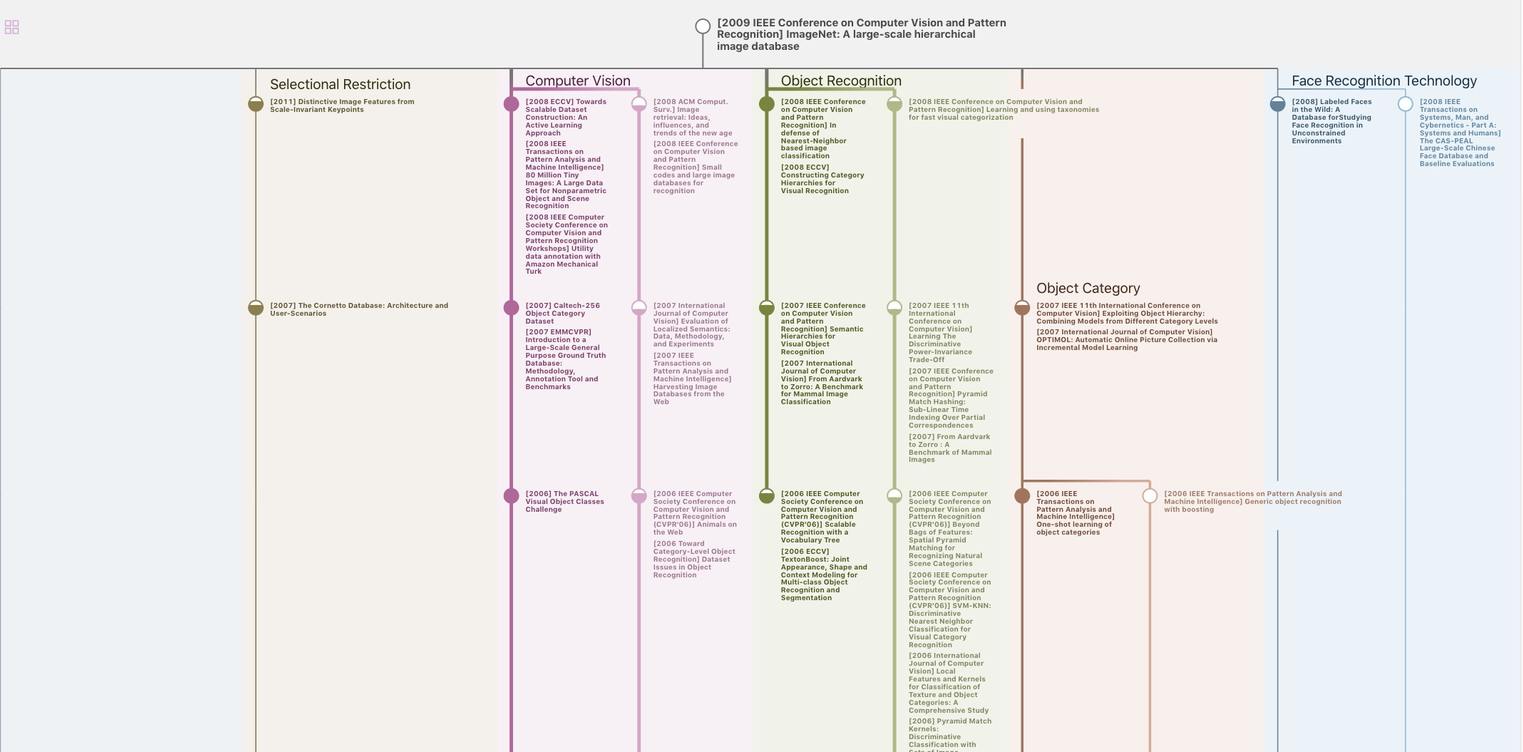
生成溯源树,研究论文发展脉络
Chat Paper
正在生成论文摘要