Visual Place Recognition Via Robust ℓ2-Norm Distance Based Holism and Landmark Integration
Proceedings of the AAAI Conference on Artificial Intelligence(2019)
摘要
Visual place recognition is essential for large-scale simultaneous localization and mapping (SLAM). Long-term robot operations across different time of the days, months, and seasons introduce new challenges from significant environment appearance variations. In this paper, we propose a novel method to learn a location representation that can integrate the semantic landmarks of a place with its holistic representation. To promote the robustness of our new model against the drastic appearance variations due to long-term visual changes, we formulate our objective to use non-squared ℓ 2 -norm distances, which leads to a difficult optimization problem that minimizes the ratio of the ℓ 2,1 -norms of matrices. To solve our objective, we derive a new efficient iterative algorithm, whose convergence is rigorously guaranteed by theory. In addition, because our solution is strictly orthogonal, the learned location representations can have better place recognition capabilities. We evaluate the proposed method using two large-scale benchmark data sets, the CMU-VL and Nord-land data sets. Experimental results have validated the effectiveness of our new method in long-term visual place recognition applications.
更多查看译文
AI 理解论文
溯源树
样例
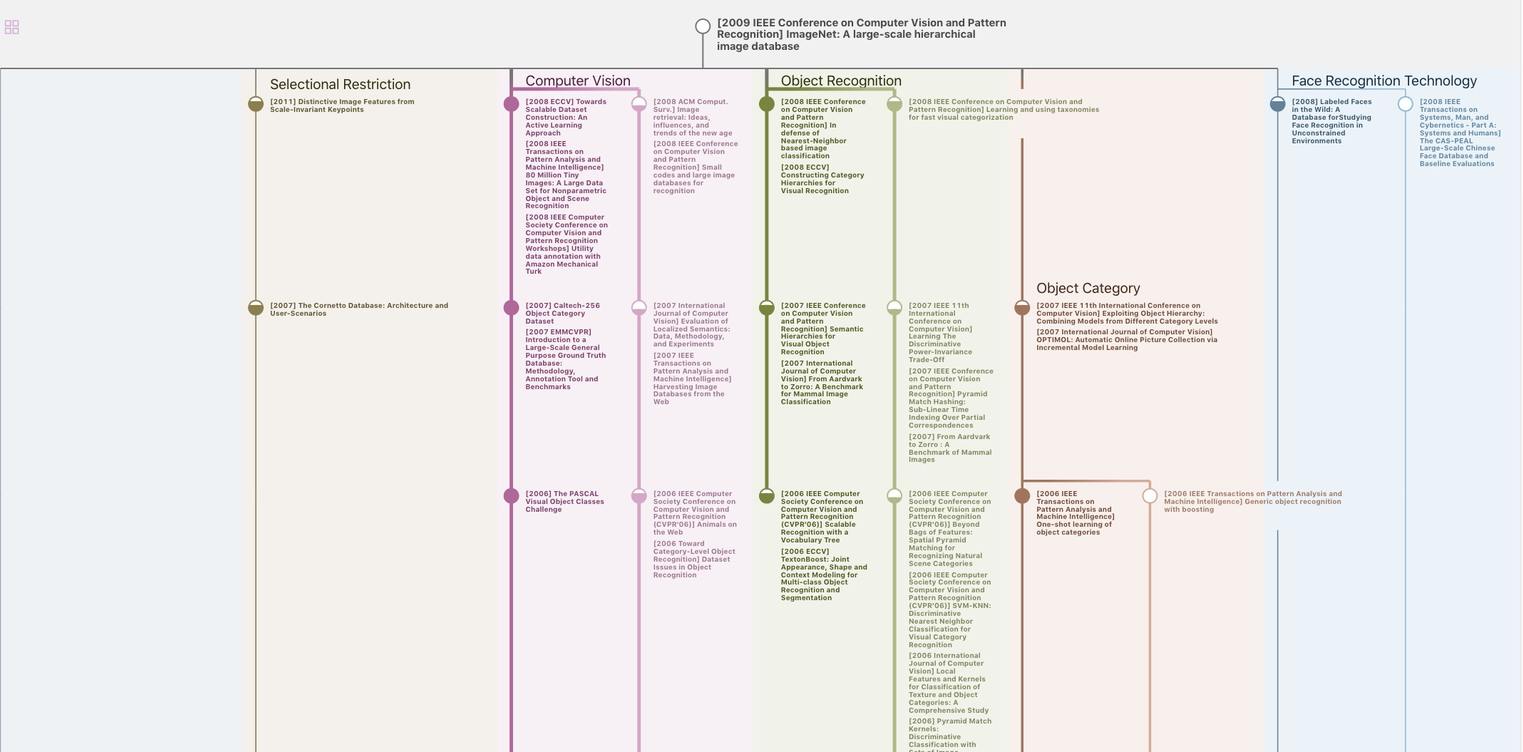
生成溯源树,研究论文发展脉络
Chat Paper
正在生成论文摘要