Identifying Bottlenecks in Practical SAT-Based Model Finding for First-Order Logic Ontologies with Datasets
AAAI(2019)
摘要
Satisfiability of first-order logic (FOL) ontologies is typically verified by translation to propositional satisfiability (SAT) problems, which is then tackled by a SAT solver. Unfortunately, SAT solvers often experience scalability issues when reasoning with FOL ontologies and even moderately sized datasets. While SAT solvers have been found to capably handle complex axiomatizations, finding models of datasets gets considerably more complex and time-intensive as the number of clause exponentially increases with increase in individuals and axiomatic complexity. We identify FOL definitions as a specific bottleneck and demonstrate via experiments that the presence of many defined terms of the highest arity significantly slows down model finding. We also show that removing optional definitions and substituting these terms by their definiens leads to a reduction in the number of clauses, which makes SAT-based model finding practical for over 100 individuals in a FOL theory.
更多查看译文
AI 理解论文
溯源树
样例
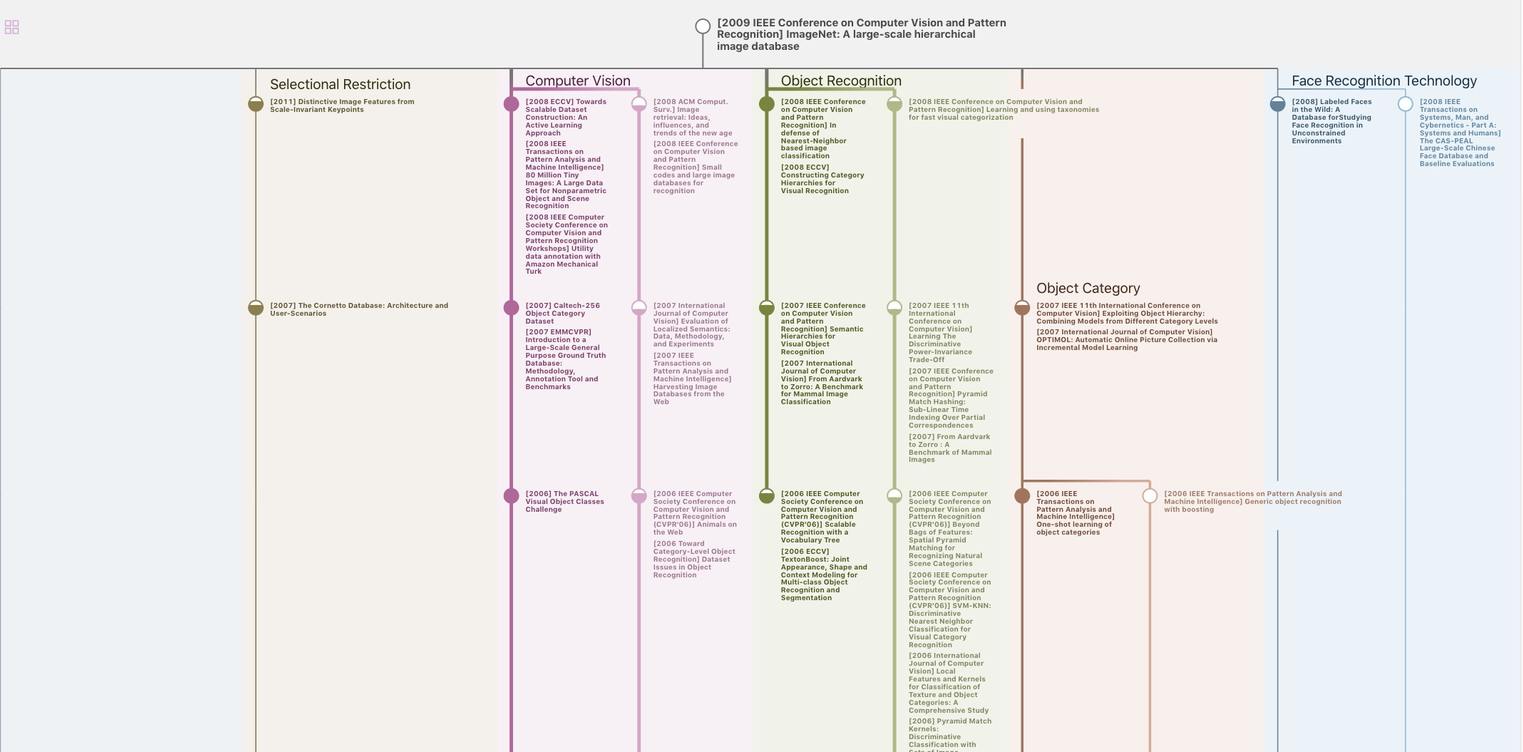
生成溯源树,研究论文发展脉络
Chat Paper
正在生成论文摘要