Efficient Deployment Of Predictive Analytics In Edge Gateways: Fall Detection Scenario
2019 IEEE 5TH WORLD FORUM ON INTERNET OF THINGS (WF-IOT)(2019)
摘要
Ambient Assisted Living (AAL) represents the most promising Internet of Things (IoT) application due to its relevance in the elders healthcare and improvement of their quality of life. Recently, the AAL IoT ecosystem has been enriched with promising technologies such as edge computing, which has demonstrated to be the best approach to overcome the demanding requirements of AAL and healthcare services by providing a reduction of the amount of data to transfer to the cloud, an improvement of the response time, and quality of experience. Also, the deployment of Artificial Intelligence (AI) technologies at the edge provides intelligence to improve the decision making timely. However, this approach has been scarcely studied in AAL scenarios and the few proposals based on deploying machine learning models at the edge lack efficiency, security, mechanisms of resource management, service management, and deployment, as well as a real and experimental AAL scenario. For these reasons, this paper proposes an innovative edge gateway architecture to support the deployment of deep learning (DL) models in AAL and healthcare scenarios efficiently. To do so, we have added a predictive analytics module to deploy the models. Since AI technologies demand more resources, a container-based virtualization technology is employed on the edge gateway to manage the limited resources, and provide security and lifecycle services management. The edge gateway performance was evaluated deploying a DL-based fall detection application on it. As a result, our approach improves the inference time compared to that based on the cloud in 34 seconds and to similar approaches in 8 seconds.
更多查看译文
关键词
Deep Learning, Edge Computing, Internet of Things, Predictive Analytics, Fall, AAL, virtualization
AI 理解论文
溯源树
样例
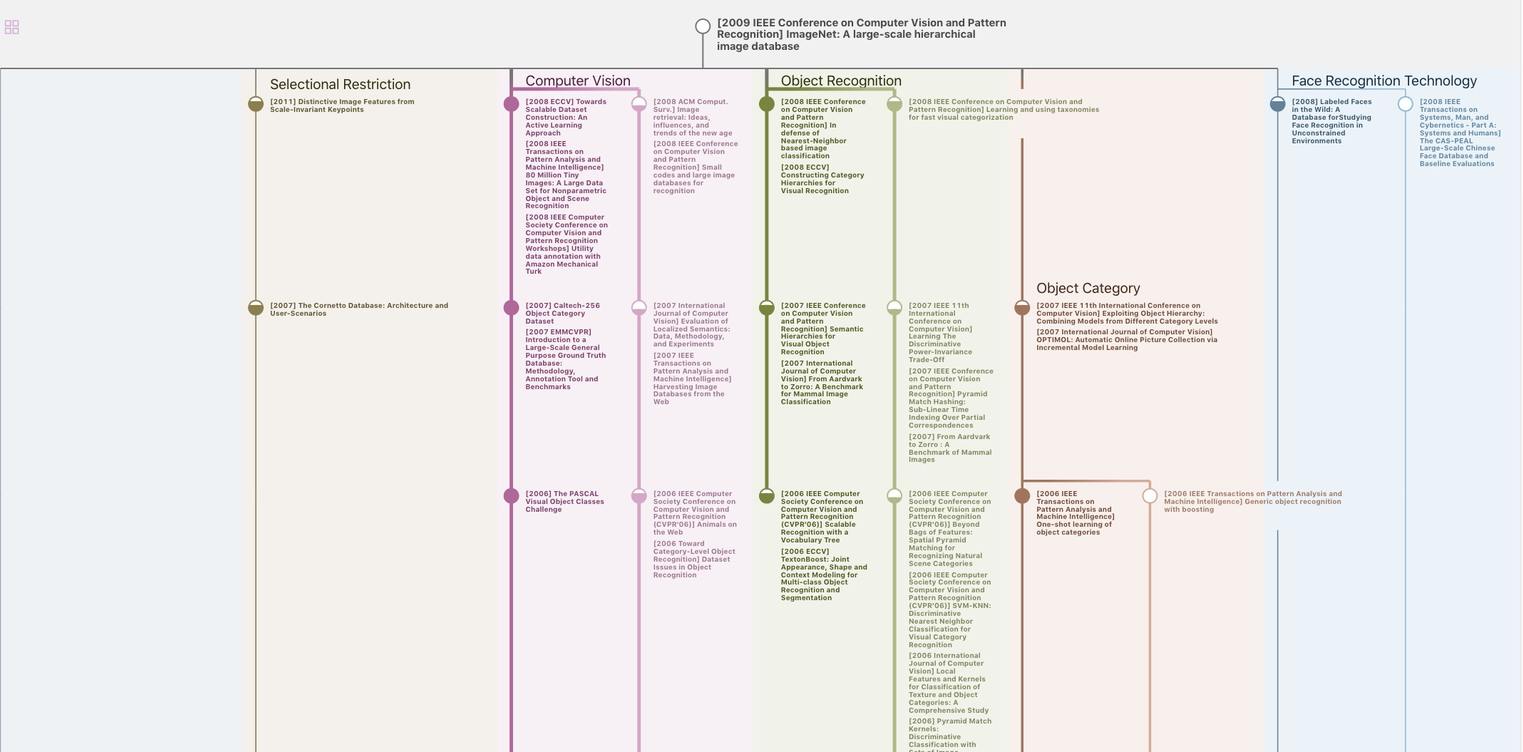
生成溯源树,研究论文发展脉络
Chat Paper
正在生成论文摘要