Understanding the Role of Style in E-commerce Shopping
KDD '19: The 25th ACM SIGKDD Conference on Knowledge Discovery and Data Mining Anchorage AK USA August, 2019(2019)
摘要
Aesthetic style is the crux of many purchasing decisions. When considering an item for purchase, buyers need to be aligned not only with the functional aspects (e.g. description, category, ratings) of an item's specification, but also its stylistic and aesthetic aspects (e.g. modern, classical, retro) as well. Style becomes increasingly important on e-commerce sites like Etsy, an online marketplace for handmade and vintage goods, where hundreds of thousands of items can differ by style and aesthetic alone. As such, it is important for industry recommender systems to properly model style when understanding shoppers' buying preference. In past work, because of its abstract nature, style is often approached in an unsupervised manner, represented by nameless latent factors or embeddings. As a result, there has been no previous work on predictive models nor analysis devoted to understanding how style, or even the presence of style, impacts a buyer's purchase decision. In this paper, we discuss a novel process by which we leverage 43 named styles given by merchandising experts in order to bootstrap large-scale style prediction and analysis of how style impacts purchase decision. We train a supervised, style-aware deep neural network that is shown to predict item style with high accuracy, while generating style-aware embeddings that can be used in downstream recommendation tasks. We share in our analysis, based on over a year's worth of transaction data and show that these findings are crucial to understanding how to more explicitly leverage style signal in industry-scale recommender systems.
更多查看译文
关键词
e-commerce, embeddings, style, supervised learning, user behavior
AI 理解论文
溯源树
样例
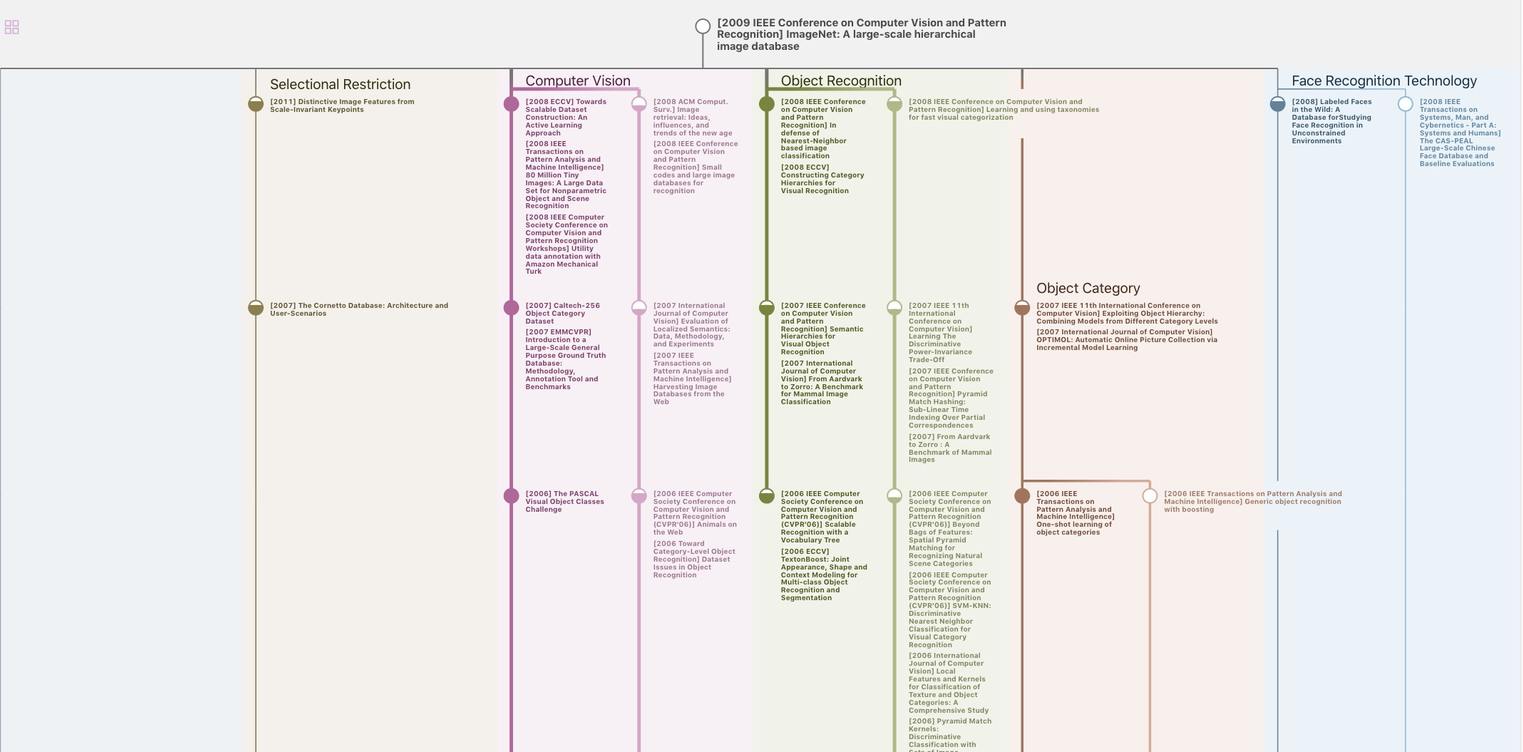
生成溯源树,研究论文发展脉络
Chat Paper
正在生成论文摘要