Conditional Random Field Enhanced Graph Convolutional Neural Networks
KDD(2019)
摘要
attention in recent years. Unlike the standard convolutional neural network, graph convolutional neural networks perform the convolutional operation on the graph data. Compared with the generic data, the graph data possess the similarity information between different nodes. Thus, it is important to preserve this kind of similarity information in the hidden layers of graph convolutional neural networks. However, existing works fail to do that. On the other hand, it is challenging to enforce the hidden layers to preserve the similarity relationship. To address this issue, we propose a novel CRF layer for graph convolutional neural networks to encourage similar nodes to have similar hidden features. In this way, the similarity information can be preserved explicitly. In addition, the proposed CRF layer is easy to compute and optimize. Therefore, it can be easily inserted into existing graph convolutional neural networks to improve their performance. At last, extensive experimental results have verified the effectiveness of our proposed CRF layer.
更多查看译文
关键词
conditional random field, graph convolutional neural networks, similarity
AI 理解论文
溯源树
样例
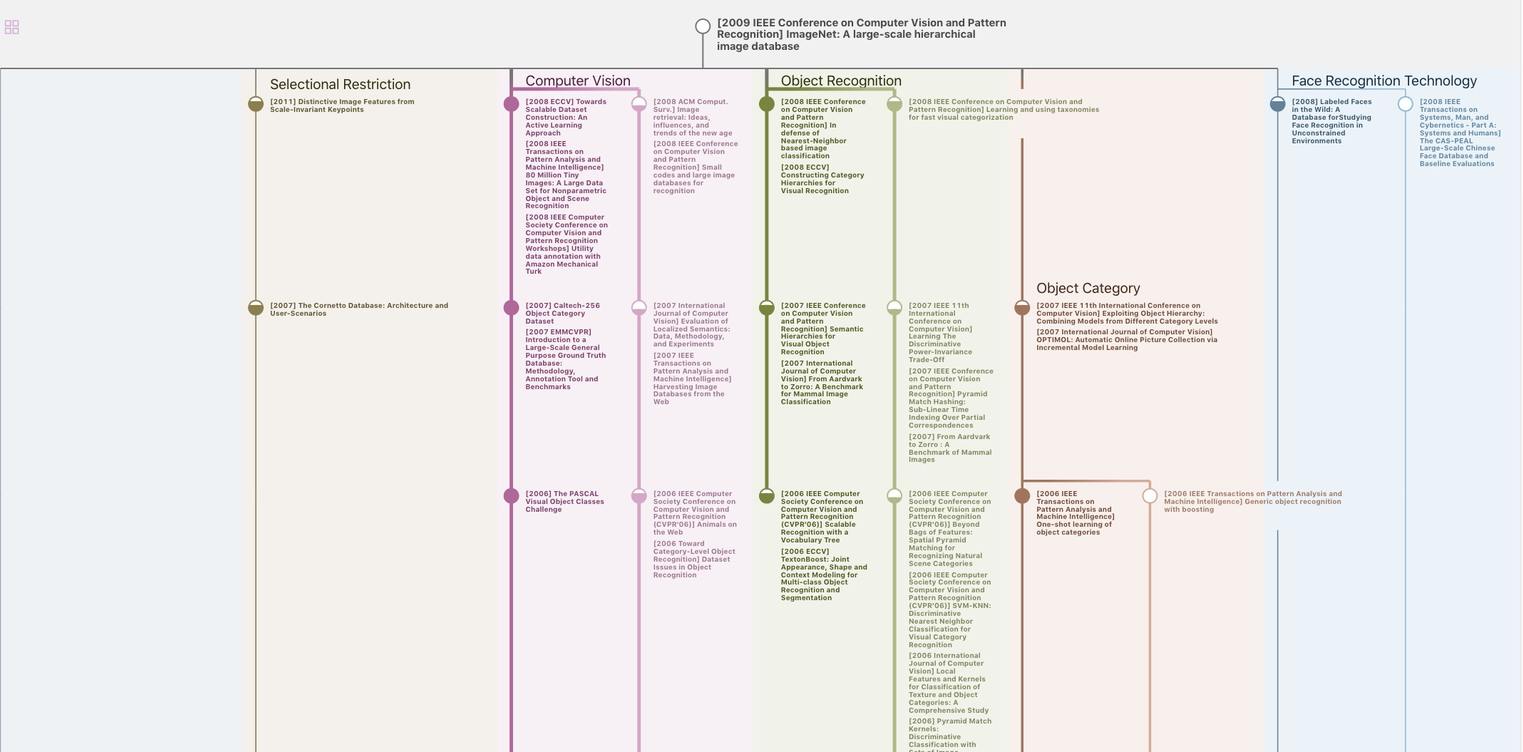
生成溯源树,研究论文发展脉络
Chat Paper
正在生成论文摘要