Text-Independent Speaker ID for Automatic Video Lecture Classification Using Deep Learning
Proceedings of the 2019 5th International Conference on Computing and Artificial Intelligence(2019)
摘要
This paper proposes to use acoustic features employing deep neural network (DNN) and convolutional neural network (CNN) models for classifying video lectures in a massive open online course (MOOC). The models exploit the voice pattern of the lecturer for identification and for classifying the video lecture according to the right speaker category. Filter bank and Mel frequency cepstral coefficient (MFCC) feature along with first and second order derivatives (Δ/ΔΔ) are used as input features to the proposed models. These features are extracted from the speech signal which is obtained from the video lectures by separating the audio from the video using FFmpeg.
The deep learning models are evaluated using precision, recall, and F1 score and the obtained accuracy is compared for both acoustic features with traditional machine learning classifiers for speaker identification. A significant improvement of 3% to 7% classification accuracy is achieved over the DNN and twice to that of shallow machine learning classifiers for 2D-CNN with MFCC. The proposed 2D-CNN model with an F1 score of 85.71% for text-independent speaker identification makes it plausible to use speaker ID as a classification approach for organizing video lectures automatically in a MOOC setting.
更多查看译文
关键词
2D-CNN, DNN, MFCC Filter banks, MOOC, Speaker identification, deep learning, video classification
AI 理解论文
溯源树
样例
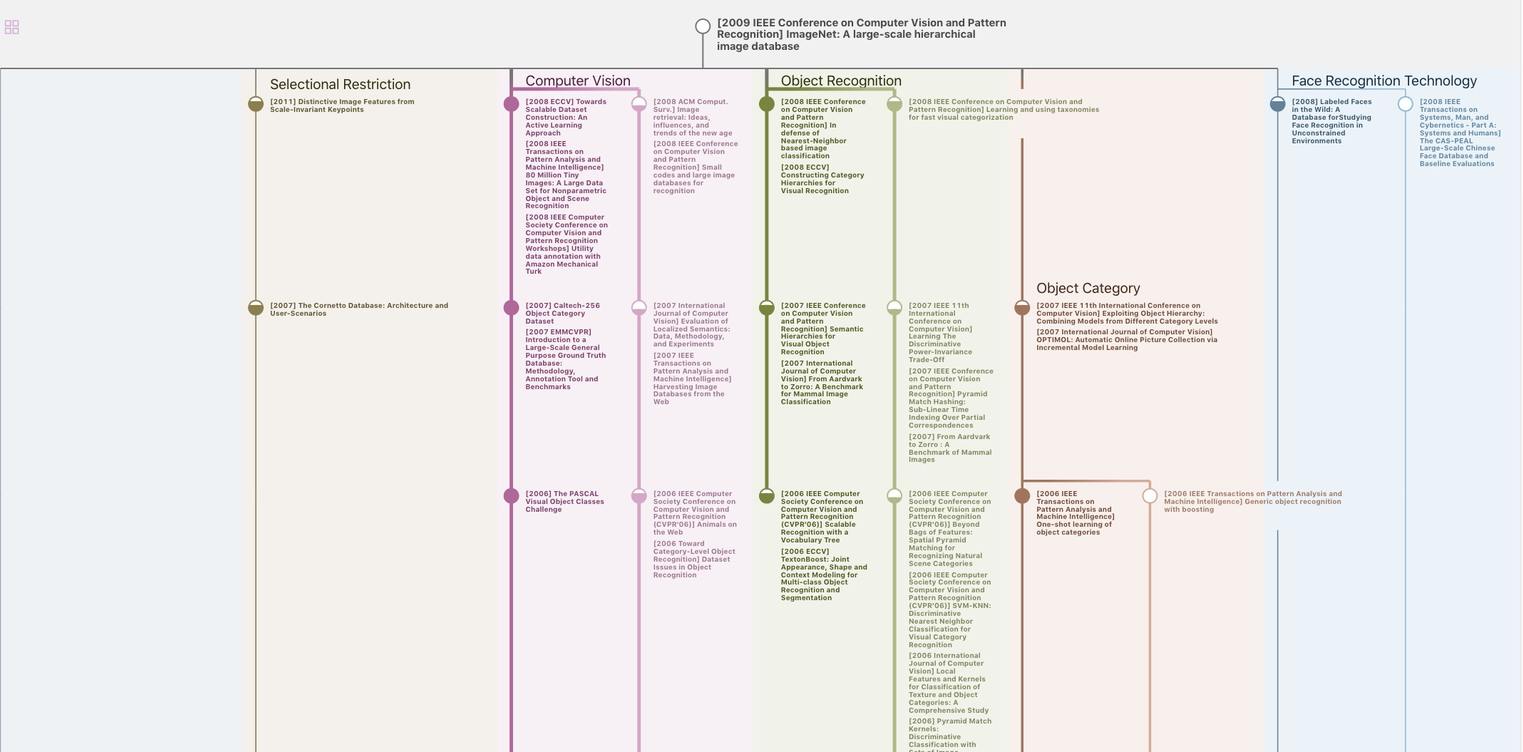
生成溯源树,研究论文发展脉络
Chat Paper
正在生成论文摘要