Continuous Time Nonstationary Correlation Models for Sparse Longitudinal Data
Model assisted statistics and applications(2019)
摘要
In this paper, we present a new continuous time model for nonstationary correlation structures for longitudinal data. This model, which provides a continuous time analogue to the antedependence model and is thus referred to as the continuous antedependence (CAD) model, is intended to provide more refined correlation models for longitudinal data and to better accommodate sparse (or highly unbalanced) data. A key component of this model is the 'nonstationarity function' which describes nonstationarity as a unidimensional function of time and has an interesting time expansion/contraction interpretation. Focusing on a Markovian version of the model, we develop a novel nonlinear regression model providing nonlinear least square estimators of model parameters. Both unstructured (for nonparametric estimation) and structured versions of the model are presented. We apply the proposed approach to data from the Multicenter AIDS Clinical Study (MACS), with a focus on inference for the nonstationarity function. In simulation studies, we show good properties (low finite sample bias, and high convergence rates and efficiency) of the proposed unstructured model estimator, which compare favorably to those of an alternative maximum likelihood estimator, particularly in sparse data situations.
更多查看译文
关键词
High-Dimensional Data,Deep Learning,Anomaly Detection,Neural Networks,Video Analysis
AI 理解论文
溯源树
样例
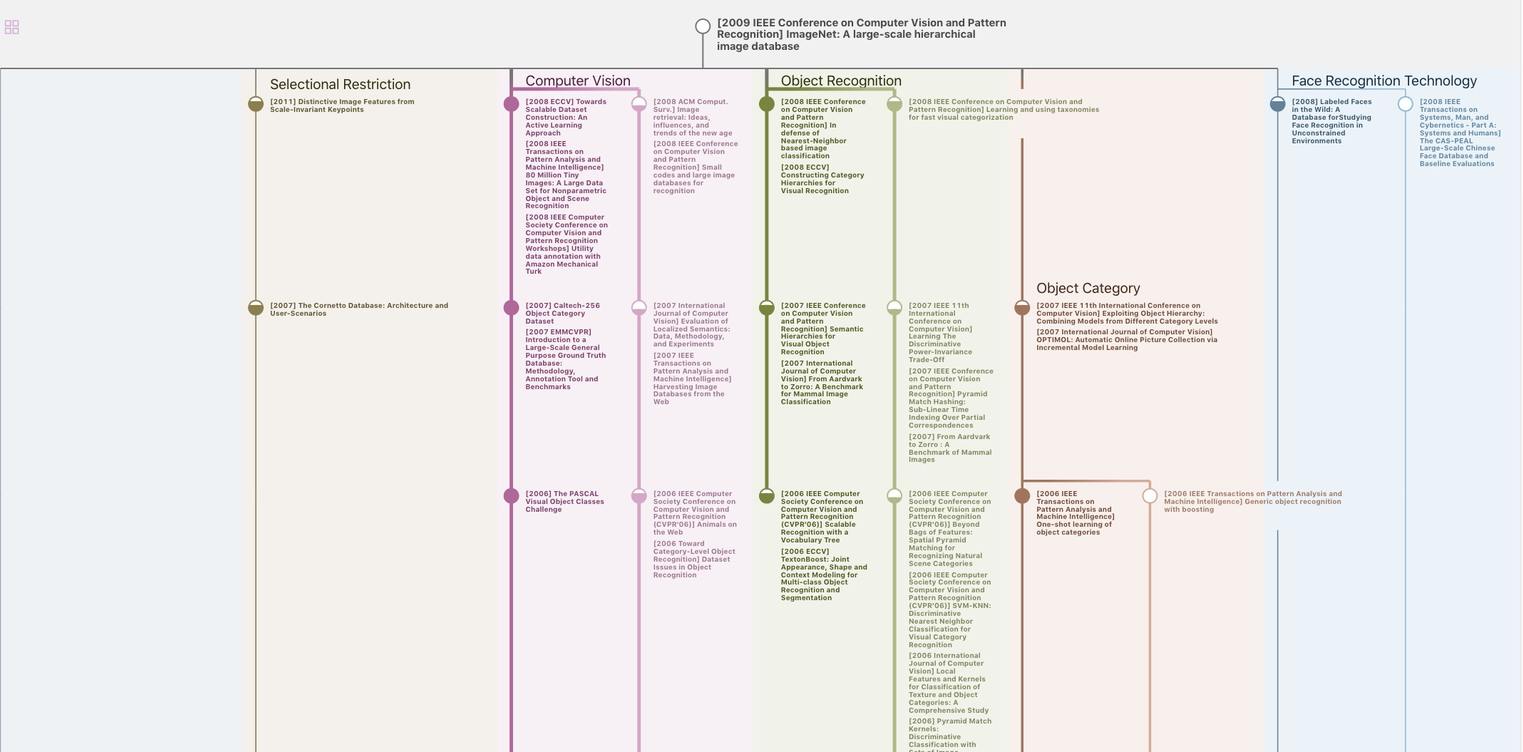
生成溯源树,研究论文发展脉络
Chat Paper
正在生成论文摘要