Multi-level Weight Indexing Scheme for Memory-Reduced Convolutional Neural Network
2019 IEEE International Conference on Artificial Intelligence Circuits and Systems (AICAS)(2019)
摘要
Targeting the resource-limited intelligent mobile systems, in this paper, we present a multi-level weight indexing method that relaxes the memory requirements for realizing the convolutional neural networks (CNNs). In contrast that the previous works are only focusing on the positions of unpruned weights, the proposed work considers the consecutive pruned positions to generate the group-level validations. Denoting the survived indices only for the valid groups, the proposed multi-level indexing scheme reduces the amount of indexing data. In addition, we introduce the indexing-aware multi-level pruning and indexing methods with variable group sizes, which can further optimize the memory overheads. For the same pruning factor, as a result, the memory size for storing the indexing information is remarkably reduced by up to 81%, leading to the practical CNN architecture for intelligent mobile devices.
更多查看译文
关键词
convolutional neural network,weight indexing,weight pruning,memory-reduced architecture
AI 理解论文
溯源树
样例
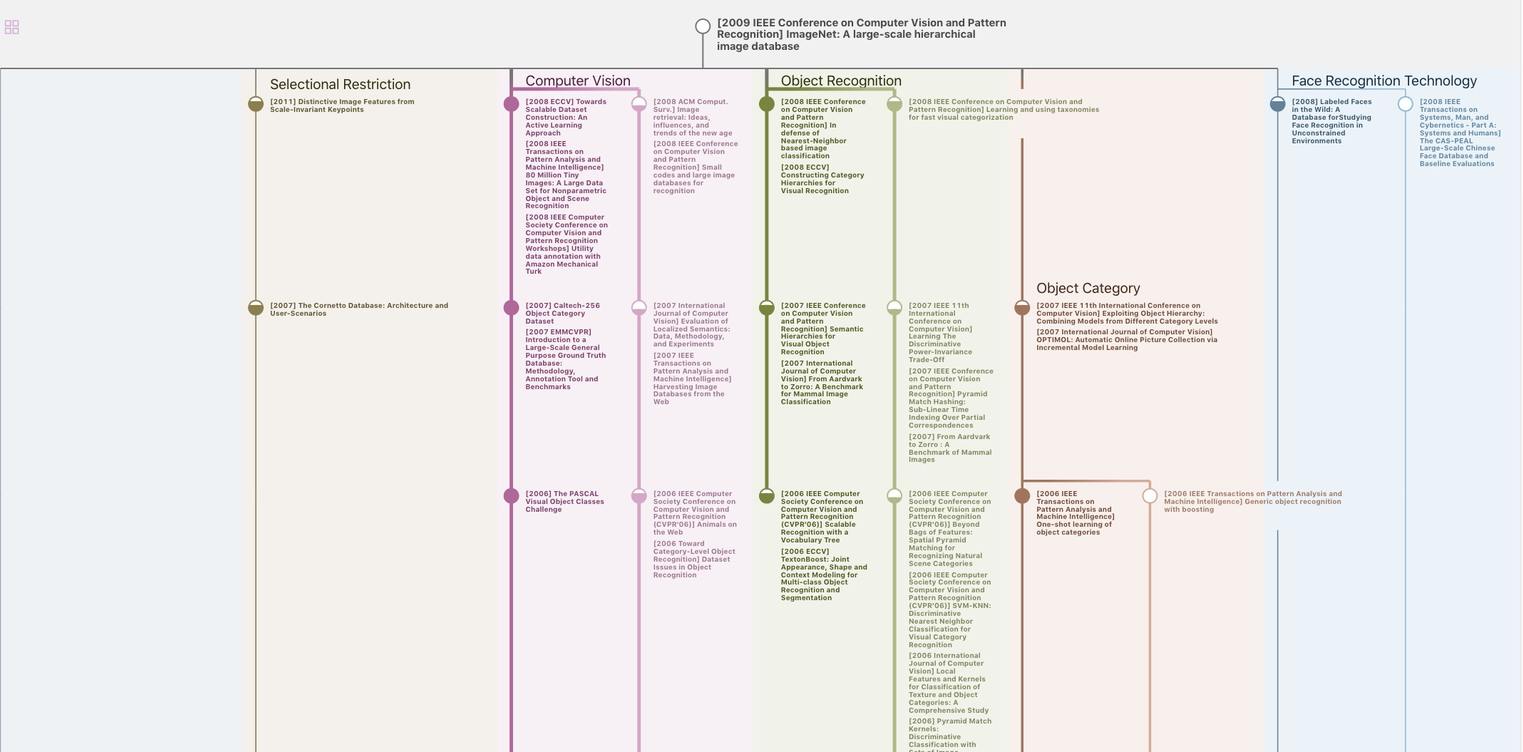
生成溯源树,研究论文发展脉络
Chat Paper
正在生成论文摘要