Data Distribution Based Weighted Extreme Learning Machine
Proceedings of the 2019 4th International Conference on Machine Learning Technologies(2019)
摘要
As an effective learning approach, extreme learning machine (ELM) has been applied to multiple fields with its faster learning speed and better generalization performance. To solve the classification problem especially the data with imbalanced class distribution, some solutions are proposed based on cost sensitive ELM. However, the existing methods only consider the effect of the misclassified sample on the class to which it belongs but ignoring the overall loss. In this paper, we propose a new weighting scheme used in ELM, data distribution based weighted extreme learning machine (D-WELM) for binary and multiclass classification problems with imbalanced data distributions. It is noteworthy that the proposed method maintains the advantages from original ELM. D-WELM considers not only the effect of sample sizes in each class, but also class distribution. Meanwhile, this work takes overall loss into account. Experimental results show that D-WELM can achieve better performance for classification problems with imbalanced data distributions than original ELM, weighted ELM(WELM) and class-specific cost regulation ELM (CCR-ELM). In addition, D-WELM with kernel can also get good performance.
更多查看译文
关键词
Cost sensitive, Extreme learning machine, Imbalanced data distribution
AI 理解论文
溯源树
样例
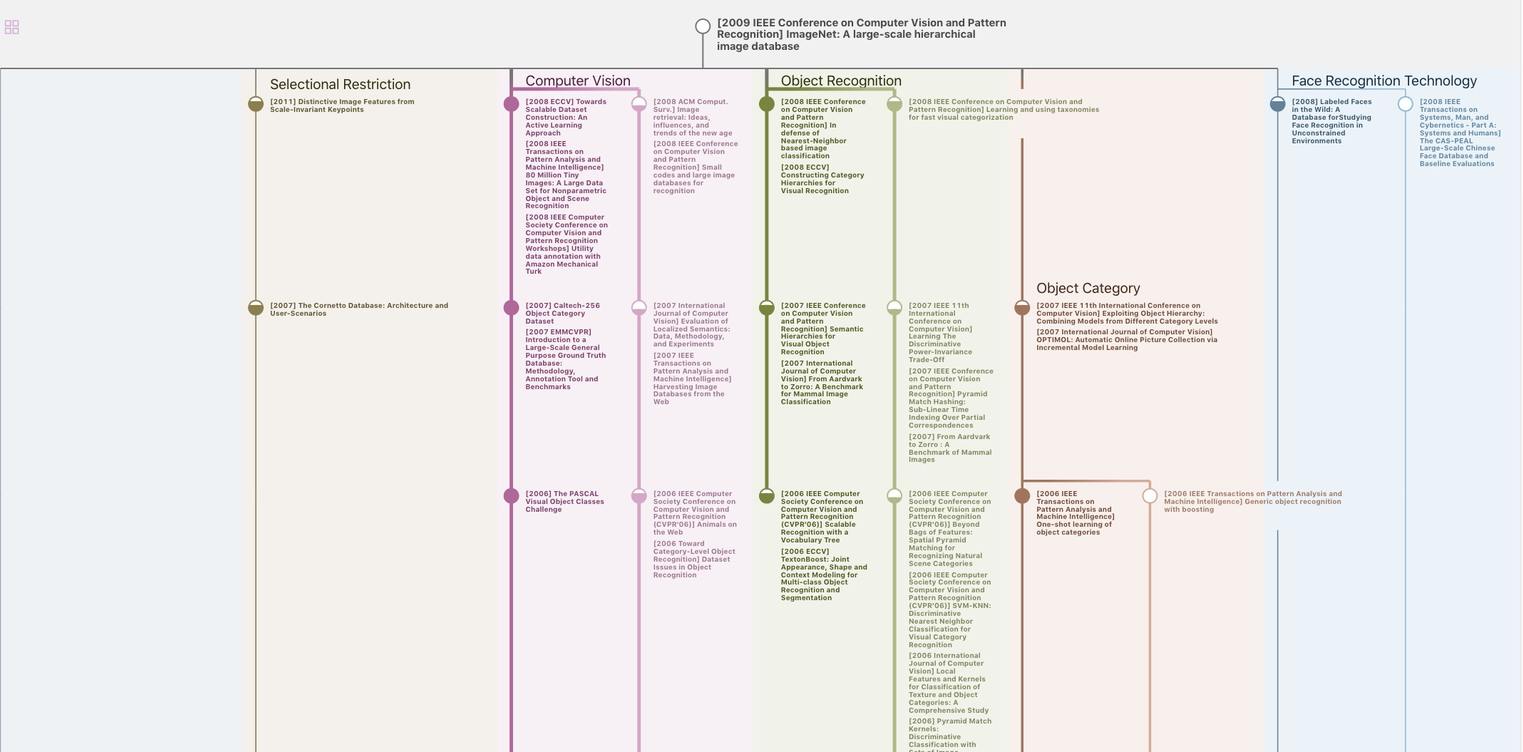
生成溯源树,研究论文发展脉络
Chat Paper
正在生成论文摘要