Adversarial Nuclei Segmentation on H&E Stained Histopathology Images
2019 IEEE International Symposium on INnovations in Intelligent SysTems and Applications (INISTA)(2019)
摘要
Computer aided methods in pathology are advancing rapidly. Problems like segmentation, classification and detection of pathology images are solved with machine learning and image processing techniques. State-of-the-art methods in nuclei segmentation problem include supervised deep learning techniques. However, labeling process of pathology images is an expensive and time consuming process. In this work, nuclei segmentation problem is formulated as image-to-image translation problem and using Cycle-Consistent Generative Adversarial Networks, an unsupervised segmentation scheme is proposed for hematoxylin & eosin stained histopathology data.
更多查看译文
关键词
GAN,Cycle,Segmentation,Nuclei,Cell,pathology,Unsupervised,Adversary
AI 理解论文
溯源树
样例
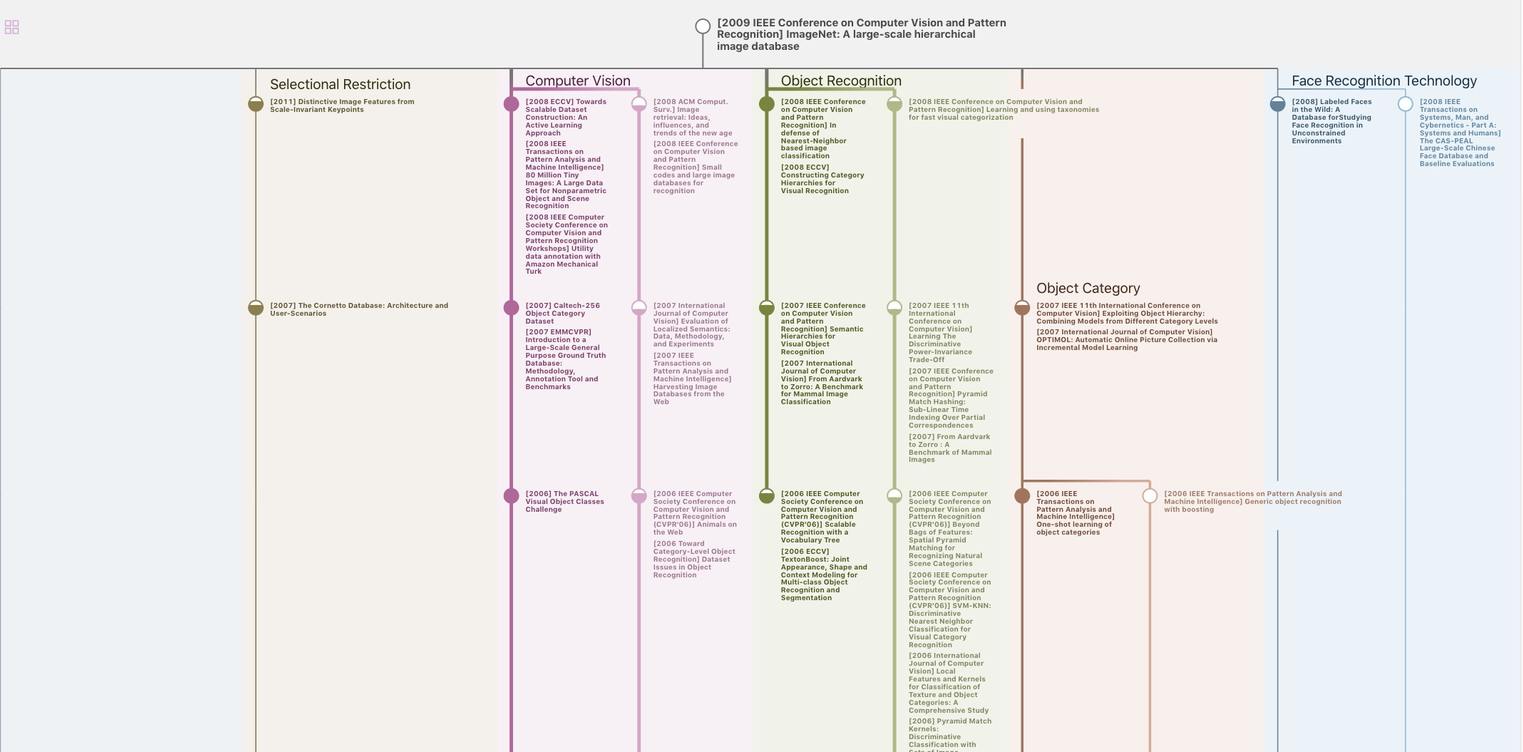
生成溯源树,研究论文发展脉络
Chat Paper
正在生成论文摘要