Disentangling Propagation And Generation For Video Prediction
2019 IEEE/CVF INTERNATIONAL CONFERENCE ON COMPUTER VISION (ICCV 2019)(2019)
摘要
A dynamic scene has two types of elements: those that move fluidly and can be predicted from previous frames, and those which are disoccluded (exposed) and cannot be extrapolated. Prior approaches to video prediction typically learn either to warp or to hallucinate future pixels, but not both. In this paper, we describe a computational model for high-fidelity video prediction which disentangles motion-specific propagation from motion-agnostic generation. We introduce a confidence-aware warping operator which gates the output of pixel predictions from a flow predictor for non-occluded regions and from a context encoder for occluded regions. Moreover, in contrast to prior works where confidence is jointly learned with flow and appearance using a single network, we compute confidence after a warping step, and employ a separate network to inpaint exposed regions. Empirical results on both synthetic and real datasets show that our disentangling approach provides better occlusion maps and produces both sharper and more realistic predictions compared to strong baselines.
更多查看译文
关键词
dynamic scene,motion-specific propagation,motion-agnostic generation,confidence-aware warping operator,pixel predictions,disentangling approach,video prediction
AI 理解论文
溯源树
样例
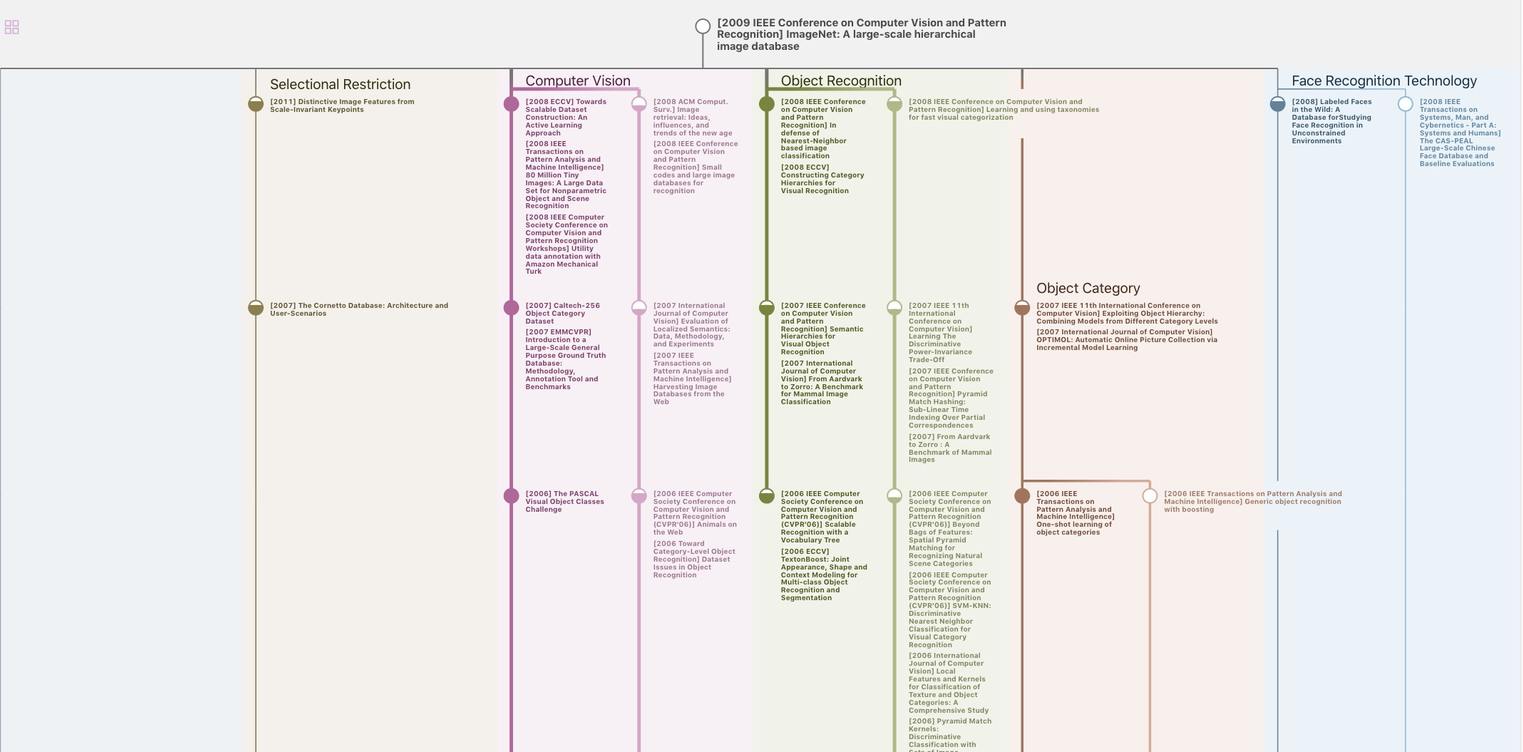
生成溯源树,研究论文发展脉络
Chat Paper
正在生成论文摘要