Attribute-Guided Coupled GAN for Cross-Resolution Face Recognition
2019 IEEE 10th International Conference on Biometrics Theory, Applications and Systems (BTAS)(2019)
摘要
In this paper, we propose a novel attribute-guided cross-resolution (low-resolution to high-resolution) face recognition framework that leverages a coupled generative adversarial network (GAN) structure with adversarial training to find the hidden relationship between the low-resolution and high-resolution images in a latent common embedding subspace. The coupled GAN framework consists of two sub-networks, one dedicated to the low-resolution domain and the other dedicated to the high-resolution domain. Each sub-network aims to find a projection that maximizes the pair-wise correlation between the two feature domains in a common embedding subspace. In addition to projecting the images into a common subspace, the coupled network also predicts facial attributes to improve the cross-resolution face recognition. Specifically, our proposed coupled framework exploits facial attributes to further maximize the pair-wise correlation by implicitly matching facial attributes of the low and high-resolution images during the training, which leads to a more discriminative embedding subspace resulting in performance enhancement for cross-resolution face recognition. The efficacy of our approach compared with the state-of-the-art is demonstrated using the LFWA, Celeb-A, SCFace and UCCS datasets.
更多查看译文
关键词
cross-resolution face recognition,novel attribute-guided cross-resolution,coupled generative adversarial network structure,high-resolution images,latent common embedding subspace,coupled GAN framework,low-resolution domain,high-resolution domain,pair-wise correlation,coupled network,coupled framework exploits facial attributes,low resolution images
AI 理解论文
溯源树
样例
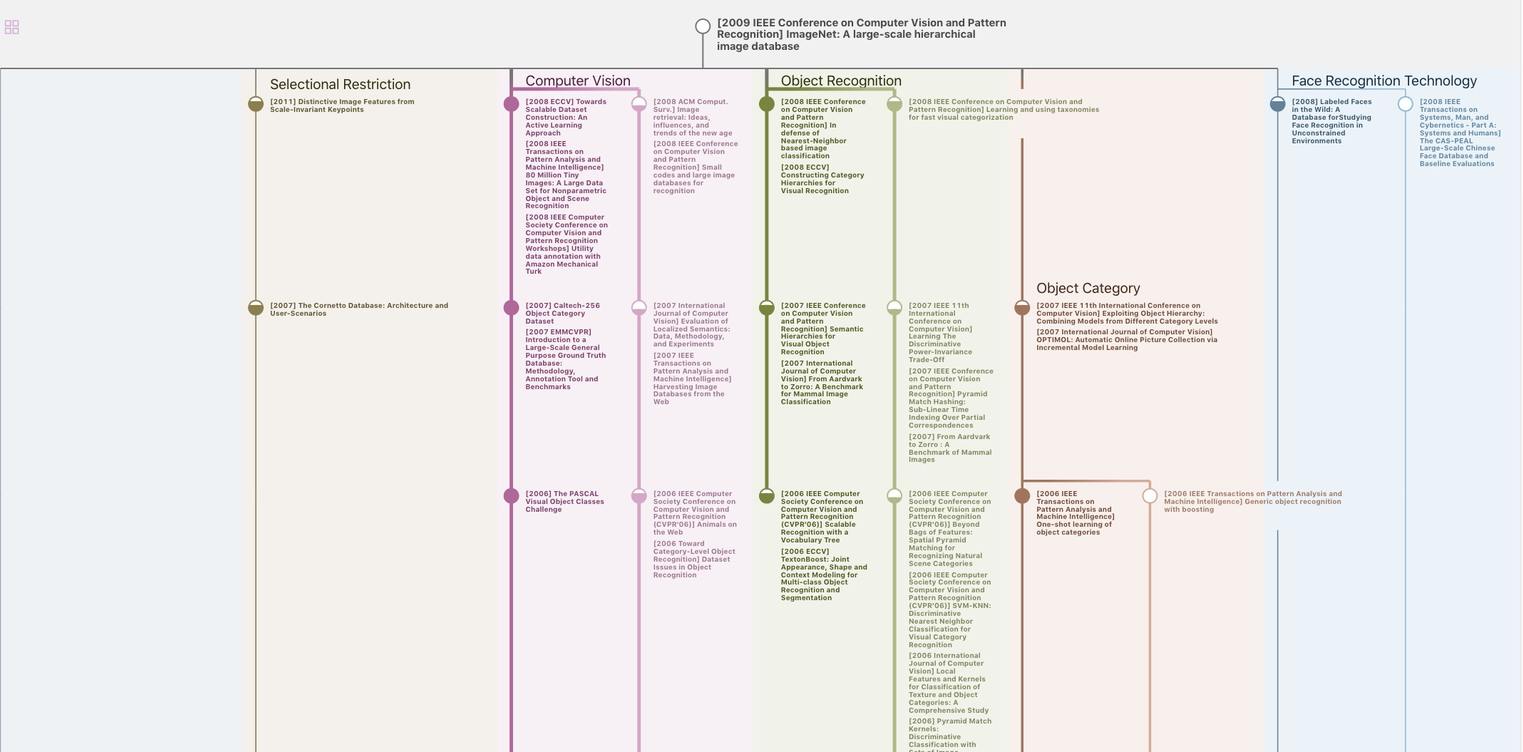
生成溯源树,研究论文发展脉络
Chat Paper
正在生成论文摘要