Zero-Shot Deep Hashing and Neural Network Based Error Correction for Face Template Protection
2019 IEEE 10th International Conference on Biometrics Theory, Applications and Systems (BTAS)(2019)
摘要
In this paper, we present a novel architecture that integrates a deep hashing framework with a neural network decoder (NND) for application to face template protection. It improves upon existing face template protection techniques to provide better matching performance with one-shot and multi-shot enrollment. A key novelty of our proposed architecture is that the framework can also be used with zero-shot enrollment. This implies that our architecture does not need to be re-trained even if a new subject is to be enrolled into the system. The proposed architecture consists of two major components: a deep hashing (DH) component, which is used for robust mapping of face images to their corresponding intermediate binary codes, and a NND component, which corrects errors in the intermediate binary codes that are caused by differences in the enrollment and probe biometrics due to factors such as variation in pose, illumination, and other factors. The final binary code generated by the NND is then cryptographically hashed and stored as a secure face template in the database. The efficacy of our approach with zero-shot, one-shot, and multi-shot enrollments is shown for CMU-PIE, Extended Yale B, WVU multimodal and Multi-PIE face databases. With zero-shot enrollment, the system achieves approximately 85% genuine accept rates (GAR) at 0.01% false accept rate (FAR), and with one-shot and multi-shot enrollments, it achieves approximately 99.95% GAR at 0.01% FAR, while providing a high level of template security.
更多查看译文
关键词
zero-shot deep hashing,deep hashing framework,neural network decoder,face template protection,multishot enrollment,zero-shot enrollment,deep hashing component,face images,intermediate binary codes,NND component,probe biometrics,secure face template,MultiPIE face databases,template security,neural network based error correction
AI 理解论文
溯源树
样例
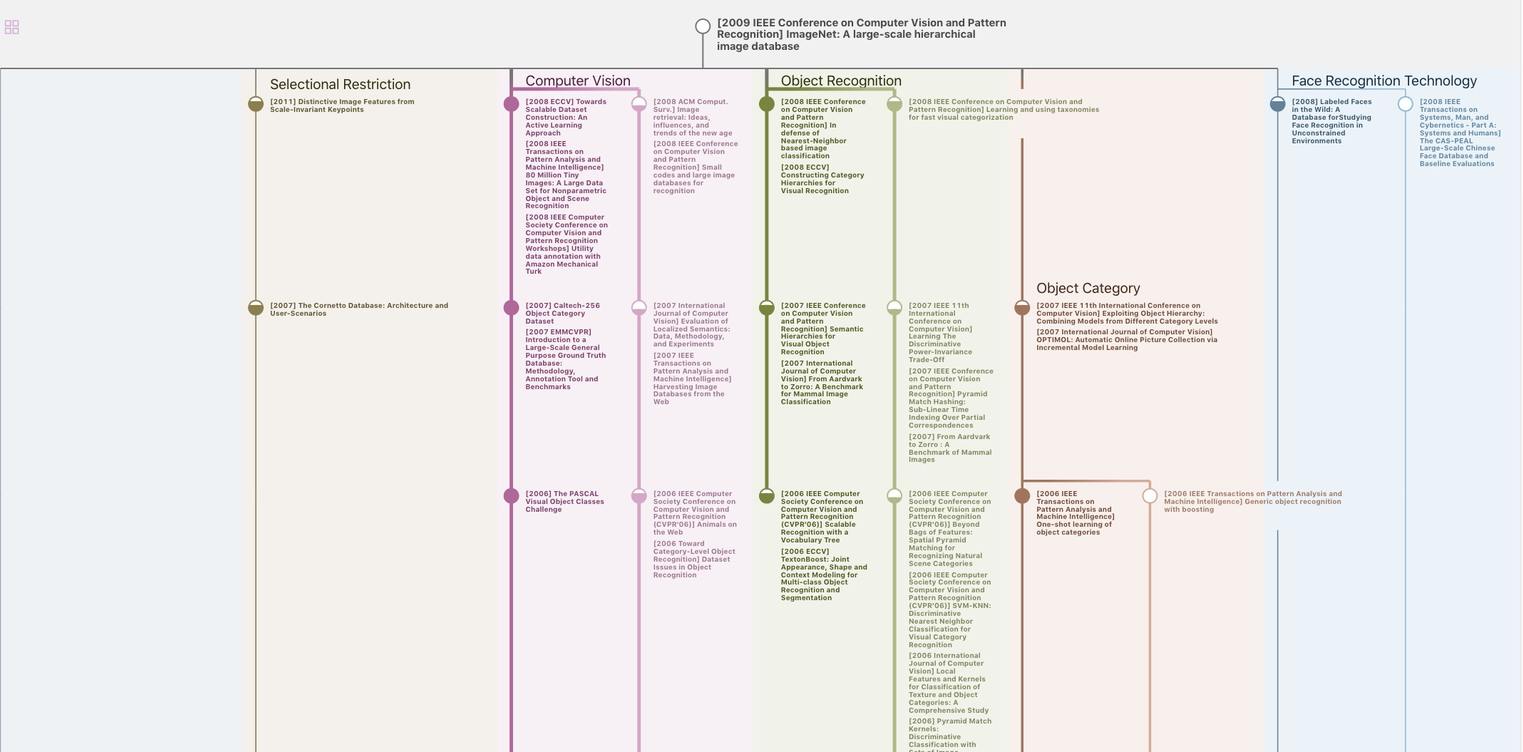
生成溯源树,研究论文发展脉络
Chat Paper
正在生成论文摘要