Multi-View Multi-Label Learning with View-Specific Information Extraction.
IJCAI(2019)
摘要
Multi-view multi-label learning serves an important framework to learn from objects with diverse representations and rich semantics. Existing multi-view multi-label learning techniques focus on exploiting shared subspace for fusing multiview representations, where helpful view-specific information for discriminative modeling is usually ignored. In this paper, a novel multi-view multi-label learning approach named SIMM is proposed which leverages shared subspace exploitation and view-specific information extraction. For shared subspace exploitation, SIMM jointly minimizes confusion adversarial loss and multi-label loss to utilize shared information from all views. For view-specific information extraction, SIMM enforces an orthogonal constraint w.r.t. the shared subspace to utilize view-specific discriminative information. Extensive experiments on real-world data sets clearly show the favorable performance of SIMM against other state-of-the-art multi-view multi-label learning approaches.
更多查看译文
AI 理解论文
溯源树
样例
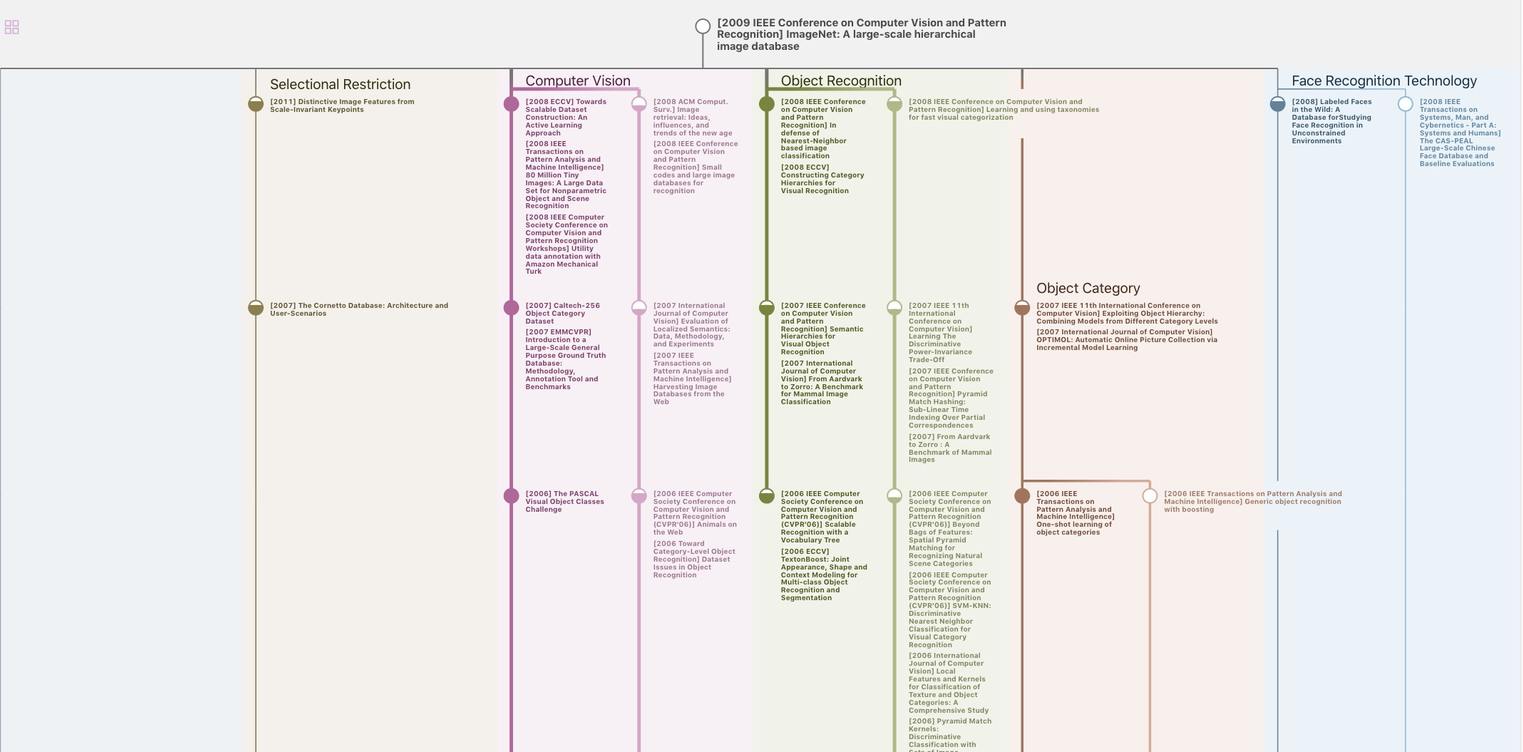
生成溯源树,研究论文发展脉络
Chat Paper
正在生成论文摘要