Robust Facial Alignment with Internal Denoising Auto-Encoder
2019 16th Conference on Computer and Robot Vision (CRV)(2019)
Abstract
The development of facial alignment models is growing rapidly thanks to the availability of large facial landmarked datasets and powerful deep learning models. However, important challenges still remain for facial alignment models to work on images under extreme conditions, such as severe occlusions or large variations in pose and illumination. Current attempts to overcome this limitation have mainly focused on building robust feature extractors with the assumption that the model will be able to discard the noise and select only the meaningful features. However, such an assumption ignores the importance of understanding the noise that characterizes unconstrained images, which has been shown to benefit computer vision models if used appropriately on the learning strategy. Thus, in this paper we investigate the introduction of specialized modules for noise detection and removal, in combination with our state-of-the-art facial alignment module and show that this leads to improved robustness both to synthesized noise and in-the-wild conditions. The proposed model is built by combining two major subnetworks: internal image denoiser (based on the Auto-Encoder architecture) and facial landmark localiser (based on the inception-resnet architecture). Our results on the 300-W and Menpo datasets show that our model can effectively handle different types of synthetic noise, which also leads to enhanced robustness in real-world unconstrained settings, reaching top state-of-the-art accuracy.
MoreTranslated text
Key words
Facial Alignment,Auto Encoder,Noise Normalization
AI Read Science
Must-Reading Tree
Example
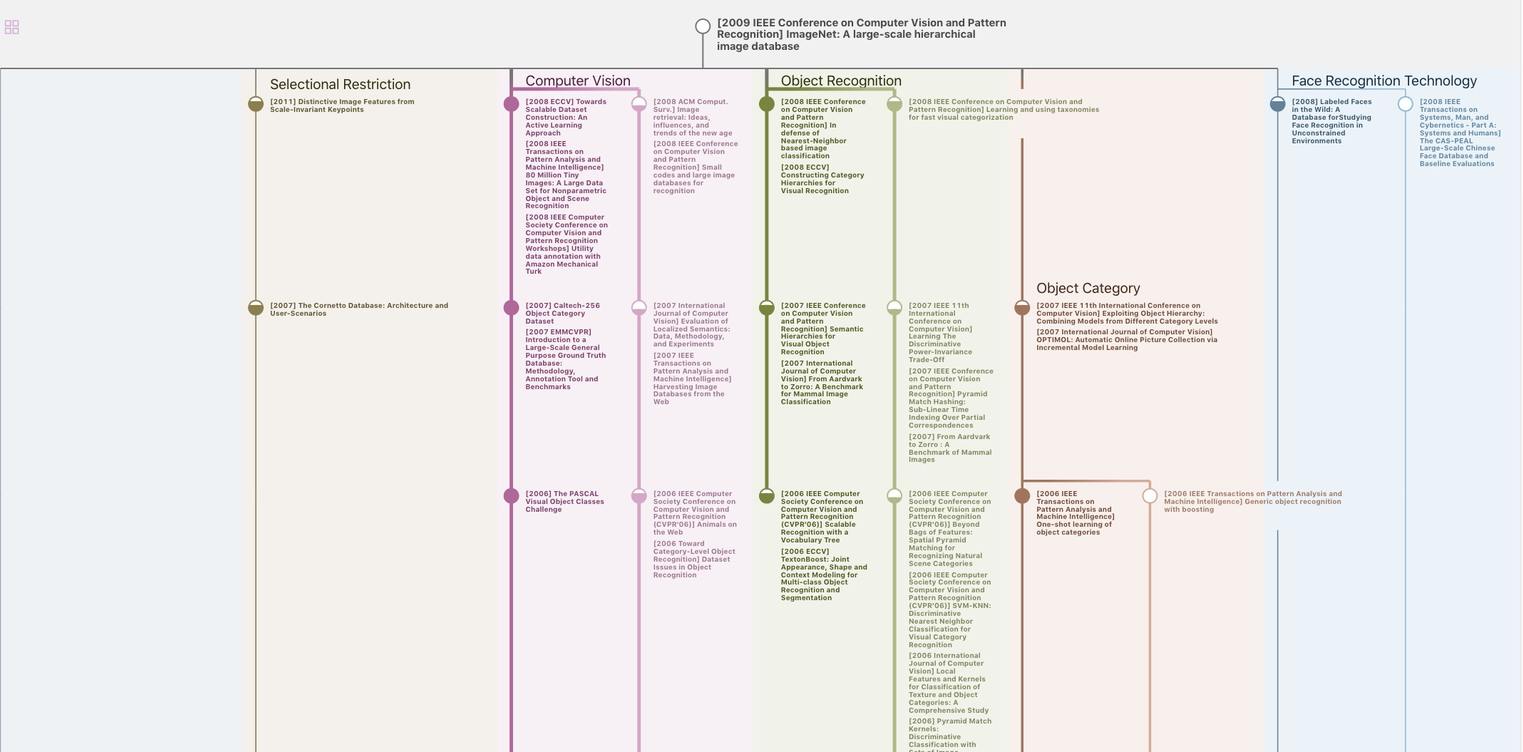
Generate MRT to find the research sequence of this paper
Chat Paper
Summary is being generated by the instructions you defined