Obesity Entity Extraction from Real Outpatient Records: When Learning-Based Methods Meet Small Imbalanced Medical Data Sets
2019 IEEE 32nd International Symposium on Computer-Based Medical Systems (CBMS)(2019)
摘要
The postoperative health status of an obesity patient indicates the outcome of the surgical treatment. By each postoperative revisit, physicians need to go through the previous patient records to recall the patient status and to evaluate the postoperative risk of readmission. In order to support in this process, we develop a method to extract indicators and to analyse weight changes, so that potential complications and risks of clinical readmission can be recognized timely. In this paper, we will compare two approaches that are based on traditional machine learning and neural networks. Relevant aspects referring to a health status change or treatment-relevant aspects are extracted from the outpatient medical records as they are generated for each postoperative revisit. The performance of traditional machine learning on the task of obesity-related entity extraction is compared with one variation of attentive recurrent neural networks. The ensemble classifier of binary attentive bi-LSTM with the data balancing using conditional generative adversarial networks (CGAN) has achieved F1 measure of 86.5% on the task of classification of eight classes of obesity-related entities. We conclude that for processing a small data set using neural networks, a data balancing method should firstly be applied to achieve an extended corpus and a general representation, which can apparently increase the differentiability of the input data. A fine-tuning in the networks can provide further enhancement of the performance.
更多查看译文
关键词
Post operative obesity patient,Named entity recognition,Conditional random fields,bi LSTM,CGAN,Imbalanced data,Oversampling
AI 理解论文
溯源树
样例
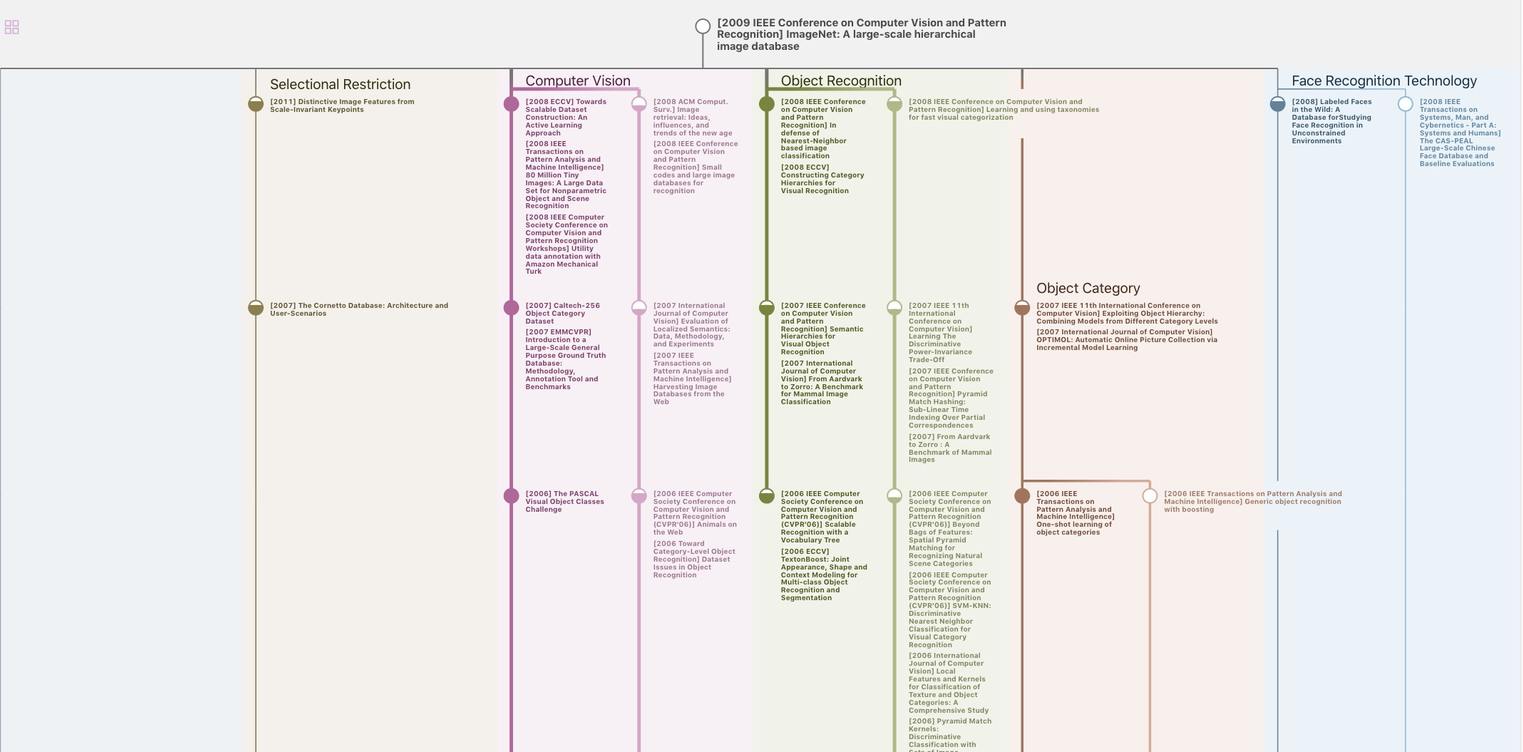
生成溯源树,研究论文发展脉络
Chat Paper
正在生成论文摘要