Low-Rank Matrix Learning Using Biconvex Surrogate Minimization.
IEEE transactions on neural networks and learning systems(2019)
摘要
Many machine learning problems involve learning a low-rank positive semidefinite matrix. However, existing solvers for this low-rank semidefinite program (SDP) are often expensive. In this paper, by factorizing the target matrix as a product of two matrices and using a Courant penalty to penalize for their difference, we reformulate the SDP as a biconvex optimization problem. This allows the use of multiconvex optimization techniques to define simple surrogates, which can be minimized easily by block coordinate descent. Moreover, while traditionally this biconvex problem approaches the original problem only when the penalty parameter is infinite, we show that the two problems are equivalent when the penalty parameter is sufficiently large. Experiments on a number of SDP applications in machine learning show that the proposed algorithm is as accurate as other state-of-the-art algorithms, but is much faster, especially on large data sets.
更多查看译文
关键词
Optimization,Machine learning,Kernel,Clustering algorithms,Machine learning algorithms,Convergence,Learning systems
AI 理解论文
溯源树
样例
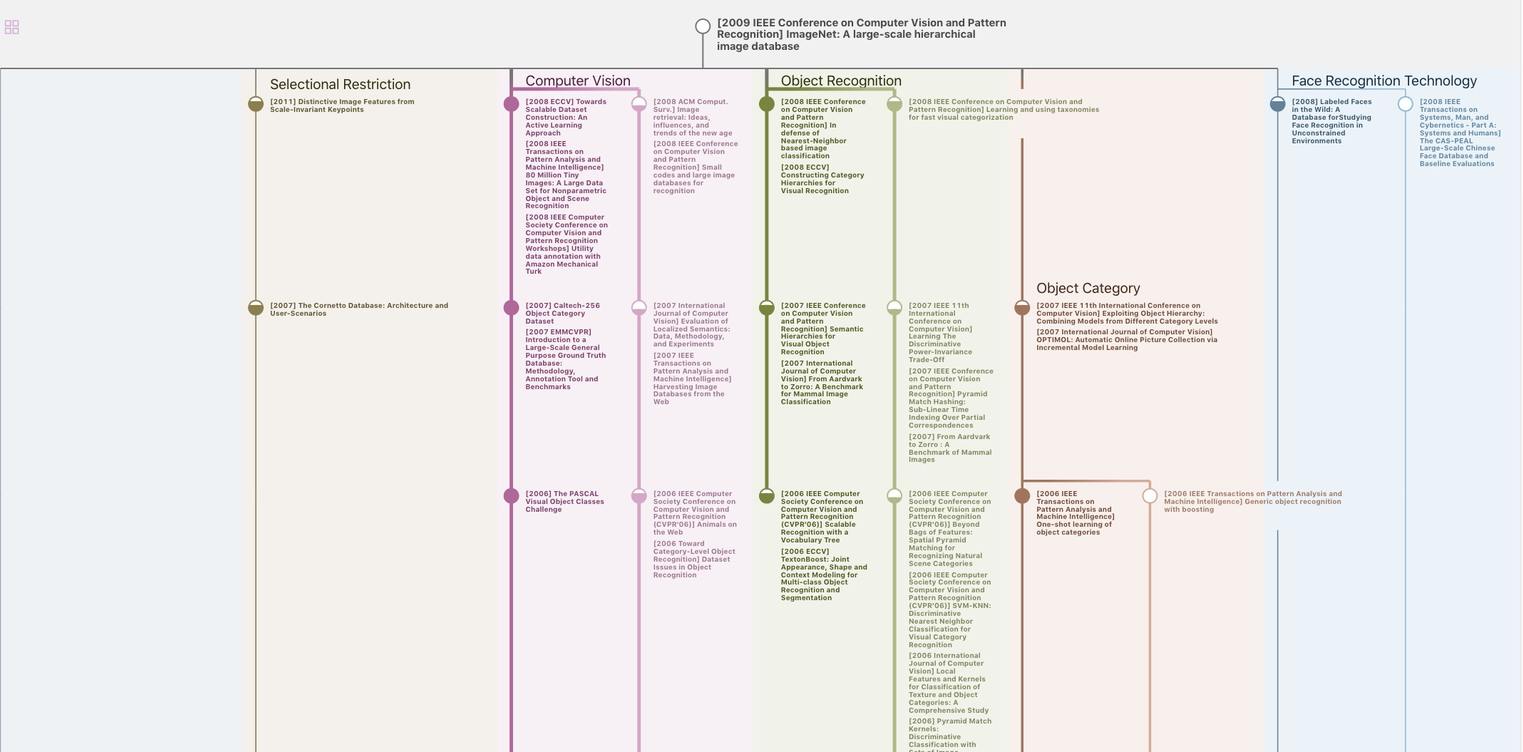
生成溯源树,研究论文发展脉络
Chat Paper
正在生成论文摘要