Manifold Alignment and Distribution Adaptation for Unsupervised Domain Adaptation
2019 IEEE International Conference on Multimedia and Expo (ICME)(2019)
摘要
Unsupervised domain adaptation is a problem which exploits the knowledge learned from the resource-rich domain to obtain an accurate classifier for the resource-poor domain. Most of the existing methods lift performance by reducing the differences between distributions, such as the difference between marginal probability distributions, the difference between conditional probability distributions, or both. However, all these methods consider the two distributions to be equally important, which could lead to poor classification performance in practical applications. Therefore, a balanced factor is required to weigh the two distributions to compensate for the degraded performance. In this paper, we first introduce this balance factor to weigh the distribution importance. On this base, we utilize the marginal distribution, introduce the ideas of manifold regularization, and then preserve the neighboring structures of the data sets, with the dimension reduction as much as possible. By this way, we propose the manifold alignment and balanced distribution adaptation algorithm. A large number of experiments have also been conducted, showing that our algorithm behaves much better than the previous ones.
更多查看译文
关键词
Transfer learning,Unsupervised domain adaptation,Distribution adaptation,Manifold alignment
AI 理解论文
溯源树
样例
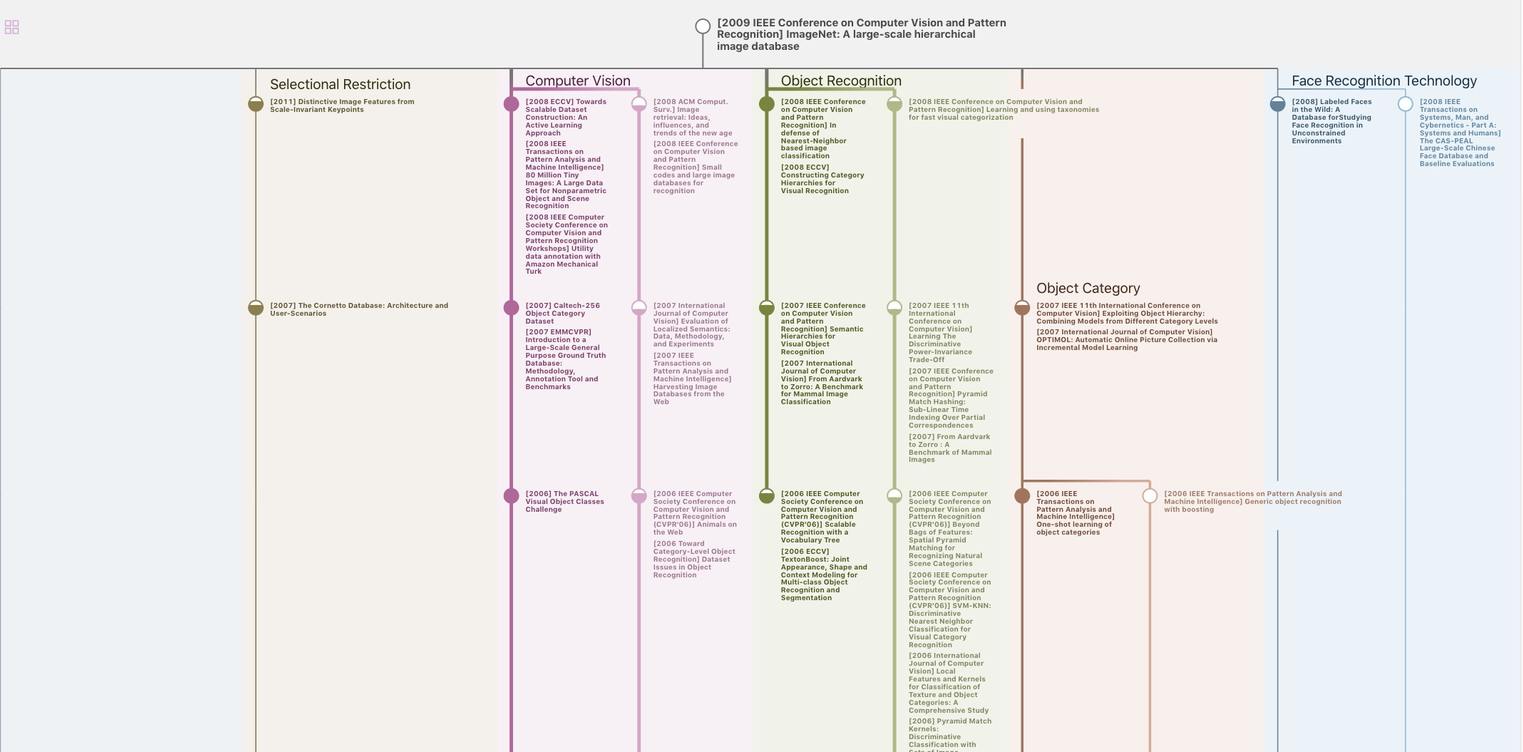
生成溯源树,研究论文发展脉络
Chat Paper
正在生成论文摘要