Stochastic Video Generation With Disentangled Representations
2019 IEEE INTERNATIONAL CONFERENCE ON MULTIMEDIA AND EXPO (ICME)(2019)
摘要
Frame-to-frame uncertainty is a major challenge in video prediction. The use of the deterministic models always leads to averaging of future states. Some methods draw samples from a prior at each time step to deal with the uncertainty of the future states, such as the SVG model [1]. However, these models always use only one set of latent variables to represent the whole stochastic part in a video clip whereas sequential data often involves multiple independent factors. In this paper, we exploit the complex representation of information in video sequences by formulating it explicitly with a disentangled-representation stochastic video generation (DR-SVG) model that imposes sequence-dependent prior and sequence-independent prior to different sets of latent variables. Through a variational lower-bound and adversarial objective functions in latent space, our model can produce crisper frames with clear content and pose which indicate the sequence-dependent and sequence-independent component respectively.
更多查看译文
关键词
stochastic video prediction, disentangled-representation, variational inference, adversarial learning
AI 理解论文
溯源树
样例
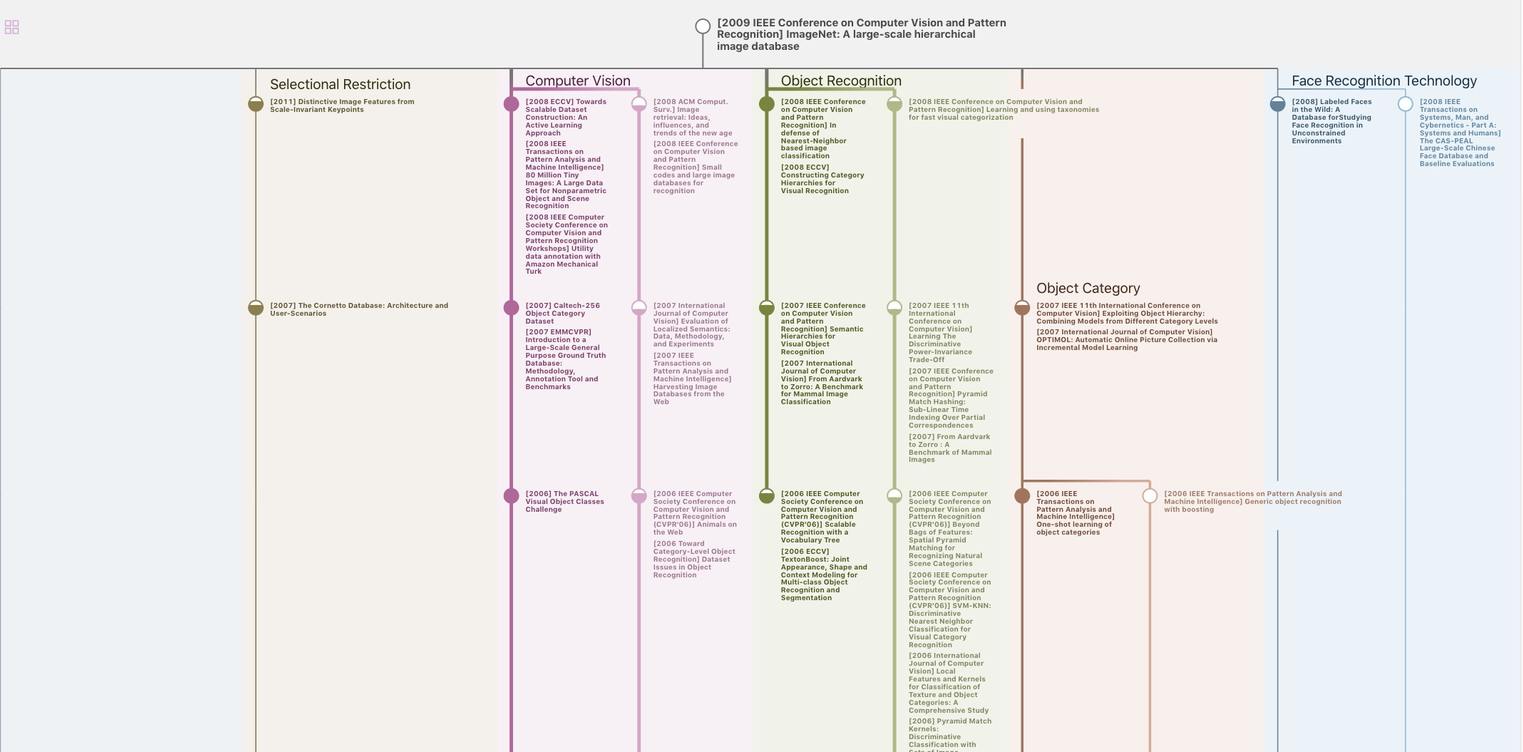
生成溯源树,研究论文发展脉络
Chat Paper
正在生成论文摘要