Improving Captioning for Low-Resource Languages by Cycle Consistency
2019 IEEE INTERNATIONAL CONFERENCE ON MULTIMEDIA AND EXPO (ICME)(2019)
摘要
Improving the captioning performance on low-resource languages by leveraging English caption datasets has received increasing research interest in recent years. Existing works mainly fall into two categories: translation-based and alignment-based approaches. In this paper, we propose to combine the merits of both approaches in one unified architecture. Specifically, we use a pre-trained English caption model to generate high-quality English captions, and then take both the image and generated English captions to generate low-resource language captions. We improve the captioning performance by adding the cycle consistency constraint on the cycle of image regions, English words, and low-resource language words. Moreover, our architecture has a flexible design which enables it to benefit from large monolingual English caption datasets. Experimental results demonstrate that our approach outperforms the state-of-the-art methods on common evaluation metrics. The attention visualization also shows that the proposed approach really improves the fine-grained alignment between words and image regions.
更多查看译文
关键词
image captioning, low-resource language, cycle consistency, fine-grained alignment
AI 理解论文
溯源树
样例
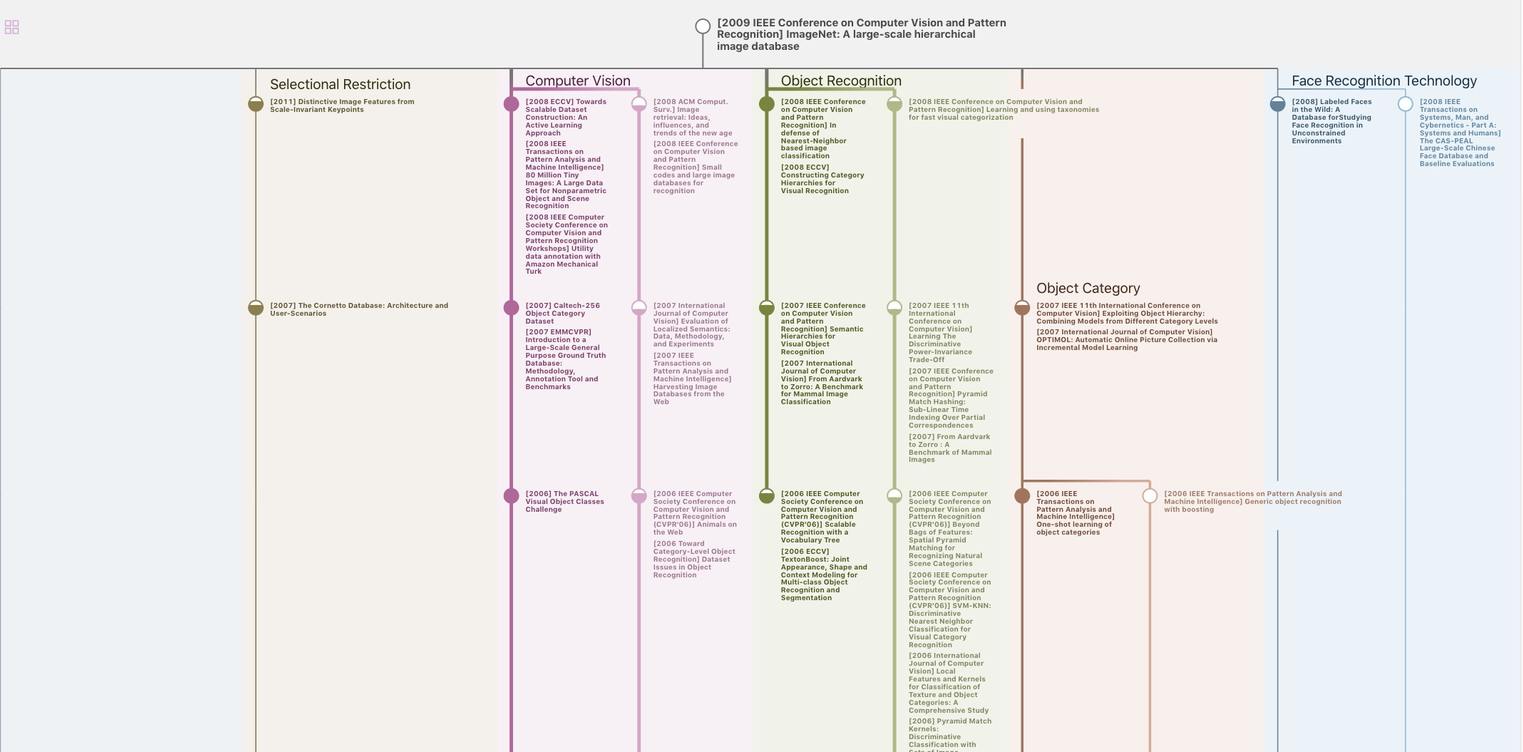
生成溯源树,研究论文发展脉络
Chat Paper
正在生成论文摘要