Learning To Segment Unseen Category Objects Using Gradient Gaussian Attention
2019 IEEE INTERNATIONAL CONFERENCE ON MULTIMEDIA AND EXPO (ICME)(2019)
摘要
Existing semantic segmentation models are trapped in the categories of training sets. Unfortunately, public datasets provide pixel-level annotations only for a small quantity of images and few categories. In this paper, we propose a novel cross-category supervised object segmentation network to explore the similar features among different categories, which can transfer the learned segmentation knowledge from categories with mask annotations to unseen categories that only have bounding boxes. Specifically, we fuse gaussian attention map of an object with guided gradient back-propagation map as an extra input, which gives localizable and discriminative prior cues to obtain precise object segmentation from the bounding box. In addition to computing a segment for each box, we also fuse segments to generate pixel-level labels. Then, without modifying the segmentation training program, the generated labels are still sufficient and achieve about 98.2% of the fully supervised model, in the case of only 50% categories with pixel-level annotations on PASCAL VOC 2012. The proposed method is also effective for interactive segmentation and salient object detection.
更多查看译文
关键词
Cross-category supervised, object segmentation, knowledge transfer, gradient gaussian attention
AI 理解论文
溯源树
样例
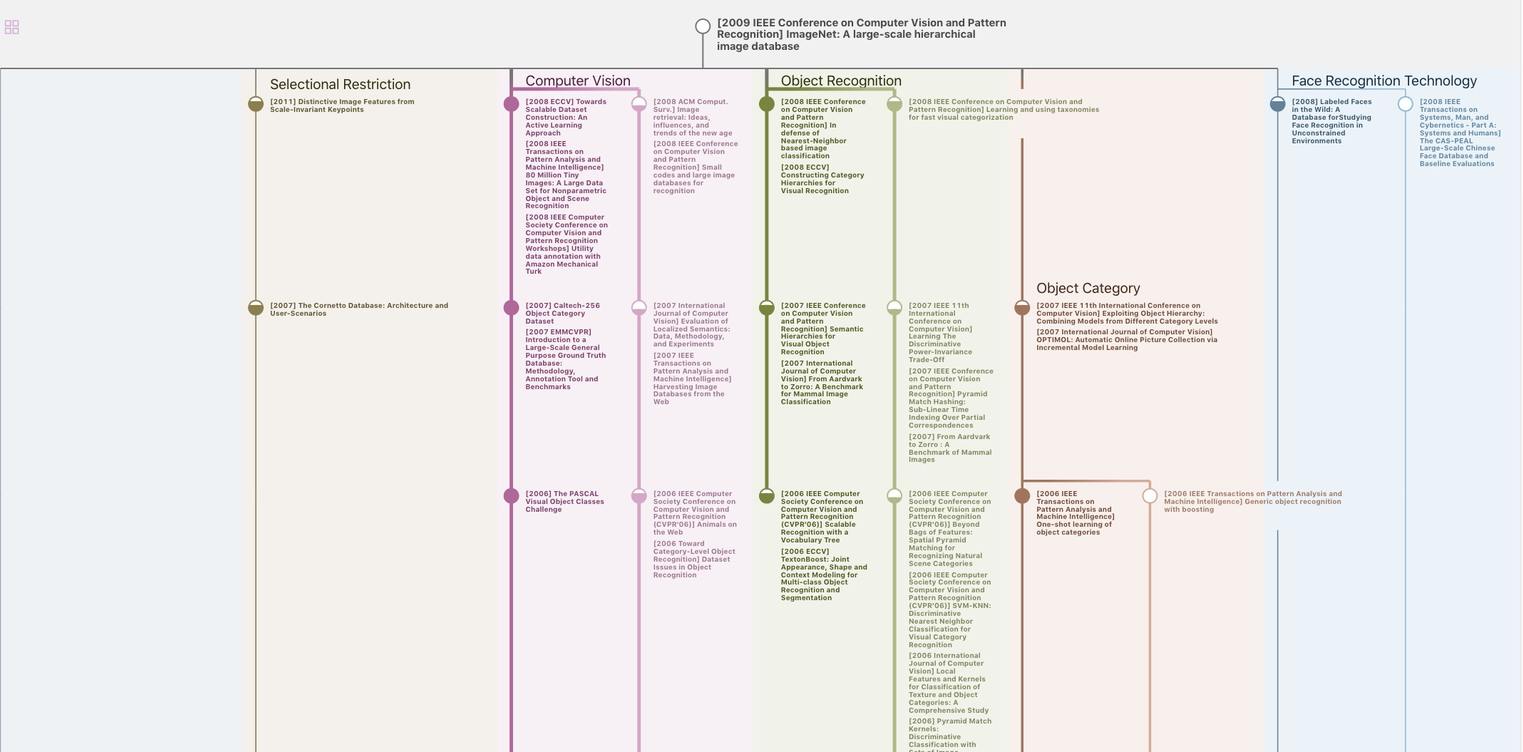
生成溯源树,研究论文发展脉络
Chat Paper
正在生成论文摘要