Mask Guided Knowledge Distillation For Single Shot Detector
2019 IEEE INTERNATIONAL CONFERENCE ON MULTIMEDIA AND EXPO (ICME)(2019)
摘要
In this paper, we explore the idea of distilling small networks for object detection task. More specifically, we propose a two-stage approach to learn more compact and efficient detectors under the single-shot object detection framework by leveraging knowledge distillation. During the 1st stage, we learn the feature maps of the student model for each of the prediction head from the teacher model. Instead of fitting the whole feature map directly, here we propose the mask guided structure including not only the entire feature map (i.e. global features) but also region features covered by the object (i.e. local features), which can significantly improve the performance of the student network. For the 2nd stage, the ground-truth is used to further refine the performance. Experimental results on PASCAL VOC and KITTI dataset demonstrate the effectiveness of our proposed approach. We achieve 56.88% mAP on VOC2007 at 143 FPS with the backbone of 1/8 VGG16.
更多查看译文
关键词
Object detection, knowledge distillation
AI 理解论文
溯源树
样例
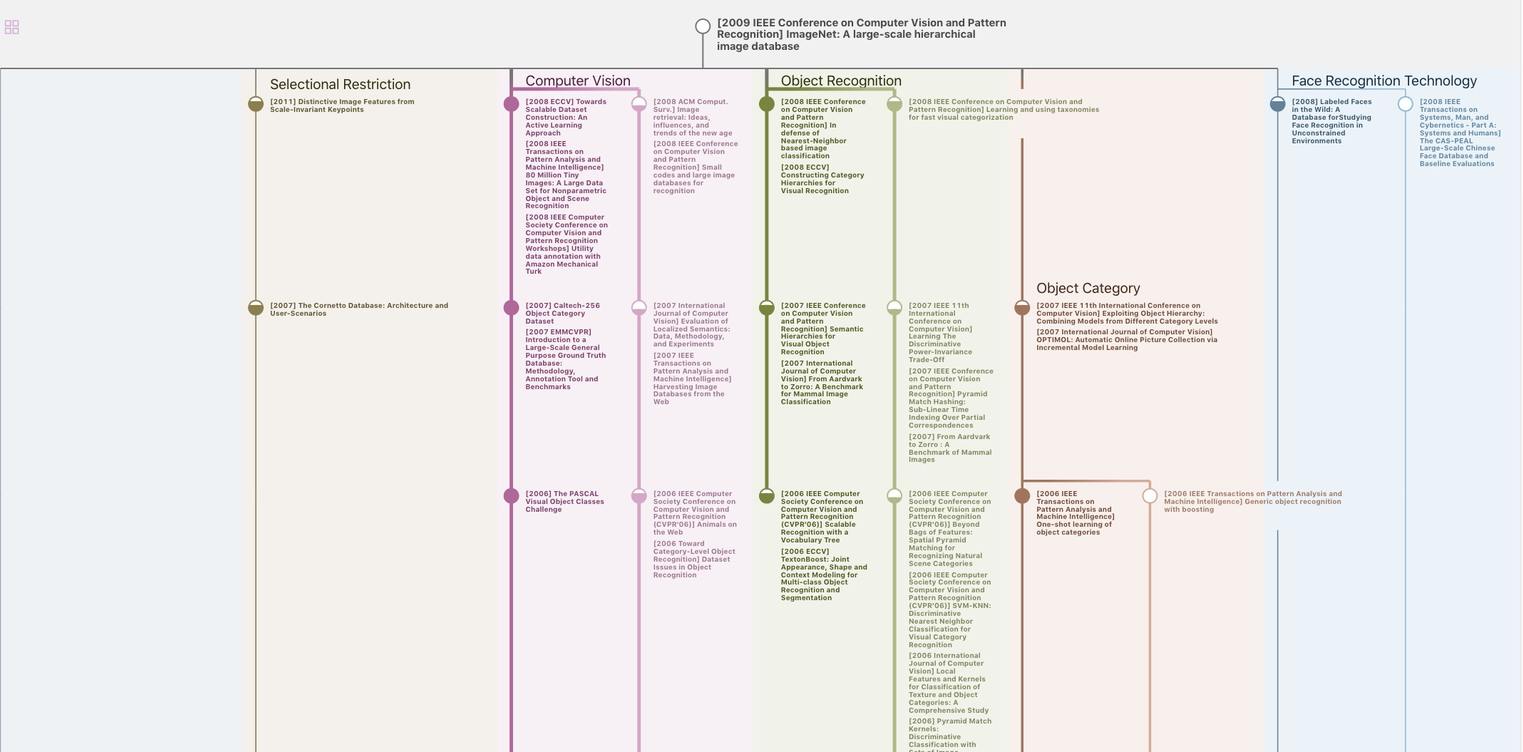
生成溯源树,研究论文发展脉络
Chat Paper
正在生成论文摘要