Inferring the Synaptical Weights of Leaky Integrate and Fire Asynchronous Neural Networks - Modelled as Timed Automata.
BIOMEDICAL ENGINEERING SYSTEMS AND TECHNOLOGIES, BIOSTEC 2018(2019)
摘要
In this work we introduce a new approach to learn the synaptical weights of neural biological networks. At this aim, we consider networks of Leaky Integrate and Fire neurons and model them as timed automata networks. Each neuron receives input spikes through its incoming synapses (modelled as channels) and computes its membrane potential value according to the (present and past) received inputs. Whenever the potential value overtakes a given firing threshold, the neuron emits a spike (modelled as a broadcast signal over the output channel of the corresponding automaton). After each spike emission, the neuron enters first an absolute refractory period, in which signal emission is not allowed, and then a relative refractory period, in which the firing threshold is higher than usual. Neural networks are modelled as sets of timed automata running in parallel and sharing channels in compliance with the network structure. Such a formal encoding allows us to propose an algorithm which automatically infers the synaptical weights of neural networks such that a given dynamical behaviour can be displayed. Behaviours are encoded as temporal logic formulae and the algorithm modifies the network weights until un assignment satisfying the specification is found.
更多查看译文
关键词
Neural networks,Parameter learning,Timed automata,Temporal logic,Model checking
AI 理解论文
溯源树
样例
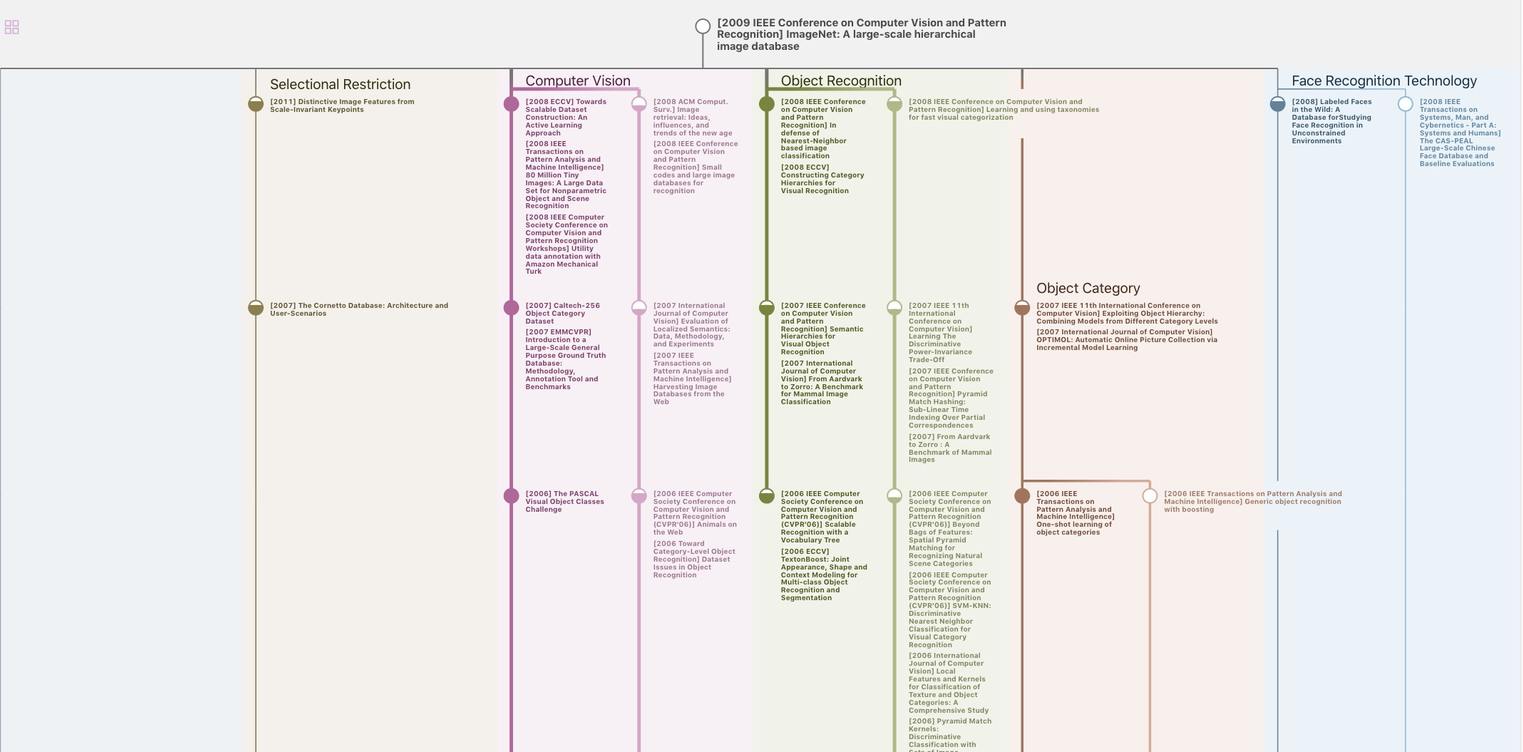
生成溯源树,研究论文发展脉络
Chat Paper
正在生成论文摘要