Evolutionary Design of Recurrent Neural Network Architecture for Human Activity Recognition
2019 IEEE Congress on Evolutionary Computation (CEC)(2019)
摘要
Recurrent Neural Networks (RNNs) are a major class of artificial neural networks and one of the most effective deep learning models for human activity recognition (HAR). However designing the architecture of an RNN together with suitable hyper-parameters for any learning task can be very time-consuming and requires expert domain knowledge. This paper focuses on exploring a Genetic Algorithm (GA) based method to automatically design suitable architectures of Long Short Term Memory (LSTM)-based RNNs in a fully automated manner. An encoding strategy is proposed to encode the architectures and hyper-parameters of LSTM, for the sake of easy operation by GA. As a variant of RNN, LSTM is selected for this work due to its special feature to handle long-term dependencies. For verification and evaluation, three real-world benchmark datasets are used. We study three models of operation for the evolved deep LSTM-RNN, i.e., unidirectional, bidirectional and cascaded. The results of our experiments show that the RNN architectures automatically designed by our GA method can outperform state-of-the-art RNN systems and machine learning systems for HAR.
更多查看译文
关键词
Recurrent Neural Networks,Genetic Algorithm,Long Short Term Memory,Human Activity Recognition
AI 理解论文
溯源树
样例
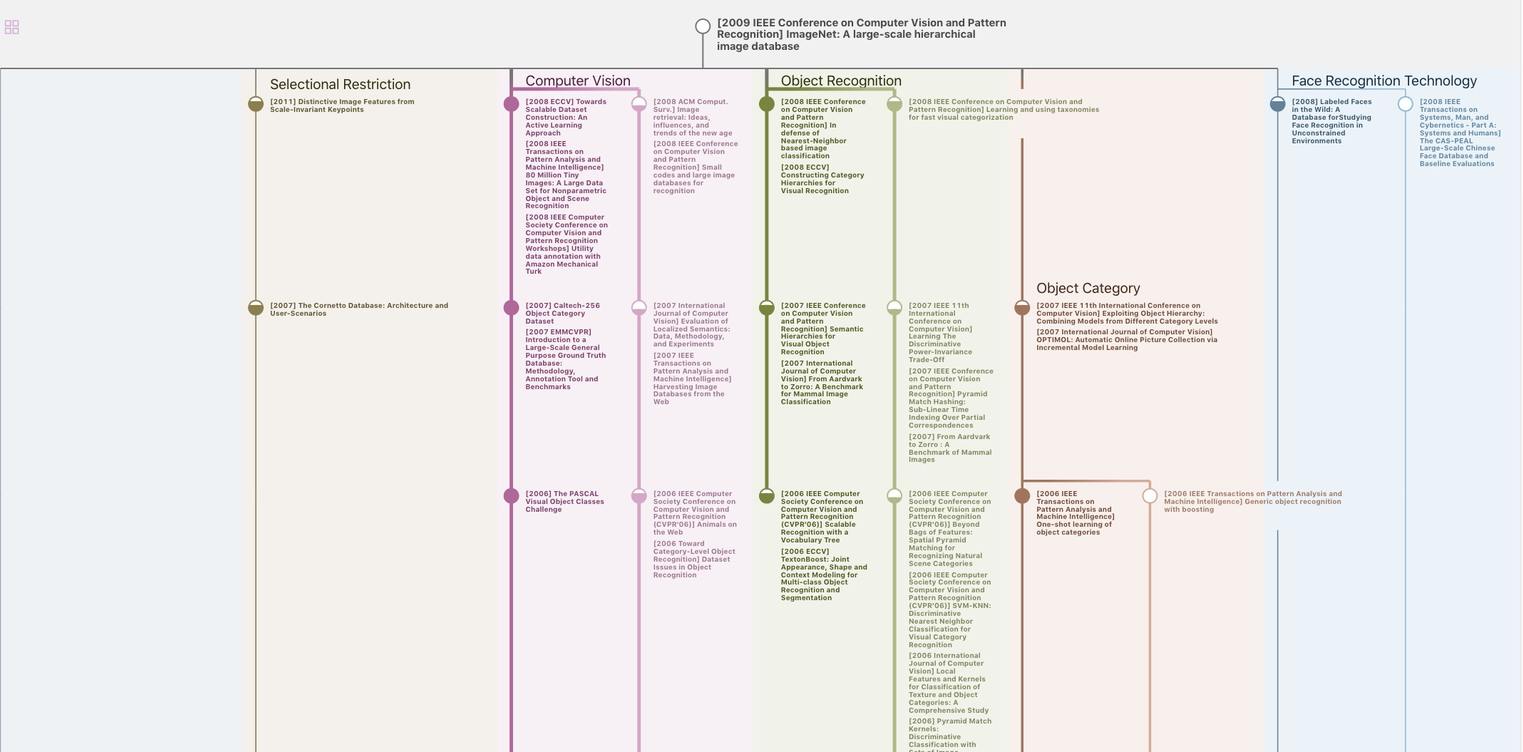
生成溯源树,研究论文发展脉络
Chat Paper
正在生成论文摘要