Deep Multi-agent Reinforcement Learning in a Common-Pool Resource System
2019 IEEE Congress on Evolutionary Computation (CEC)(2019)
摘要
In complex social-ecological systems, multiple agents with diverse objectives take actions that affect the long-term dynamics of the system. Common pool resources are a subset of such systems, where property rights are typically poorly defined and dynamics are unknown a priori, creating a social dilemma reflected by the well-known `tragedy of the commons.' In this paper, we investigated the efficacy of deep reinforcement learning in a multi-agent setting of a common pool resource system. We used an abstract mathematical model of the system, represented as a partially-observable general-sum Markov game. In the first set of experiments, the independent agents used a deep Q-Network with discrete action spaces to guide decision-making. However, significant shortcomings were evident. Consequently, in a second set of experiments, a Deep Deterministic Policy Gradient learning model with continuous state and action spaces guided agent learning. Simulation results show that agents performed significantly better in terms of both sustainability and economic goals when using the second deep learning model. Despite the fact that agents do not have perfect foresight nor understanding of the implications of their `harvesting' efforts, deep reinforcement learning can be used effectively to `learn in the commons'.
更多查看译文
关键词
deep Q-Network,deep learning model,common-pool resource system,social-ecological systems,long-term dynamics,deep multiagent reinforcement learning,deep deterministic policy gradient learning model,partially-observable general-sum Markov game
AI 理解论文
溯源树
样例
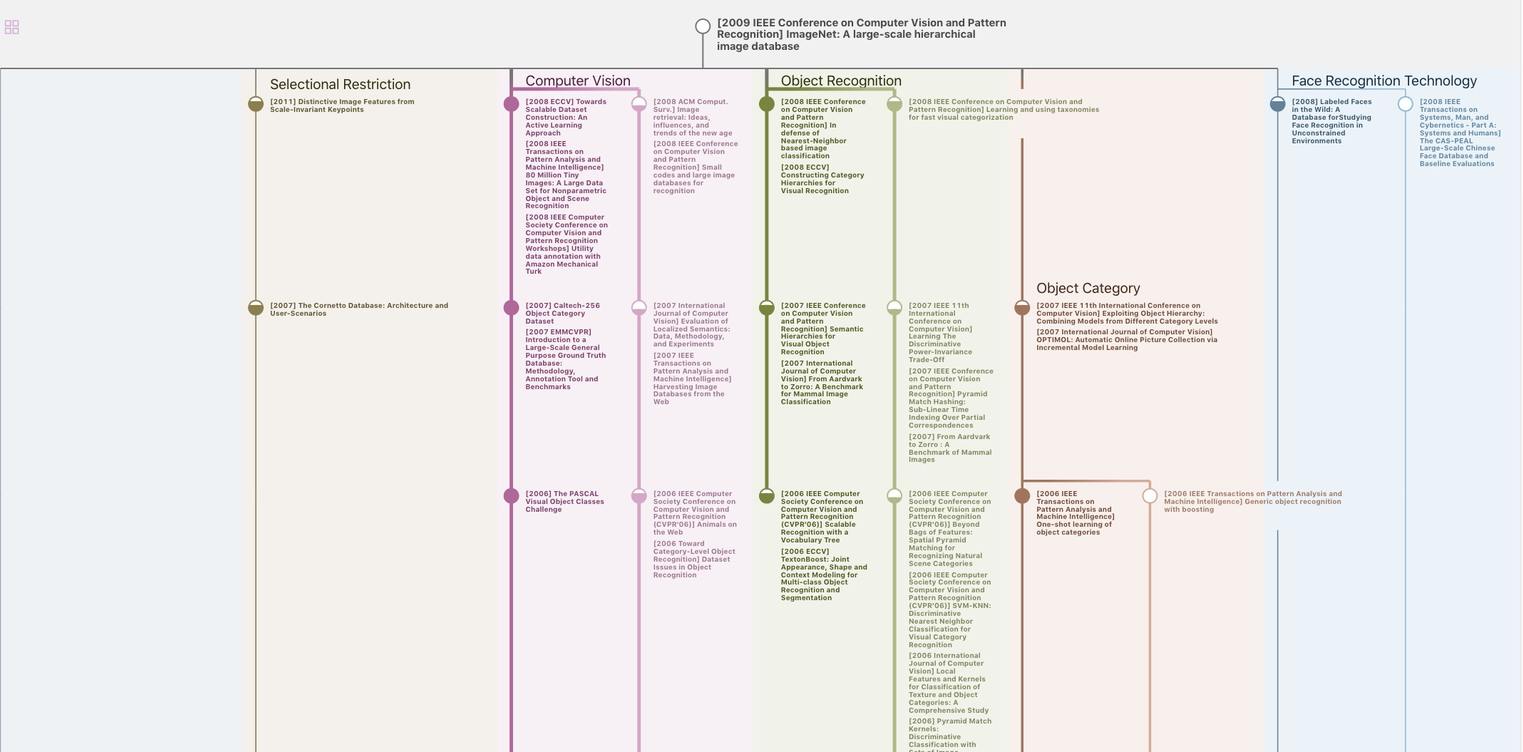
生成溯源树,研究论文发展脉络
Chat Paper
正在生成论文摘要