Deep Multi-Sensory Object Category Recognition Using Interactive Behavioral Exploration
2019 INTERNATIONAL CONFERENCE ON ROBOTICS AND AUTOMATION (ICRA)(2019)
摘要
When identifying an object and its properties, humans use features from multiple sensory modalities produced when manipulating the object. Motivated by this cognitive process, we propose a deep learning methodology for object category recognition which uses visual, auditory, and haptic sensory data coupled with exploratory behaviors (e.g., grasping, lifting, pushing, etc.). In our method, as the robot performs an action on an object, it uses a Tensor-Train Gated Recurrent Unit network to process its visual data, and Convolutional Neural Networks to process haptic and auditory data. We propose a novel strategy to train a single neural network that inputs video, audio and haptic data, and demonstrate that its performance is better than separate neural networks for each sensory modality. The proposed method was evaluated on a dataset in which the robot explored 100 different objects, each belonging to one of 20 categories. While the visual information was the dominant modality for most categories, adding the additional haptic and auditory networks further improves the robot's category recognition accuracy. For some of the behaviors, our approach outperforms the previous published baseline for the dataset which used handcrafted features for each modality. We also show that a robot does not need the sensory data from the entire interaction, but instead can make a good prediction early on during behavior execution.
更多查看译文
关键词
multisensory object category recognition,interactive behavioral exploration,deep learning methodology,visual data,haptic sensory data,haptic data,auditory data,sensory modality,visual information,dominant modality,auditory networks,convolutional neural networks,haptic networks,tensor-train gated recurrent unit network,robot category recognition
AI 理解论文
溯源树
样例
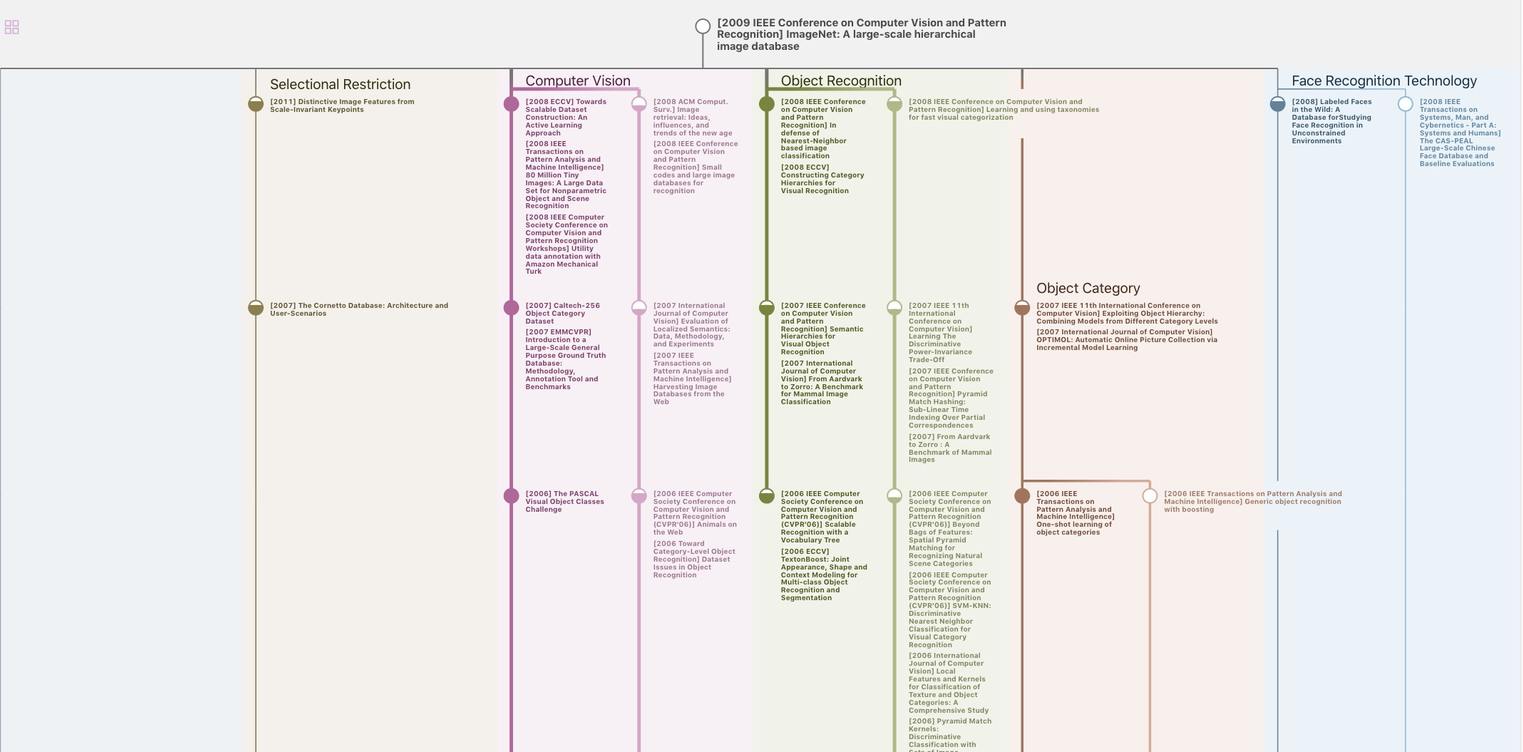
生成溯源树,研究论文发展脉络
Chat Paper
正在生成论文摘要