Individualized treatment response prediction of dialectical behavior therapy for borderline personality disorder using multimodal magnetic resonance imaging.
BRAIN AND BEHAVIOR(2019)
摘要
Introduction Individualized treatment prediction is crucial for the development and selection of personalized psychiatric interventions. Here, we use random forest classification via pretreatment clinical and demographical (CD), functional, and structural magnetic resonance imaging (MRI) data from patients with borderline personality disorder (BPD) to predict individual treatment response. Methods Before dialectical behavior therapy (DBT), 31 female patients underwent functional (three different emotion regulation tasks) and structural MRI. DBT response was predicted using CD and MRI data in previously identified anatomical regions, which have been reported to be multimodally affected in BPD. Results Amygdala and parahippocampus activation during a cognitive reappraisal task (in contrasts displaying neural activation for emotional challenge and for regulation), along with severity measures of BPD psychopathology and gray matter volume of the amygdala, provided best predictive power with neuronal hyperractivities in nonresponders. All models, except one model using CD data solely, achieved significantly better accuracy (>70.25%) than a simple all-respond model, with sensitivity and specificity of >0.7 and >0.7, as well as positive and negative likelihood ratios of >2.74 and <0.36 each. Surprisingly, a model combining all data modalities only reached rank five of seven. Among the functional tasks, only the activation elicited by a cognitive reappraisal paradigm yielded sufficient predictive power to enter the final models. Conclusion This proof of principle study shows that it is possible to achieve good predictions of psychotherapy outcome to find the most valid predictors among numerous variables via using a random forest classification approach.
更多查看译文
关键词
functional MRI,machine learning,multimodal data analysis,prediction of treatment response,random forest,structural MRI
AI 理解论文
溯源树
样例
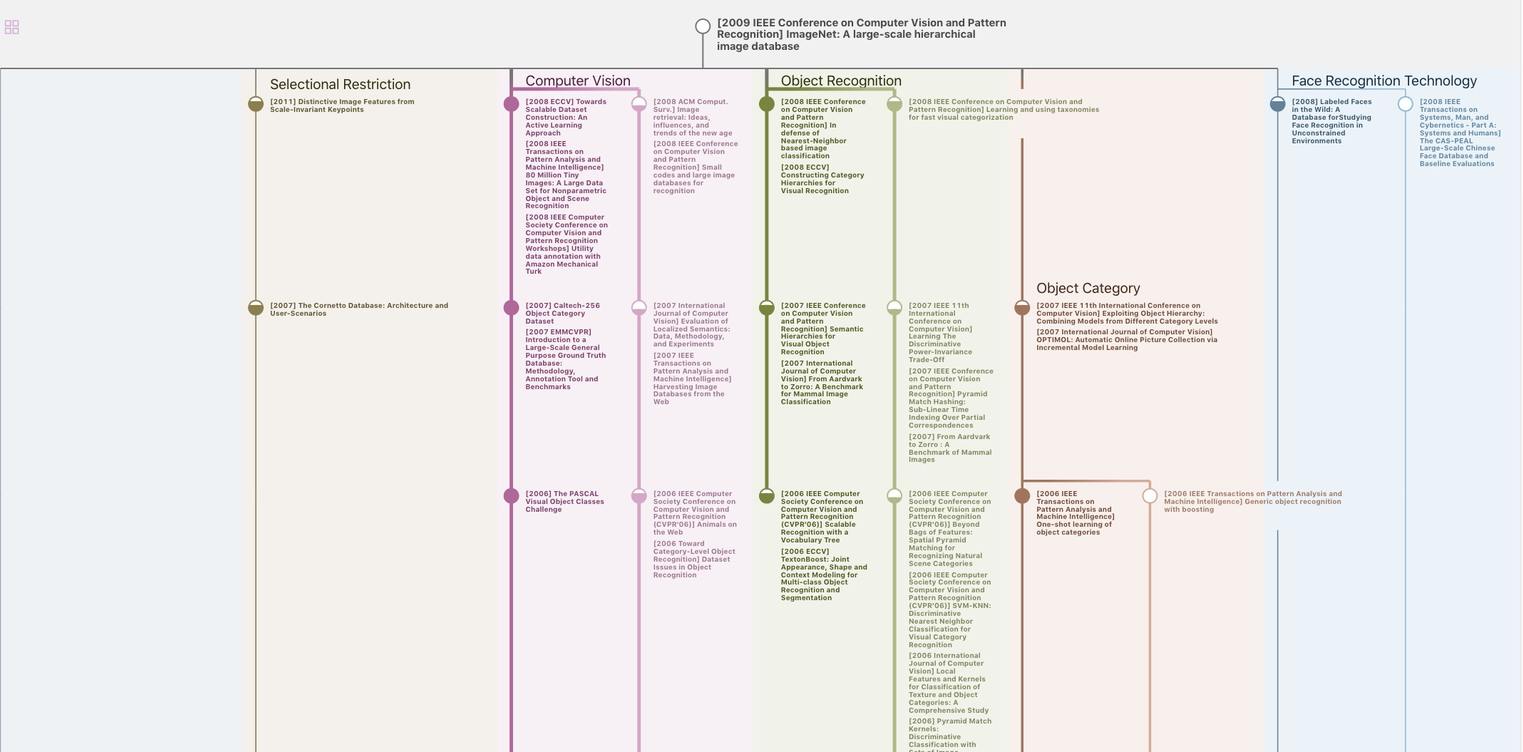
生成溯源树,研究论文发展脉络
Chat Paper
正在生成论文摘要