Automatic Compiler Based FPGA Accelerator for CNN Training
2019 29th International Conference on Field Programmable Logic and Applications (FPL)(2019)
摘要
Training of convolutional neural networks (CNNs) on embedded platforms to support on-device learning is earning vital importance in recent days. Designing flexible training hardware is much more challenging than inference hardware, due to design complexity and large computation/memory requirement. In this work, we present an automatic compiler based FPGA accelerator with 16-bit fixed-point precision for complete CNN training, including Forward Pass (FP), Backward Pass (BP) and Weight Update (WU). We implemented an optimized RTL library to perform training-specific tasks and developed an RTL compiler to automatically generate FPGA-synthesizable RTL based on user-defined constraints. We present a new cyclic weight storage/access scheme for on-chip BRAM and off-chip DRAM to efficiently implement non-transpose and transpose operations during FP and BP phases, respectively. Representative CNNs for CIFAR-10 dataset are implemented and trained on Intel Stratix 10 GX FPGA using proposed hardware architecture, demonstrating up to 479 GOPS performance.
更多查看译文
关键词
Convolution neural networks, neural network training, back-propagation, hardware accelerator, FPGA
AI 理解论文
溯源树
样例
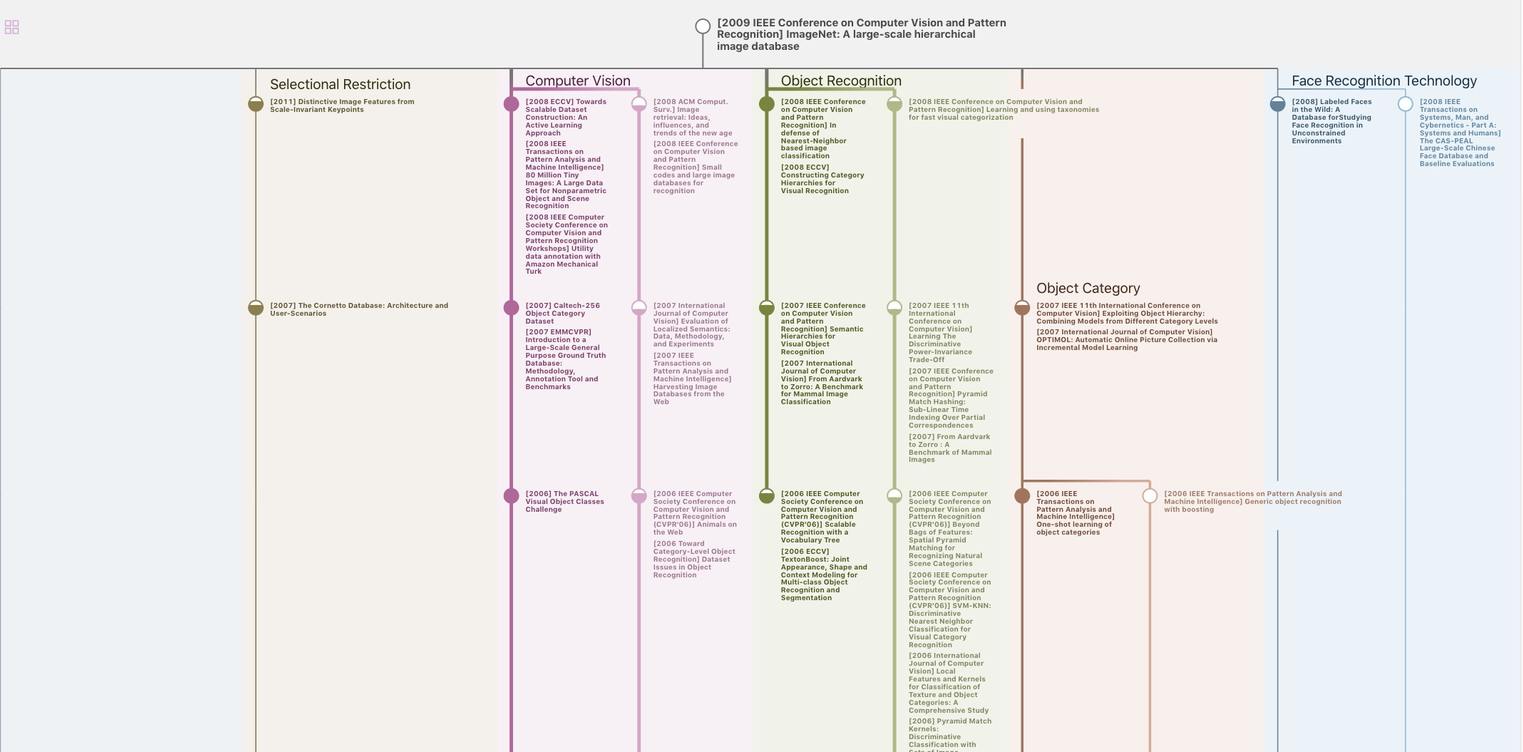
生成溯源树,研究论文发展脉络
Chat Paper
正在生成论文摘要