Efficient -anonymous microaggregation of multivariate numerical data via principal component analysis.
Information Sciences(2019)
摘要
•The primary goal of this work is to reduce the running time of k-anonymous microaggregation algo-rithms operating on datasets with a large quantity of numerical demographic attributes, acting as quasi-identifiers. Principal component analysis (PCA), an algebraic-statistical procedure that constructs an or-thogonal projection onto a lower-dimensional subspace, permits the effective reduction of the number of attributes of the original dataset. The optimality principles of multivariate PCA strive to preserve Euclidean distances between the projected data points.•The compressed data is fed to the microaggregation algorithm, but the k-anonymous microcells or groups obtained are directly applied to the original data. The distance-preservation properties of multivariate PCA help construct a micropartition of the set of respondents similar to that obtained when the original data is microaggregated in the conventional fashion, but in fewer dimensions.•This means that we are able to achieve significant time gains ( ≈ 14–31%) with very little impact on information utility ( < 2%, with respect to the total variance) with respect to the traditional procedure on the original data.•Additional variants of the above method are devised and analyzed with extensive experimentation on standardized datasets, in terms of running time and information loss, pushing the already substantial speed-up even further ( ≈ 48–64%), with mild distortion impact ( < 3%, with respect to the total variance).
更多查看译文
关键词
Data privacy,Statistical disclosure control,k-anonymity,Microaggregation,Principal component analysis,Large-scale datasets
AI 理解论文
溯源树
样例
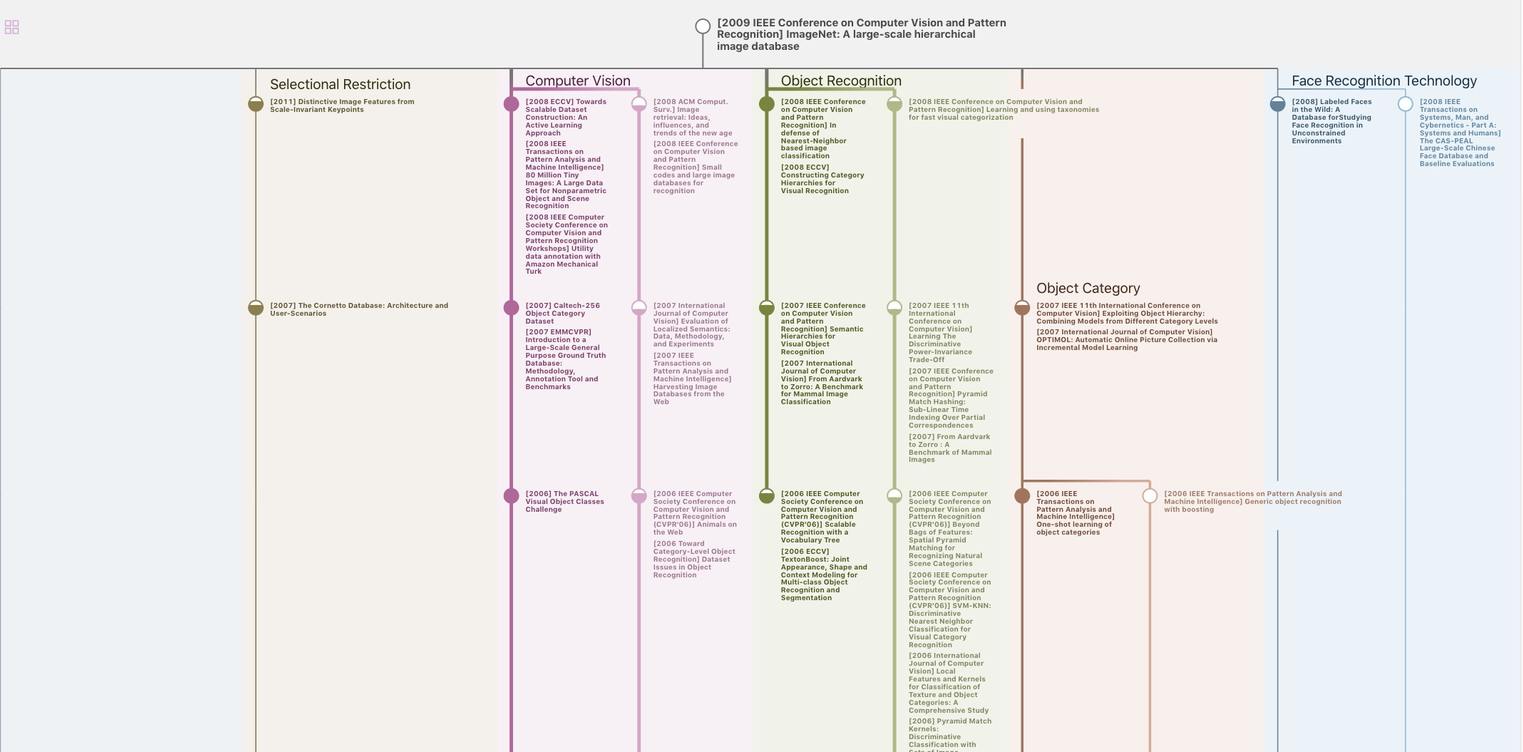
生成溯源树,研究论文发展脉络
Chat Paper
正在生成论文摘要