BGADAM: Boosting based Genetic-Evolutionary ADAM for Convolutional Neural Network Optimization
arxiv(2019)
摘要
Among various optimization algorithms, ADAM can achieve outstanding performance and has been widely used in model learning. ADAM has the advantages of fast convergence with both momentum and adaptive learning rate. For deep neural network learning problems, since their objective functions are nonconvex, ADAM can also get stuck in local optima easily. To resolve such a problem, the genetic evolutionary ADAM (GADAM) algorithm, which combines the ADAM and genetic algorithm, was introduced in recent years. To further maximize the advantages of the GADAM model, we propose to implement the boosting strategy for unit model training in GADAM. In this paper, we introduce a novel optimization algorithm, namely Boosting based GADAM (BGADAM). We will show that after adding the boosting strategy to the GADAM model, it can help unit models jump out the local optima and converge to better solutions.
更多查看译文
关键词
Training,Deep learning,Analytical models,Neural networks,Optimization methods,Boosting,Genetics
AI 理解论文
溯源树
样例
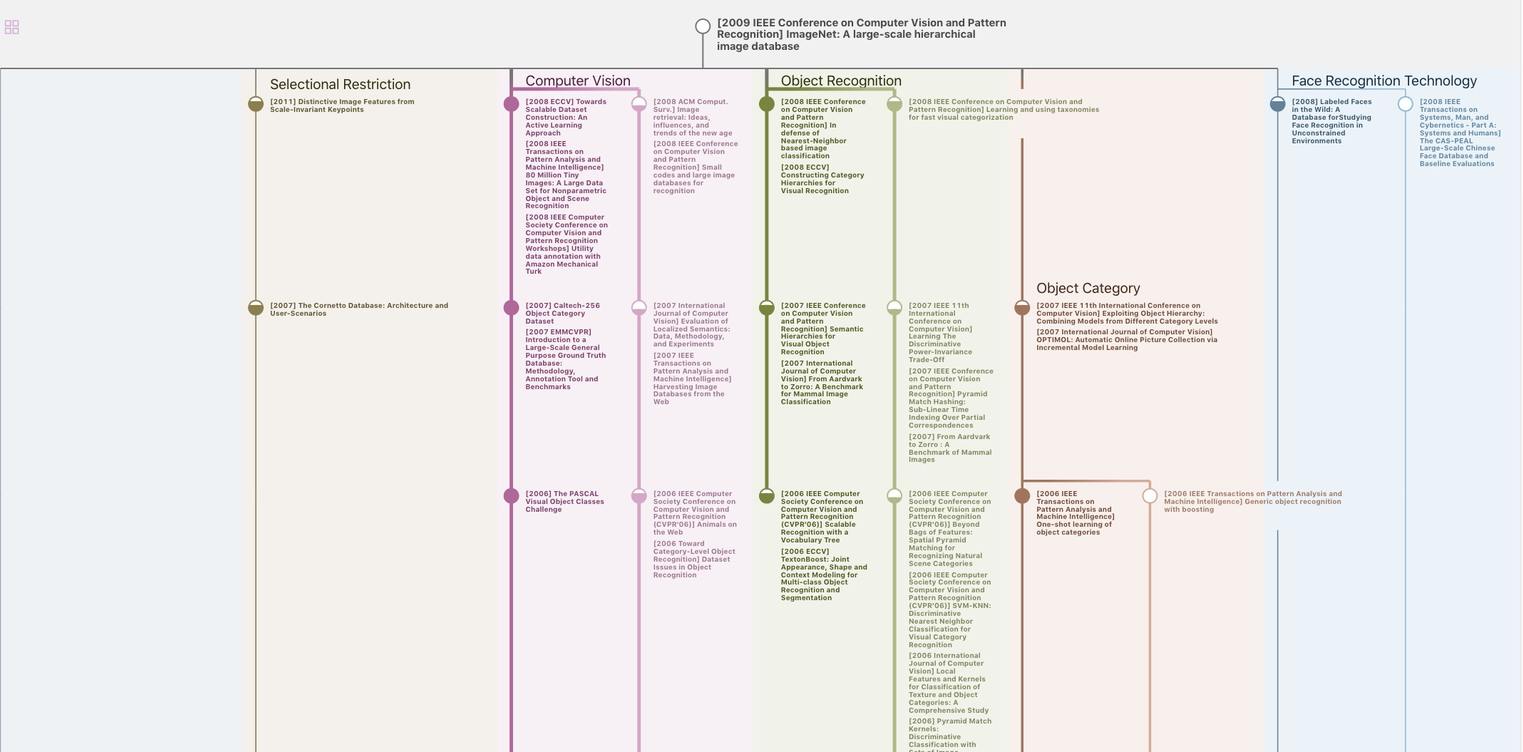
生成溯源树,研究论文发展脉络
Chat Paper
正在生成论文摘要