More Unlabelled Data or Label More Data? A Study on Semi-supervised Laparoscopic Image Segmentation
CoRR(2019)
摘要
Improving a semi-supervised image segmentation task has the option of adding more unlabelled images, labelling the unlabelled images or combining both, as neither image acquisition nor expert labelling can be considered trivial in most clinical applications. With a laparoscopic liver image segmentation application, we investigate the performance impact by altering the quantities of labelled and unlabelled training data, using a semi-supervised segmentation algorithm based on the mean teacher learning paradigm. We first report a significantly higher segmentation accuracy, compared with supervised learning. Interestingly, this comparison reveals that the training strategy adopted in the semi-supervised algorithm is also responsible for this observed improvement, in addition to the added unlabelled data. We then compare different combinations of labelled and unlabelled data set sizes for training semi-supervised segmentation networks, to provide a quantitative example of the practically useful trade-off between the two data planning strategies in this surgical guidance application.
更多查看译文
关键词
Semi-supervised,Laparoscopic video,Image segmentation
AI 理解论文
溯源树
样例
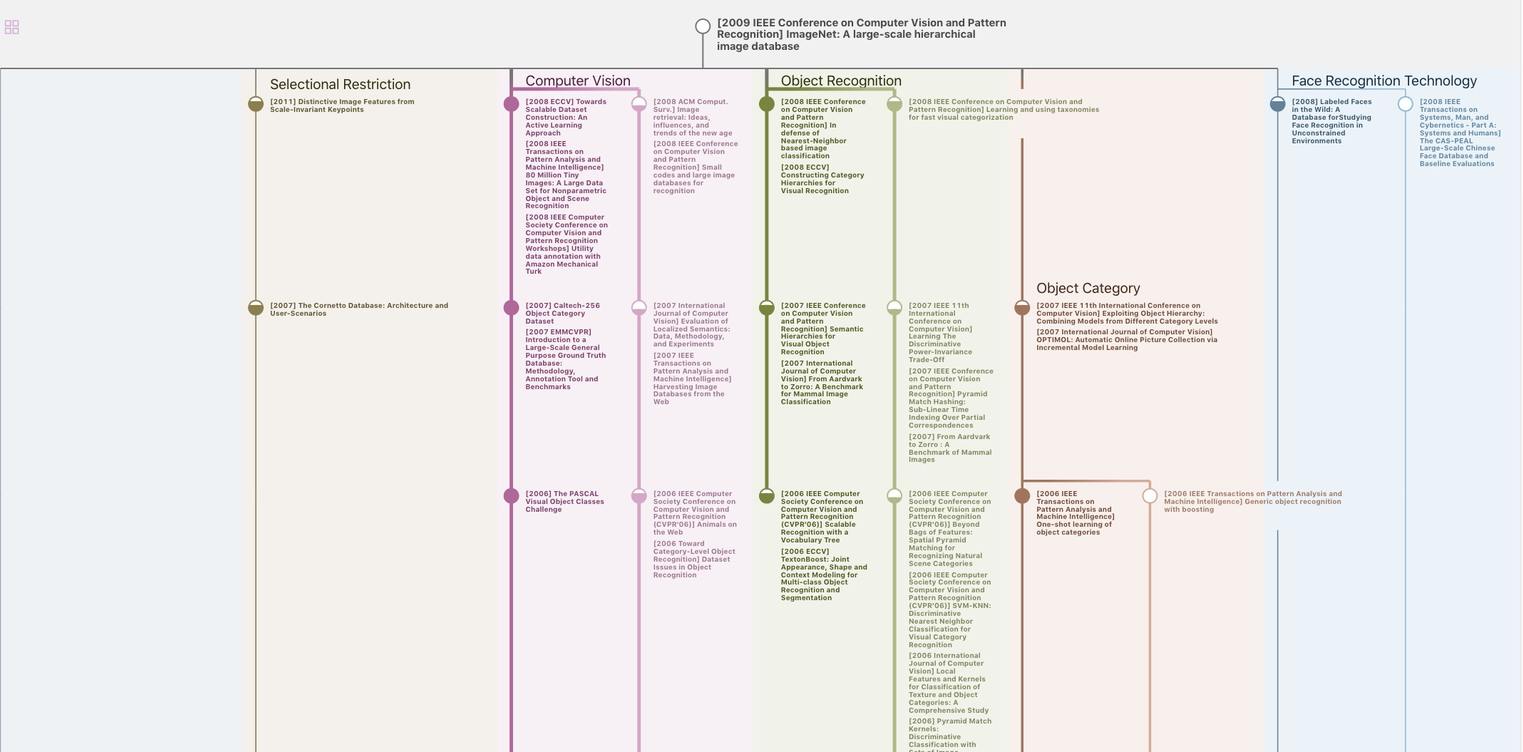
生成溯源树,研究论文发展脉络
Chat Paper
正在生成论文摘要