Automated Pipeline for Photovoltaic Module Electroluminescence Image Processing and Degradation Feature Classification
IEEE Journal of Photovoltaics(2019)
摘要
An automated data analysis pipeline is developed to preprocess electroluminescence (EL) module images, and parse the images into individual cells to be used as an input for machine learning algorithms. The dataset used in the study includes EL images of three 60 cell modules from each of five commercial brands at six steps of damp heat exposure, from 500 to 3000 h. Preprocessing of the original raw EL images includes lens distortion correction, filtering, thresholding, convex hull, regression fitting, and perspective transformation to produce planar indexed module and single cell images. Parsing of PV cells from each of the preprocessed 90 EL module images gives us 5400 cell images, which are function of module brand and damp heat exposure step. From the dataset, two unique degradation categories (“cracked” and “corroded”) were observed, while cells that did not degrade were classified as “good.” For supervised machine learning modeling, cell images were sorted into these three classes yielding 3550 images. A training and testing framework with 80:20 sampling ratio was generated using stratified sampling. Three machine learning algorithms (support vector machine, Random Forest, and convolutional neural network) were trained and tuned independently on the training set and then given the test set to predict the scores for each of the three models. Five-fold cross validation was done on training set to tune hyper-parameters of the models. Model prediction scores showed that convolutional neural network outperforms support vector machine and Random Forest for supervised PV cell classification.
更多查看译文
关键词
Computer vision,electroluminescence (EL) imaging,photovoltaic (PV) cell segmentation,prediction,supervised machine learning
AI 理解论文
溯源树
样例
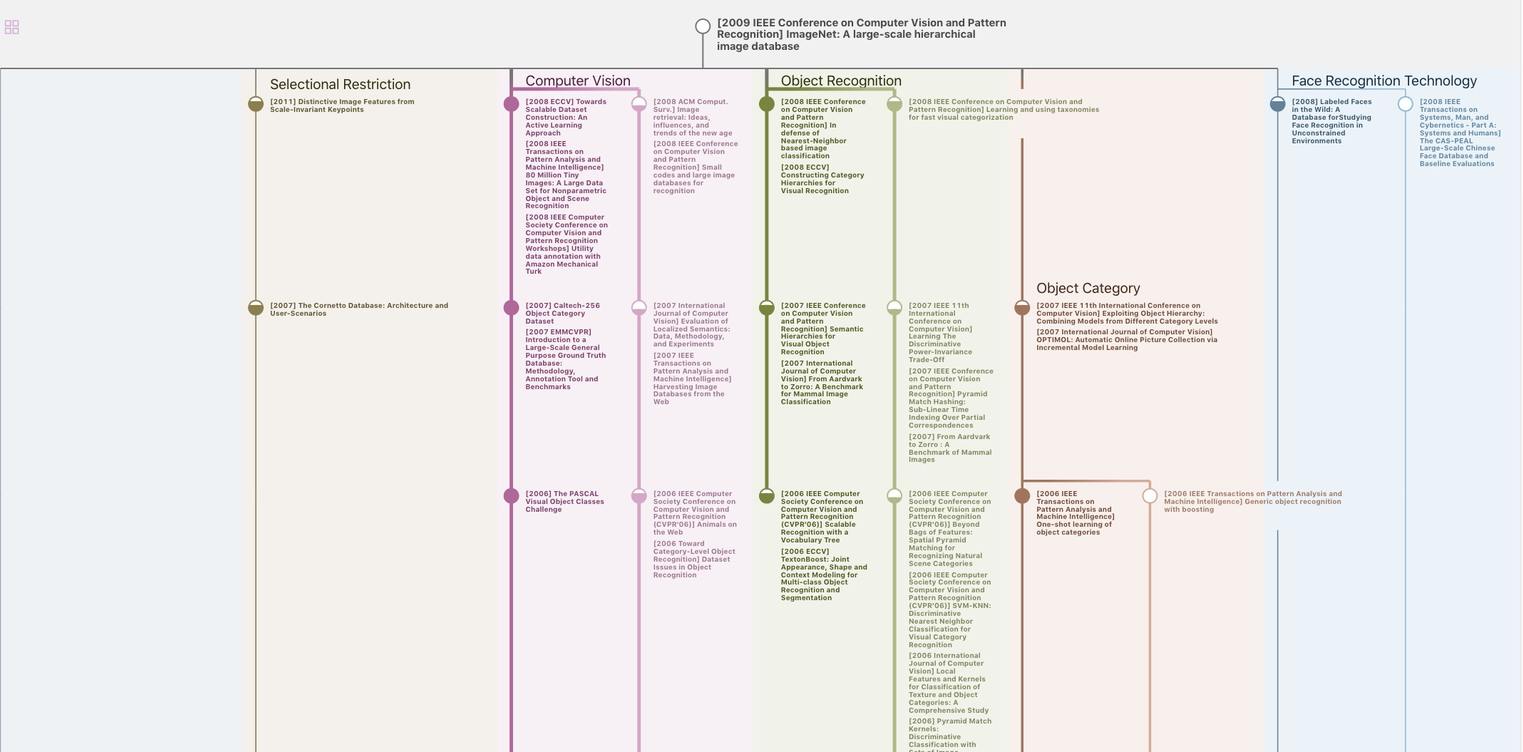
生成溯源树,研究论文发展脉络
Chat Paper
正在生成论文摘要