Spatiotemporal Sequence Memory for Prediction using Deep Sparse Coding
Proceedings of the 7th Annual Neuro-inspired Computational Elements Workshop(2019)
摘要
Our brains are, "prediction machines", where we are continuously comparing our surroundings with predictions from internal models generated by our brains. This is demonstrated by observing our basic low level sensory systems and how they predict environmental changes as we move through space and time. Indeed, even at higher cognitive levels, we are able to do prediction. We can predict how the laws of physics affect people, places, and things and even predict the end of someone's sentence.
In our work, we sought to create an artificial model that is able to mimic early, low level biological predictive behavior in a computer vision system. Our predictive vision model uses spatiotemporal sequence memories learned from deep sparse coding. This model is implemented using a biologically inspired architecture: one that utilizes sequence memories, lateral inhibition, and top-down feedback in a generative framework. Our model learns the causes of the data in a completely unsupervised manner, by simply observing and learning about the world. Spatiotemporal features are learned by minimizing a reconstruction error convolved over space and time, and can subsequently be used for recognition, classification, and future video prediction. Our experiments show that we are able to accurately predict what will happen in the future; furthermore, we can use our predictions to detect anomalous, unexpected events in both synthetic and real video sequences.
更多查看译文
AI 理解论文
溯源树
样例
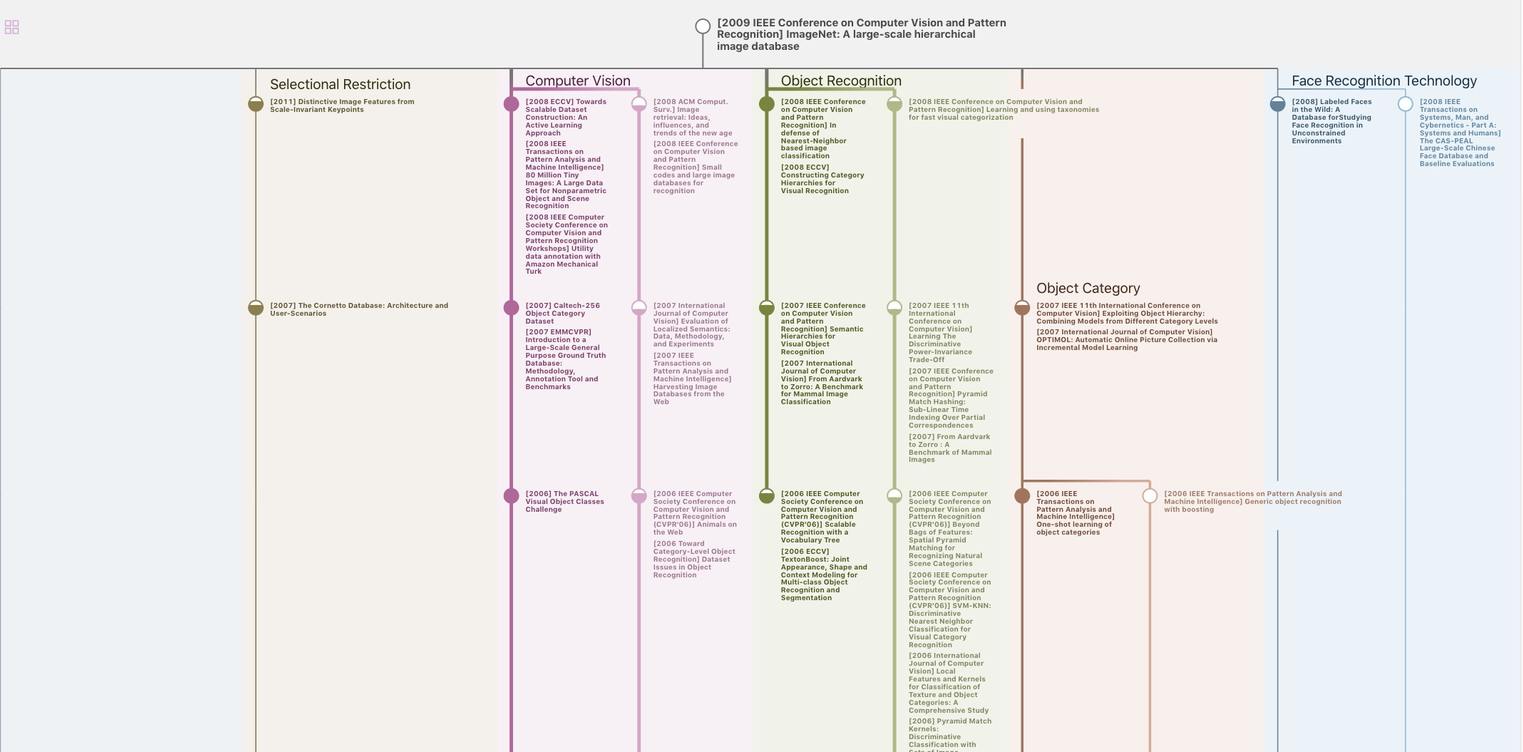
生成溯源树,研究论文发展脉络
Chat Paper
正在生成论文摘要