On The Feasibility Of Local Utility Redesign For Multiagent Optimization
2019 18TH EUROPEAN CONTROL CONFERENCE (ECC)(2019)
摘要
We study settings in which autonomous agents are designed with the goal of optimizing a given system-level objective. In typical approaches to this problem, each agent is endowed with a decision-making rule that specifies the agent's choice as a function of the information pertaining to the choices made by other agents in the system. This paper considers the scenario where the designed decision-making rules are not implementable in the realized system due to discrepancies between the anticipated and realized information available to the agents, i.e., the agents are in a situation where they do not have access to all information necessary to execute their designed decision-making rule. Given such inconsistencies, the focus of this paper is on whether the agents can preserve system-level efficiency guarantees in these unanticipated scenarios through local and independent redesigns of their decision-making rules. First, we show that distributed submodular maximization problems are particularly amenable to local redesigns of utility functions, i.e., agents can adapt to informational inconsistencies independently without incurring much loss in terms of system-level performance. However, for general non-submodular objective functions, our second result demonstrates that there are no local redesign methodologies that can offer any preservation of system-level efficiency guarantees. Accordingly, centralized coordination of a redesign may be imperative for maintaining efficiency guarantees in such systems.
更多查看译文
关键词
designed decision-making rule,realized system,anticipated realized information,system-level efficiency guarantees,local redesigns,independent redesigns,submodular maximization problems,informational inconsistencies,system-level performance,nonsubmodular objective functions,local redesign methodologies,local utility redesign,multiagent optimization,autonomous agents,system-level objective
AI 理解论文
溯源树
样例
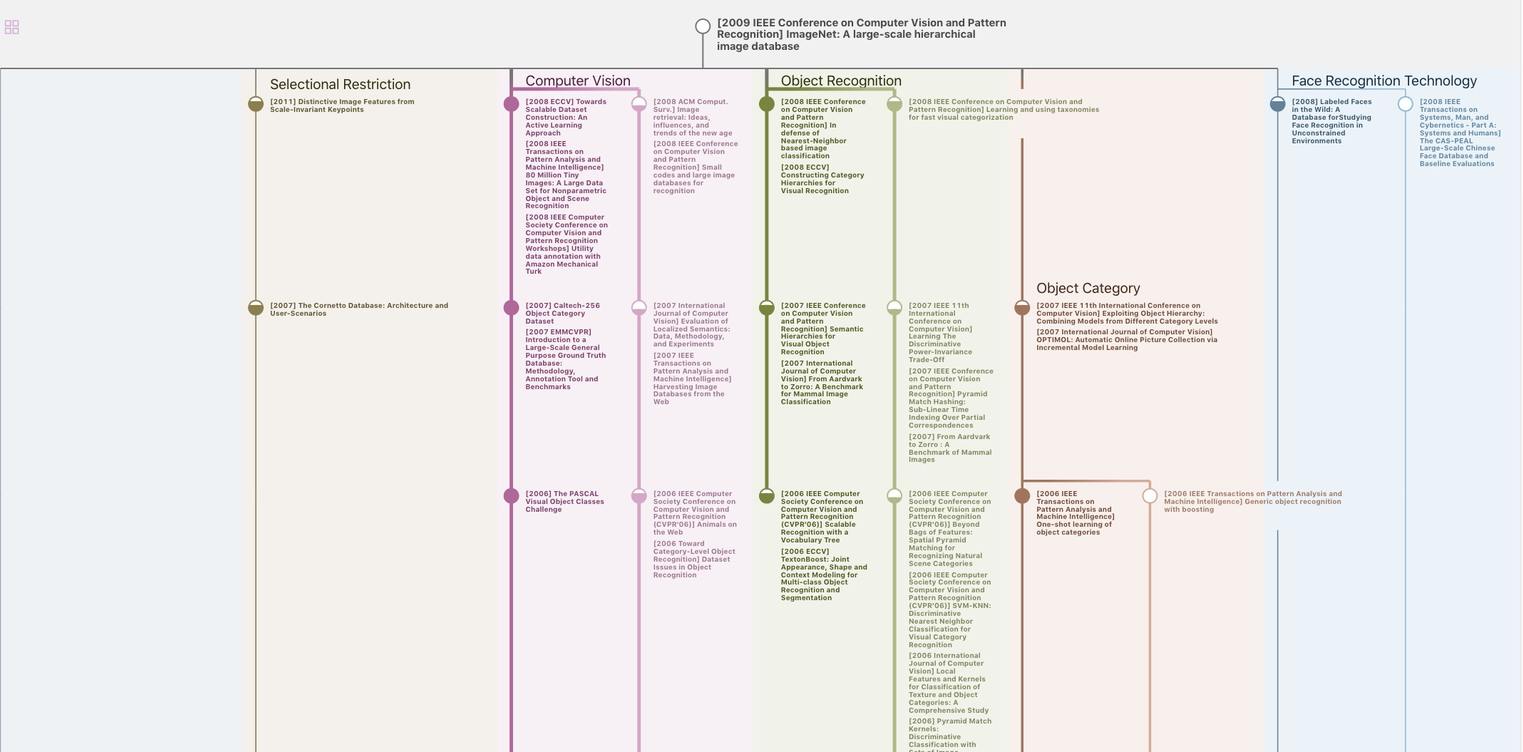
生成溯源树,研究论文发展脉络
Chat Paper
正在生成论文摘要