A predictive learning approach to optimal load sharing in energy management systems
2019 18th European Control Conference (ECC)(2019)
摘要
Given the total power demand, Pd, current practice of equal load sharing in the process industry is to distribute the load among power supply units and machines (e.g., diesel/gas/wind turbines) in proportion to the maximum power, i.e., P
i
= P
max
i
/Σ
i
P
d
, where P
max
i
denotes the maximum power of the i
th
unit. However, the efficiency of power supply units, vary in time and are highly individual, even in the case of units from same brand and model. Thus, by considering and utilizing these individual differences, it is possible to share the load in a more fuel/cost/energy optimal manner. To capture this potential, the work presented in this paper proposes an optimization and learning-based approach for sharing the load among a set of heterogeneous power supply units. The main contributions of this paper include formulation of the overall energy management system algorithm, including power grid connection and Energy Storage System (ESS), as a Mixed Integer Non-Linear Program (MINLP), as well as algorithms for robust moving horizon estimation of efficiency curves and machine learning-based algorithms for classification of turbine state and prediction of future power demand needed for the predictive planning scheme. The soundness and performance of the proposed algorithms are verified using actual data from a power plant including gas turbines and power grid connection in combination with ESS.
更多查看译文
关键词
predictive learning approach,heterogeneous power supply units,energy management system algorithm,power grid connection,machine learning-based algorithms,power plant,optimal load sharing,power demand,energy storage system,gas turbine,ESS,mixed integer non-linear program,MINLP,horizon estimation
AI 理解论文
溯源树
样例
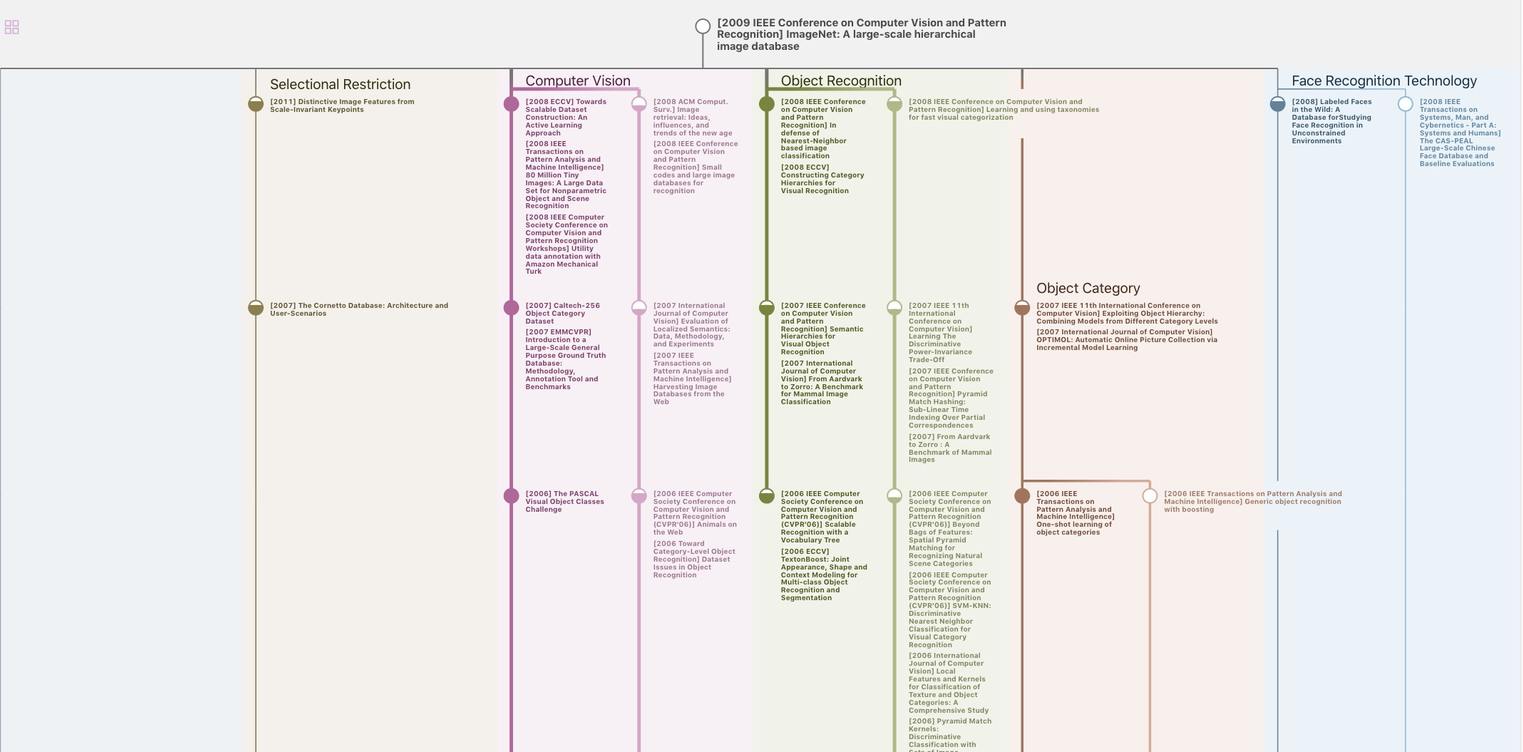
生成溯源树,研究论文发展脉络
Chat Paper
正在生成论文摘要