Learning and long-term retention of dynamic self-stabilization skills
Experimental Brain Research(2019)
摘要
In earlier studies, we had subjects use a joystick to balance themselves when seated in a device programmed to behave like an inverted pendulum. Subjects tested in a vertically oriented roll plane showed rapid learning for dynamically stabilizing themselves about the direction of balance when it corresponded with the direction of gravity. Subjects tested in a horizontally oriented roll plane, unlike the vertical roll plane subjects, did not have gravitational cues to determine their angular positions and showed minimal learning and persistent cyclical drifting. We describe here a training program to enhance learning and performance of dynamic stabilization in the horizontal roll plane based on our previous finding that balance control involves two dissociable components: alignment using gravity-dependent positional cues and alignment using dynamic cues. We hypothesized that teaching subjects to balance in a vertical roll plane to directions of balance that did not correspond with the direction of gravity would enhance the ability to stabilize at the direction of balance in the horizontal roll plane where gravity-dependent cues are absent. All subjects trained in vertical roll later showed greatly improved performance in horizontal plane balance. Control subjects exposed only to horizontal roll plane balancing showed minimal improvements. When retested 4 months later, the training subjects showed further performance improvements during the course of the retest trials whereas the control group showed no further improvement. Our findings indicate that balance control can be enhanced in situations lacking gravitationally dependent position cues as in weightlessness, when initial training occurs with such cues present.
更多查看译文
关键词
Dynamic balance, Vehicle control, Spatial disorientation, Motor skill learning, Long-term retention, Vestibular system, Somatosensation, Path integration, Spaceflight analog
AI 理解论文
溯源树
样例
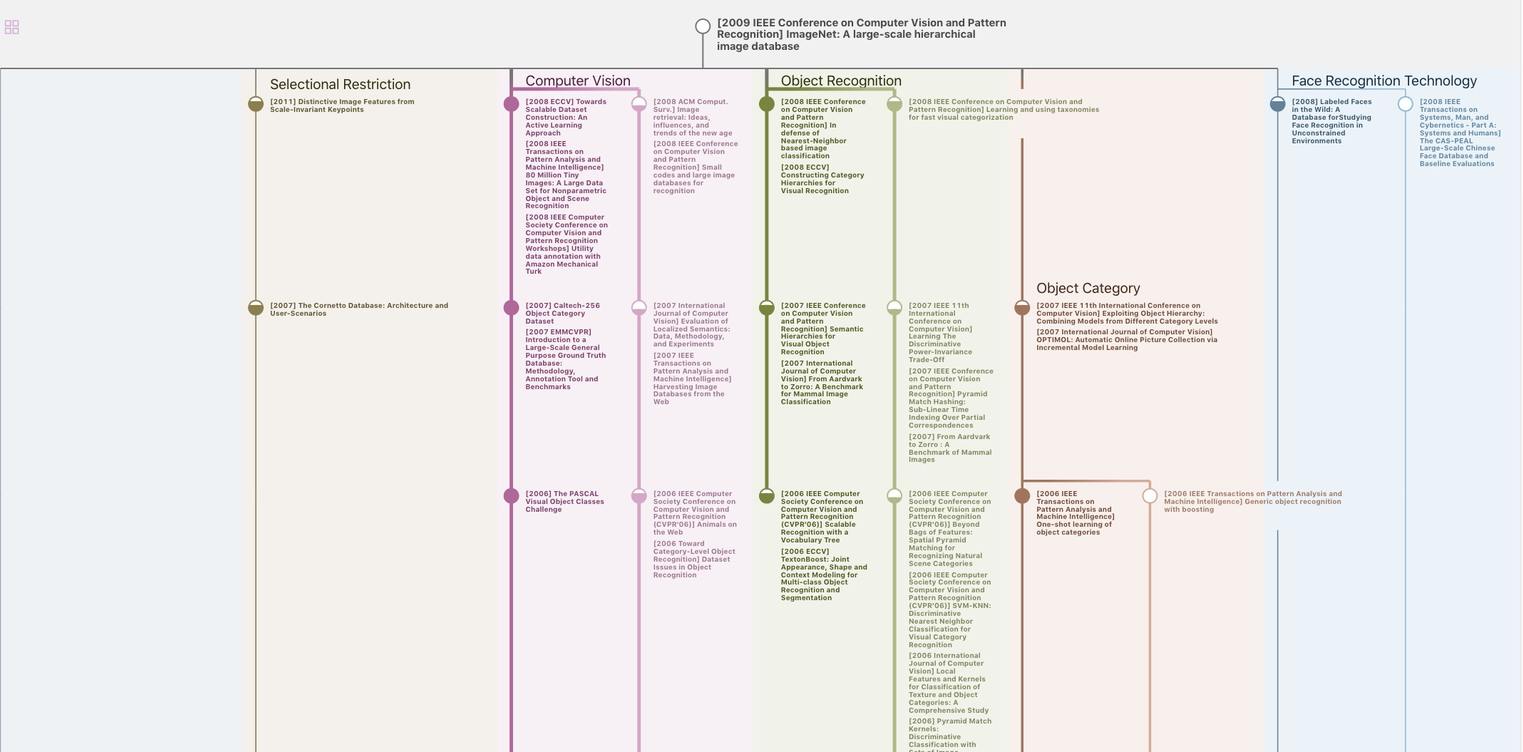
生成溯源树,研究论文发展脉络
Chat Paper
正在生成论文摘要