A Convolutional Neural Network With Mapping Layers for Hyperspectral Image Classification
IEEE TRANSACTIONS ON GEOSCIENCE AND REMOTE SENSING(2020)
摘要
In this article, we propose a convolutional neural network with mapping layers (MCNN) for hyperspectral image (HSI) classification. The proposed mapping layers map the input patch into a low-dimensional subspace by multilinear algebra. We use our mapping layers to reduce the spectral and spatial redundancies and maintain most energy of the input. The feature extracted by our mapping layers can also reduce the number of following convolutional layers for feature extraction. Our MCNN architecture avoids the declining accuracy with increasing layers phenomenon of deep learning models for HSI classification and also saves the training time for its effective mapping layers. Furthermore, we impose the 3-D convolutional kernel on the convolutional layer to extract the spectral-spatial features for HSI. We tested our MCNN on three data sets of Indian Pines, University of Pavia, and Salinas, and we achieved the classification accuracy of 98.3%, 99.5%, and 99.3%, respectively. Experimental results demonstrate that the proposed MCNN can significantly improve classification accuracy and save much time consumption.
更多查看译文
关键词
Convolutional neural network (CNN),dimension reduction,feature extraction,hyperspectral image (HSI) classification,mapping layers
AI 理解论文
溯源树
样例
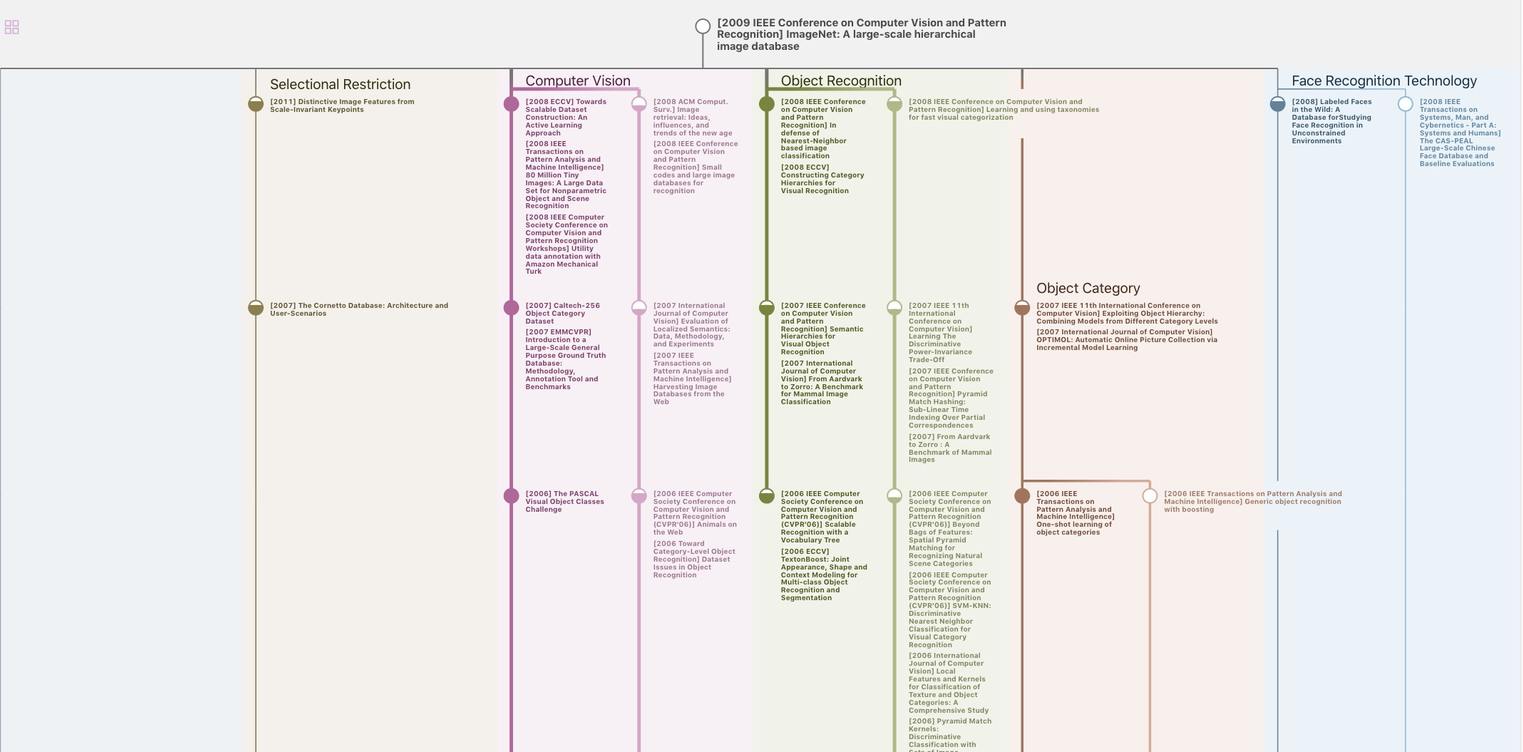
生成溯源树,研究论文发展脉络
Chat Paper
正在生成论文摘要