Exploiting Behavioral Differences to Detect Fake Ne
2018 9th IEEE Annual Ubiquitous Computing, Electronics & Mobile Communication Conference (UEMCON)(2018)
摘要
Online social platforms have become the most influential media and their impact will be far greater in the highly connected and super-efficient smart cities. The speed, reach and sheer volume of digital media pose a global challenge to combat fake news. There is an urgent need to build resilience in a post-truth era. Misinformation gnaws social cohesion and erodes the trust of the citizens. This study seeks to identify the key differences in the traits between fake news and normal information in tweets and present two case studies to showcase two such features, namely, user sentiments and spread pattern. We then we propose an AI-based system using Autoencoder and Recurrent Neural Networks to detect fake news in Sina Weibo. This Proof-of-Concept (POC) can achieve a reasonable accuracy and F1 score and also proves its applicability to other online social platforms. The proposed POC is especially useful for governments, companies and other organizations to identify such misinformation as early as possible so that immediate actions can be taken to minimize the potential negative effect. It can also be deployed for use by social media platform users.
更多查看译文
关键词
Fake news detection,Feature selection,Autoencoder,Recurrent neural network
AI 理解论文
溯源树
样例
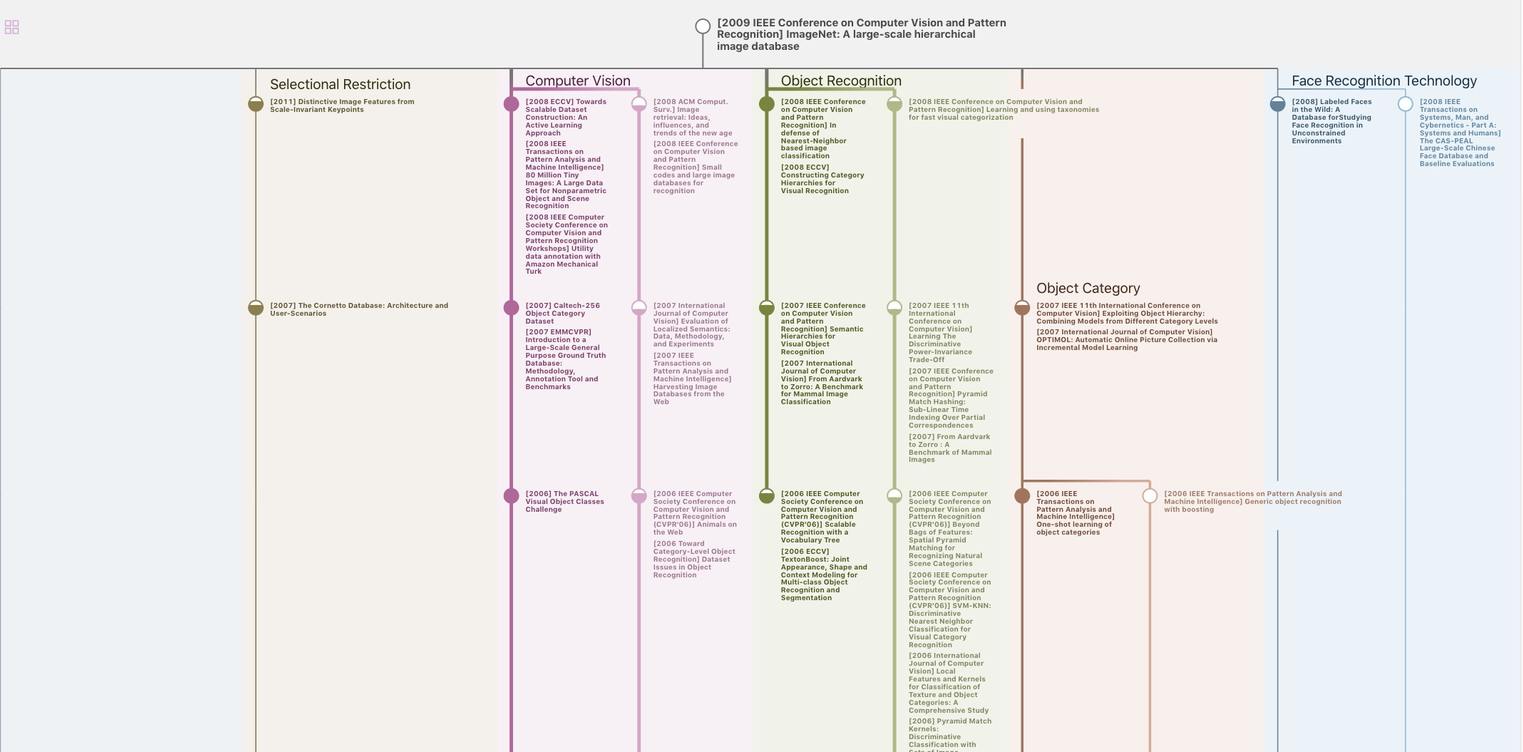
生成溯源树,研究论文发展脉络
Chat Paper
正在生成论文摘要