COMPUTATION OF SUM OF SQUARES POLYNOMIALS FROM DATA POINTS
SIAM JOURNAL ON NUMERICAL ANALYSIS(2020)
摘要
We propose an iterative algorithm for the numerical computation of sums of squares of polynomials approximating given data at prescribed interpolation points. The method is based on the definition of a convex functional $G$ arising from the dualization of a quadratic regression over the Cholesky factors of the sum of squares decomposition. In order to justify the construction, the domain of $G$, the boundary of the domain, and the behavior at infinity are analyzed in detail. When the data interpolate a positive univariate polynomial, we show that in the context of the Lukács sum of squares representation, $G$ is coercive and strictly convex, which yields a unique critical point and a corresponding decomposition in a sum of squares. For multivariate polynomials which admit a decomposition in a sum of squares and up to a small perturbation of size $\varepsilon$, $G^\varepsilon$ is always coercive, and so its minimum yields an approximate decomposition in a sum of squares. Various unconstrained descent algorithms are proposed to minimize $G$. Numerical examples are provided for univariate and bivariate polynomials.
更多查看译文
关键词
squares polynomials,data points,computation,sum
AI 理解论文
溯源树
样例
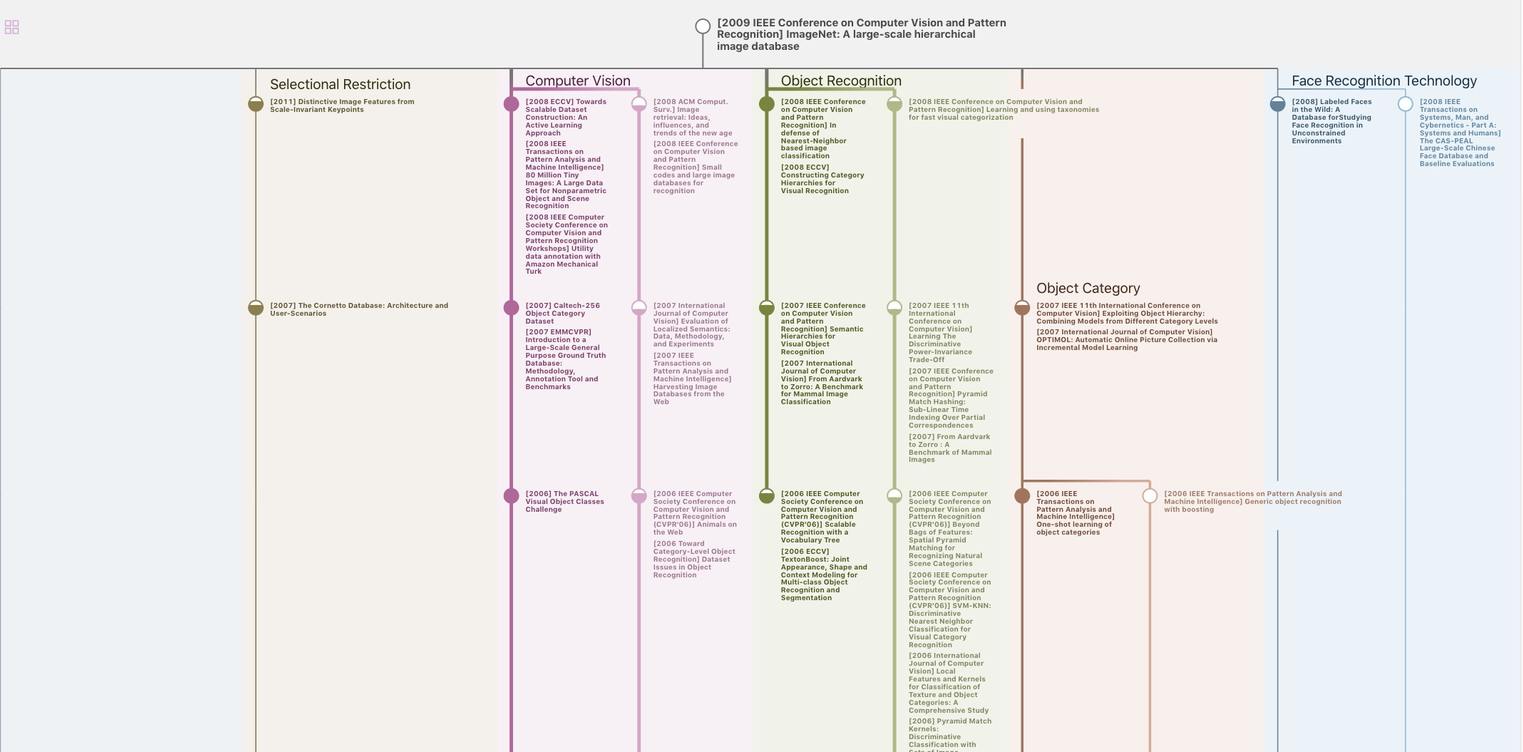
生成溯源树,研究论文发展脉络
Chat Paper
正在生成论文摘要