Pattern recognition and prognostic analysis of longitudinal blood pressure records in hemodialysis treatment based on a convolutional neural network.
Journal of biomedical informatics(2019)
摘要
OBJECTIVE:The aim of this study is to analyze and visualize blood pressure (BP) patterns during continuous hemodialysis (HD) sessions, referred to as multiple-session patterns (MSPs), and explore whether deep learning models with MSPs have better performance.
MATERIAL AND METHODS:Data from 3.79 million hemodialysis BP records collected from July 30, 2007, to August 25, 2016, were obtained from the health system's electronic health records. We analyzed BP patterns during 36 continuous HD sessions (approximately 3 months) and selected 1311 (survival: 1246; death: 65) end-stage renal disease patients to classify 1-year outcomes (survival or death). Convolution kernels of different sizes were used to construct convolutional neural networks to recognize MSPs and BP patterns during a single HD session, referred to as single-session patterns (SSPs). BP patterns corresponded to convolution kernels and were represented and visualized as the input patches that activate the feature maps most. We used global average pooling (GAP) to measure the overall response of the inputs to each convolution kernel (pattern). The weights of the fully connected layers after GAP can measure the correlations between the convolution kernels (patterns) and the classification results. We solved the problem of data imbalance with a two-phase training strategy.
RESULTS:The F1_score was 0.782 ± 0.058 (95% CI) in the models with SSPs and was approximately 19.5% higher (0.977 ± 0.014, 95% CI) in the models with MSPs.
CONCLUSIONS:The results indicated that consistent with previous studies, patients with lower BPs and longer HD sessions have better prognoses. BP patterns during continuous HD sessions can represent patients' 1-year mortality risk better than BP patterns during a single HD session and therefore improve the performance of prediction models.
更多查看译文
AI 理解论文
溯源树
样例
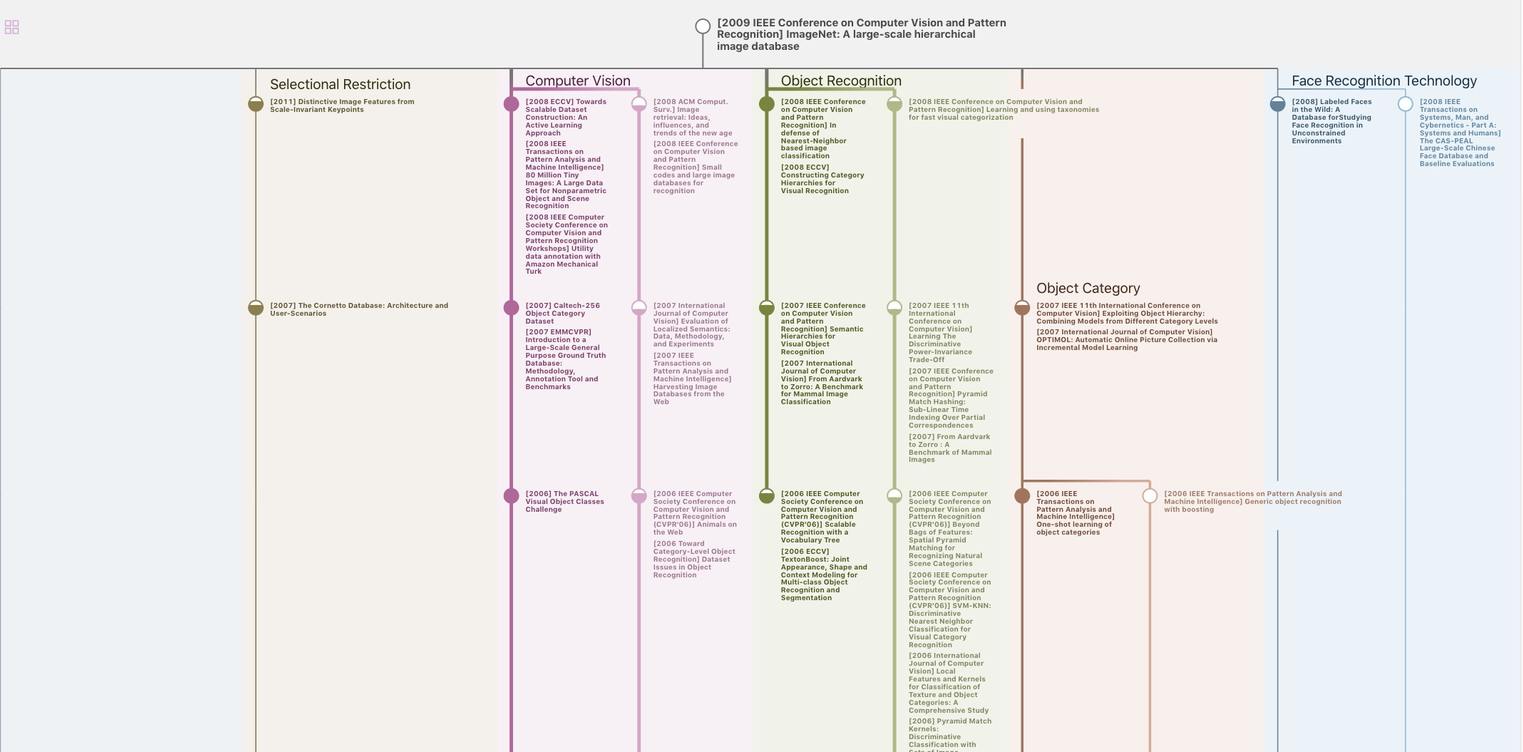
生成溯源树,研究论文发展脉络
Chat Paper
正在生成论文摘要