Solving Discounted Stochastic Two-Player Games with Near-Optimal Time and Sample Complexity
INTERNATIONAL CONFERENCE ON ARTIFICIAL INTELLIGENCE AND STATISTICS, VOL 108(2019)
摘要
In this paper, we settle the sampling complexity of solving discounted two-player turn-based zero-sum stochastic games up to polylogarithmic factors. Given a stochastic game with discount factor $\gamma\in(0,1)$ we provide an algorithm that computes an $\epsilon$-optimal strategy with high-probability given $\tilde{O}((1 - \gamma)^{-3} \epsilon^{-2})$ samples from the transition function for each state-action-pair. Our algorithm runs in time nearly linear in the number of samples and uses space nearly linear in the number of state-action pairs. As stochastic games generalize Markov decision processes (MDPs) our runtime and sample complexities are optimal due to Azar et al (2013). We achieve our results by showing how to generalize a near-optimal Q-learning based algorithms for MDP, in particular Sidford et al (2018), to two-player strategy computation algorithms. This overcomes limitations of standard Q-learning and strategy iteration or alternating minimization based approaches and we hope will pave the way for future reinforcement learning results by facilitating the extension of MDP results to multi-agent settings with little loss.
更多查看译文
关键词
stochastic,complexity,two-player,near-optimal
AI 理解论文
溯源树
样例
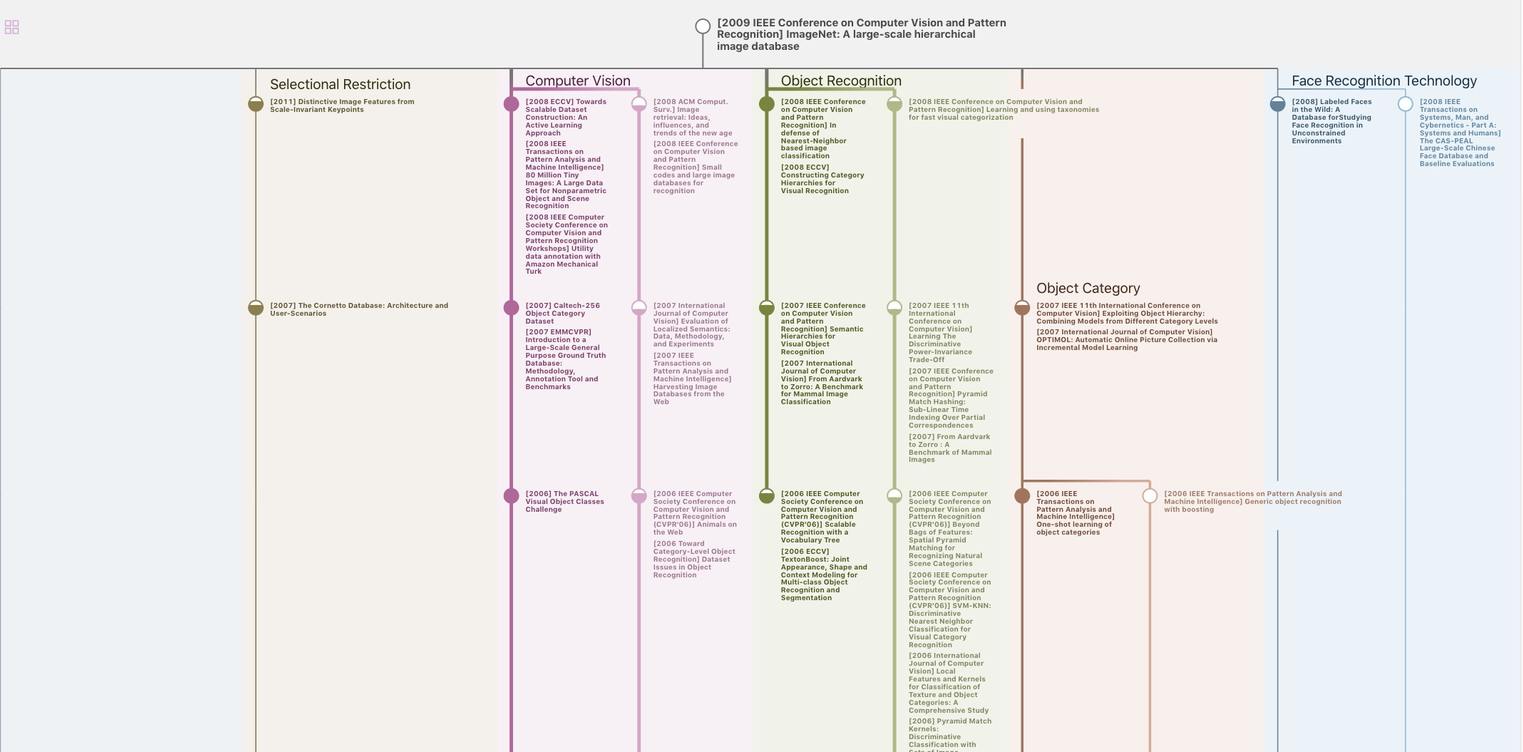
生成溯源树,研究论文发展脉络
Chat Paper
正在生成论文摘要