LWPCMF: Logistic Weighted Profile-Based Collaborative Matrix Factorization for Predicting MiRNA-Disease Associations
IEEE/ACM Transactions on Computational Biology and Bioinformatics(2021)
摘要
As is known to all, constructing experiments to predict unknown miRNA-disease association is time-consuming, laborious and costly. Accordingly, new prediction model should be conducted to predict novel miRNA-disease associations. What's more, the performance of this method should be high and reliable. In this paper, a new computation model Logistic Weighted Profile-based Collaborative Matrix Factorization (LWPCMF) is put forward. In this method, weighted profile (WP) is combined with collaborative matrix factorization (CMF) to increase the performance of this model. And, the neighbor information is considered. In addition, logistic function is applied to miRNA functional similarity matrix and disease semantic similarity matrix to extract valuable information. At the same time, by adding WP and logistic function, the known correlation can be protected. And, Gaussian Interaction Profile (GIP) kernels of miRNAs and diseases are added to miRNA functional similarity network and disease semantic similarity network to augment kernel similarities. Then, a five-fold cross validation is implemented to evaluate the predictive ability of this method. Besides, case studies are conducted to view the experimental results. The final result contains not only known associations but also newly predicted ones. And, the result proves that our method is better than other existing methods. This model is able to predict potential miRNA-disease associations.
更多查看译文
关键词
Diseases,Kernel,Logistics,Semantics,Computational modeling,Databases,Collaboration,miRNA-disease association,collaborative matrix factorization,logistic function,weighted profile
AI 理解论文
溯源树
样例
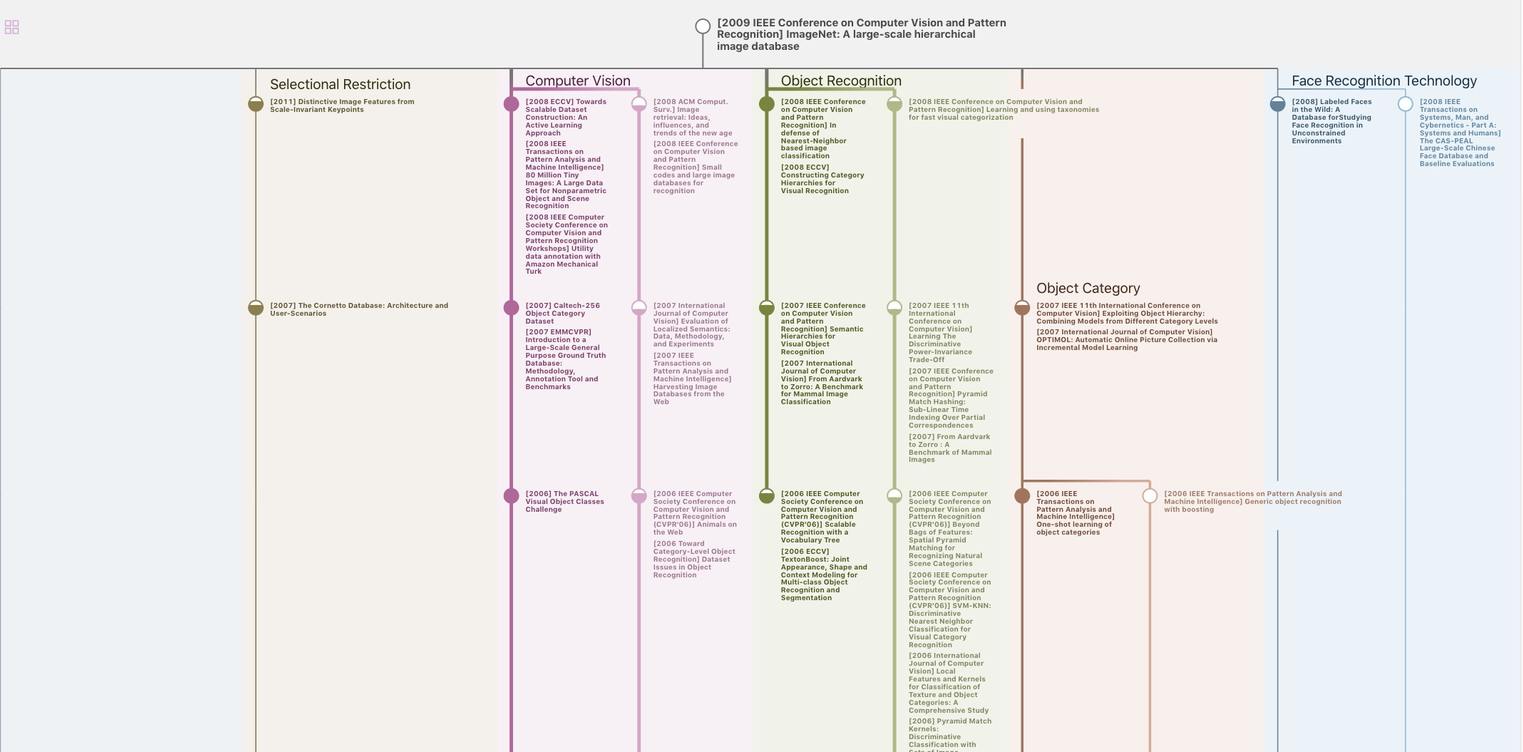
生成溯源树,研究论文发展脉络
Chat Paper
正在生成论文摘要