DiCeS: Detecting Communities in Network Streams over the Cloud
2019 IEEE 12th International Conference on Cloud Computing (CLOUD)(2019)
摘要
We consider the problem of uncovering communities in complex real-world networks whose nodes and their respective associations originate in streams of data. Although community detection has received much attention in centralized settings, the prevalence of online social networks has resulted in unprecedented volumes of data whose handling calls for novel streaming approaches. Moreover, bursty production of network interactions necessitates cloud-enabled techniques that can both deal with diverse data rates and deploy more computing resources on the fly for improved performance yields. We propose a distributed streaming community detection approach termed DiCeS, and implement it as a cloud application. While seeking communities, the novelty of our approach is at balancing the incoming load to a cluster of computing nodes and adjusting the cluster processing capacity in an elastic manner. We also provide fault tolerance by ensuring that temporarily suspended or failed nodes are restored and all edges of the network stream ultimately received their due processing. Lastly, DiCeS is interactive regarding i) updating the target communities, and ii) obtaining results on demand. Our experimental results demonstrate that DiCeS does handle the edges of real-world network streams at impressive rates, allows for near-linear scaling, and outperforms previous non-distributed approaches. While using ground-truth communities for a wide range of large real-word networks, we also show that DiCeS attains improved accuracy if compared to earlier centralized algorithms.
更多查看译文
关键词
graph streams,community detection,cloud
AI 理解论文
溯源树
样例
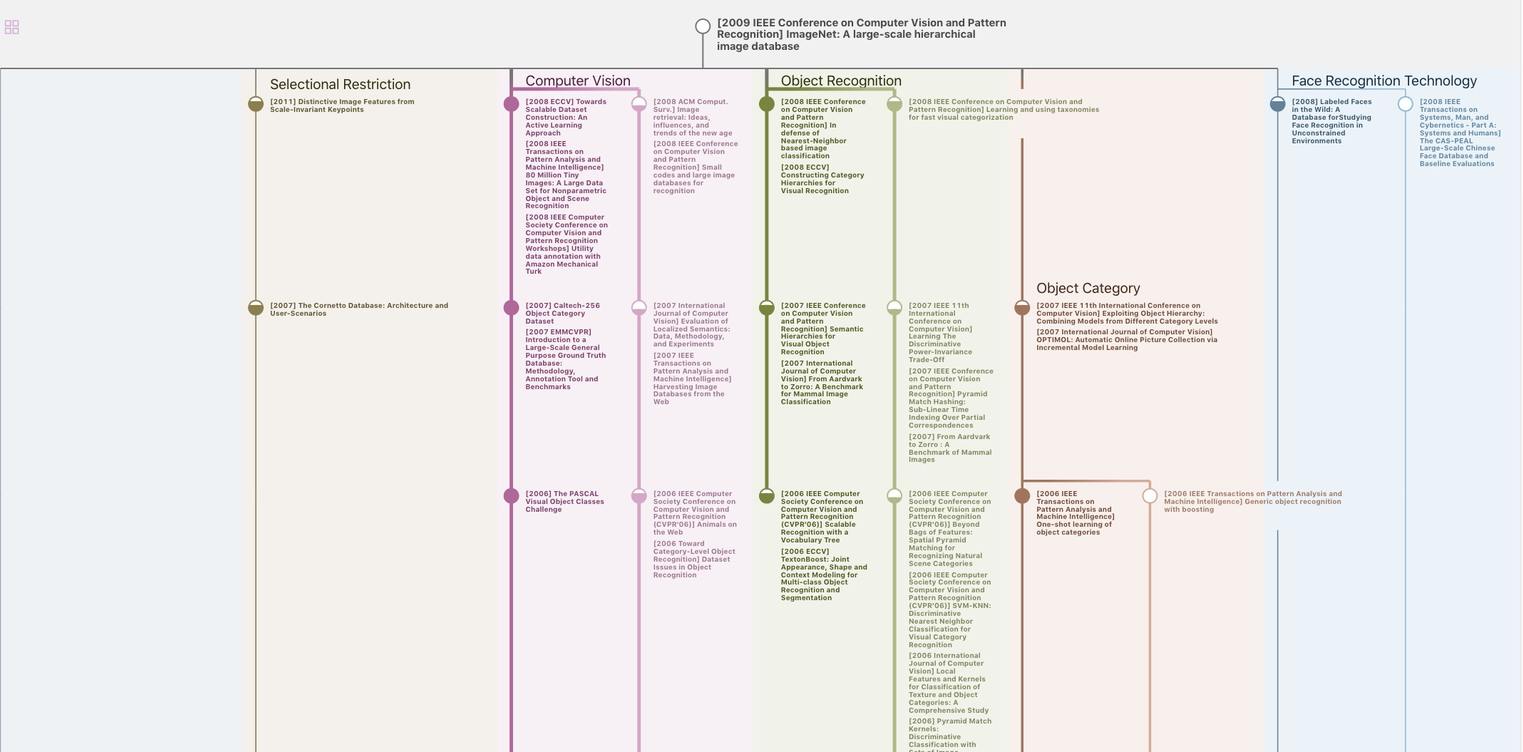
生成溯源树,研究论文发展脉络
Chat Paper
正在生成论文摘要