Degradation Estimation of Turbines in Wind Farm Using Denoising Autoencoder Model
2019 IEEE International Conference on Prognostics and Health Management (ICPHM)(2019)
摘要
We propose a method to estimate the power performance degradation in wind turbines (WTs) that arises from damage in the turbine blade and other components. In general, the single anemometer mounted on the nacelle is unable to measure precise wind speed distributions that the WT receives, thus, degradation of the power output is difficult to evaluate. By focusing on the fact that the power output data of adjacent WTs have some correlation although the wake effect on downstream turbines sometimes exists, our method uses the power output data of every WT in a farm to estimate the amount of degraded power performance of each turbine by the introduction of the virtual variable which corresponds to each turbine's degraded amount. The feature of the correlation among each WT's non-degradation data was learned by a denoising autoencoder (DAE). The virtual variables along with the power output are fed into the trained DAE model and these variables were updated by minimizing the reconstruction error. Moreover, the proposed method can perform the estimation even when some WTs are down, i.e., due to the periodical maintenance, and can classify between non-degraded and degraded WTs without enforcing diagnostics to set suitable threshold parameters. We demonstrated the superiority of this novel method over traditional methods by using real and artificial data inputs.
更多查看译文
关键词
wind farm,denoising autoencoder model,wind turbines,turbine blade damage,anemometer,nacelle,wake effect,maintenance method
AI 理解论文
溯源树
样例
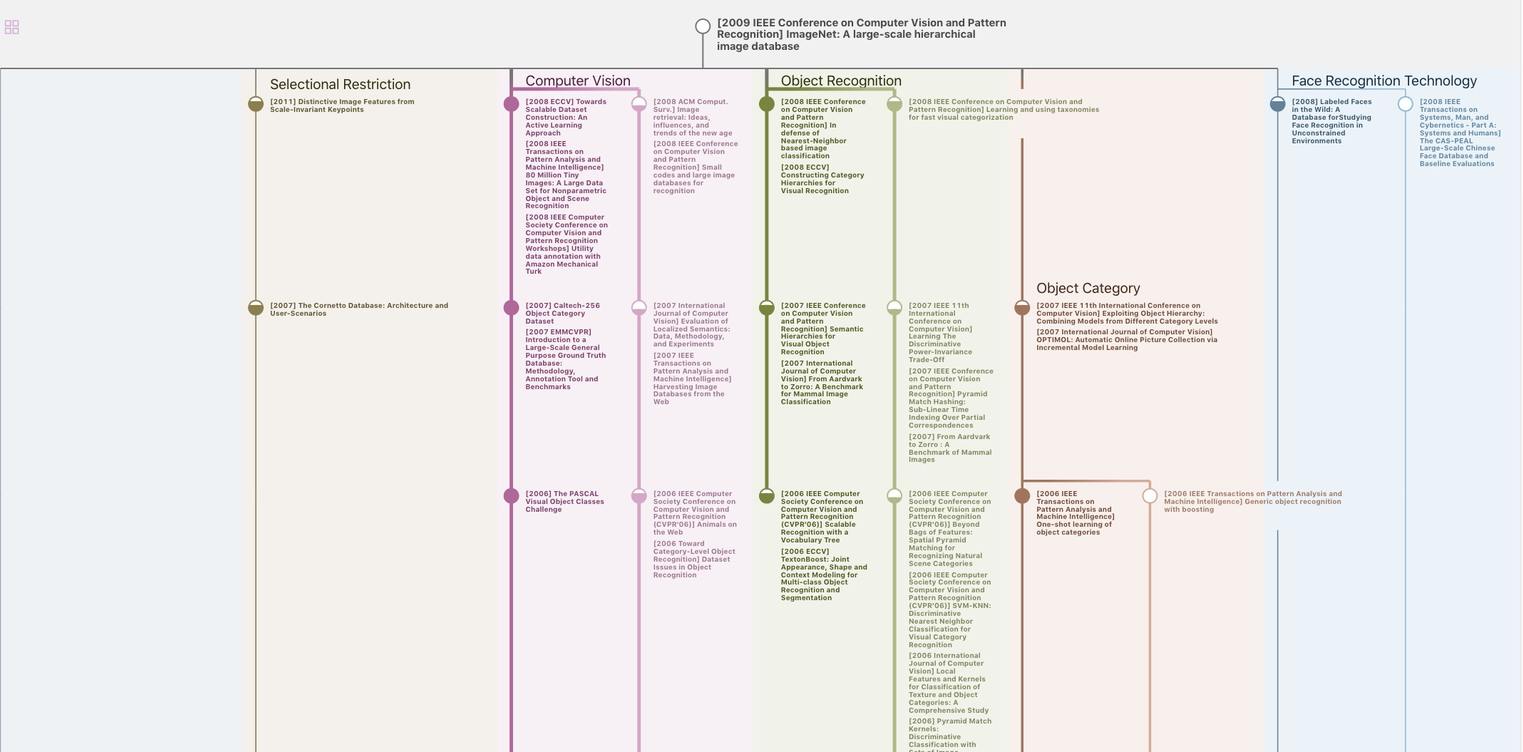
生成溯源树,研究论文发展脉络
Chat Paper
正在生成论文摘要